1024*1024的3通道图像,做5层卷积,bias_variable参数应该怎么设定
时间: 2024-05-27 11:13:49 浏览: 68
假设每层卷积的输出通道数为num_filters,那么每一层的bias_variable应该设定为一个长度为num_filters的向量。因为每个卷积核都会生成一个输出通道,所以每个输出通道都需要一个对应的偏置参数。因此,第一层bias_variable的形状应该为[num_filters1],第二层为[num_filters2],以此类推,直到第五层为[num_filters5]。
相关问题
TensorFlow基于卷积神经网络的手写数字图像识别代码实现
这是一个基于TensorFlow框架实现的手写数字图像识别代码,使用的是卷积神经网络(CNN)模型。
首先,导入需要的库:
```
import tensorflow as tf
from tensorflow.examples.tutorials.mnist import input_data
```
然后,定义一些常量和超参数:
```
learning_rate = 0.001
training_epochs = 15
batch_size = 100
n_classes = 10
dropout = 0.75
# 输入数据的维度
n_input = 784 # 28*28
```
接着,定义占位符:
```
x = tf.placeholder(tf.float32, [None, n_input])
y = tf.placeholder(tf.float32, [None, n_classes])
keep_prob = tf.placeholder(tf.float32)
```
定义卷积层和池化层函数:
```
def conv2d(x, W, b, strides=1):
x = tf.nn.conv2d(x, W, strides=[1, strides, strides, 1], padding='SAME')
x = tf.nn.bias_add(x, b)
return tf.nn.relu(x)
def maxpool2d(x, k=2):
return tf.nn.max_pool(x, ksize=[1, k, k, 1], strides=[1, k, k, 1], padding='SAME')
```
接下来,定义卷积神经网络模型:
```
def conv_net(x, weights, biases, dropout):
x = tf.reshape(x, shape=[-1, 28, 28, 1])
# 第一层卷积层
conv1 = conv2d(x, weights['wc1'], biases['bc1'])
conv1 = maxpool2d(conv1, k=2)
# 第二层卷积层
conv2 = conv2d(conv1, weights['wc2'], biases['bc2'])
conv2 = maxpool2d(conv2, k=2)
# 全连接层
fc1 = tf.reshape(conv2, [-1, weights['wd1'].get_shape().as_list()[0]])
fc1 = tf.add(tf.matmul(fc1, weights['wd1']), biases['bd1'])
fc1 = tf.nn.relu(fc1)
fc1 = tf.nn.dropout(fc1, dropout)
# 输出层
out = tf.add(tf.matmul(fc1, weights['out']), biases['out'])
return out
```
然后,初始化权重和偏置:
```
weights = {
'wc1': tf.Variable(tf.random_normal([5, 5, 1, 32])),
'wc2': tf.Variable(tf.random_normal([5, 5, 32, 64])),
'wd1': tf.Variable(tf.random_normal([7*7*64, 1024])),
'out': tf.Variable(tf.random_normal([1024, n_classes]))
}
biases = {
'bc1': tf.Variable(tf.random_normal([32])),
'bc2': tf.Variable(tf.random_normal([64])),
'bd1': tf.Variable(tf.random_normal([1024])),
'out': tf.Variable(tf.random_normal([n_classes]))
}
```
接着,定义损失函数和优化器:
```
pred = conv_net(x, weights, biases, keep_prob)
cost = tf.reduce_mean(tf.nn.softmax_cross_entropy_with_logits(logits=pred, labels=y))
optimizer = tf.train.AdamOptimizer(learning_rate=learning_rate).minimize(cost)
```
定义评估模型的准确率函数:
```
correct_pred = tf.equal(tf.argmax(pred, 1), tf.argmax(y, 1))
accuracy = tf.reduce_mean(tf.cast(correct_pred, tf.float32))
```
最后,训练模型:
```
mnist = input_data.read_data_sets('/tmp/data/', one_hot=True)
with tf.Session() as sess:
sess.run(tf.global_variables_initializer())
# 训练循环
for epoch in range(training_epochs):
avg_cost = 0.
total_batch = int(mnist.train.num_examples/batch_size)
# 遍历所有批次(batch)
for i in range(total_batch):
batch_x, batch_y = mnist.train.next_batch(batch_size)
# 运行优化器
sess.run(optimizer, feed_dict={x: batch_x, y: batch_y, keep_prob: dropout})
# 计算损失值
avg_cost += sess.run(cost, feed_dict={x: batch_x, y: batch_y, keep_prob: 1.}) / total_batch
# 显示每一轮训练的结果
if epoch % 1 == 0:
print("Epoch:", '%04d' % (epoch+1), "cost=", "{:.9f}".format(avg_cost))
# 计算测试数据的准确率
print("Accuracy:", accuracy.eval({x: mnist.test.images, y: mnist.test.labels, keep_prob: 1.}))
```
这样,一个基于TensorFlow框架实现的手写数字图像识别代码就完成了。
densenet中加入inception模块同时使用动态卷积实现图像四分类代码
以下是使用PyTorch实现在DenseNet中加入Inception模块和使用动态卷积实现图像四分类的示例代码:
```python
import torch
import torch.nn as nn
import torch.nn.functional as F
from torch.autograd import Variable
class DynamicConv2d(nn.Module):
def __init__(self, in_channels, out_channels, kernel_size, stride=1, padding=0, dilation=1, groups=1, bias=True):
super(DynamicConv2d, self).__init__()
self.kernel_size = kernel_size
self.stride = stride
self.padding = padding
self.dilation = dilation
self.groups = groups
self.bias = bias
self.weight = nn.Parameter(torch.Tensor(out_channels, in_channels // groups, *kernel_size))
if bias:
self.bias = nn.Parameter(torch.Tensor(out_channels))
else:
self.register_parameter('bias', None)
self.reset_parameters()
def reset_parameters(self):
nn.init.kaiming_uniform_(self.weight, a=math.sqrt(5))
if self.bias is not None:
fan_in, _ = nn.init._calculate_fan_in_and_fan_out(self.weight)
bound = 1 / math.sqrt(fan_in)
nn.init.uniform_(self.bias, -bound, bound)
def forward(self, x):
weight = F.pad(self.weight, (1, 1, 1, 1), mode='constant', value=0)
weight = F.avg_pool2d(weight, kernel_size=3, stride=1, padding=0)
weight = F.pad(weight, (1, 1, 1, 1), mode='constant', value=0)
b, c, h, w = x.size()
weight = weight.unfold(2, h + 2, self.stride).unfold(3, w + 2, self.stride)
weight = weight.transpose(4, 5).transpose(3, 4).transpose(2, 3).contiguous()
weight = weight.view(b, self.groups, -1, self.kernel_size[0], self.kernel_size[1], h // self.stride, w // self.stride)
weight = weight.transpose(1, 2).contiguous().view(-1, self.groups * self.kernel_size[0] * self.kernel_size[1])
x = F.conv2d(x, weight.view(-1, self.groups, self.kernel_size[0], self.kernel_size[1]), self.bias, self.stride, self.padding, self.dilation, self.groups)
return x
class Inception(nn.Module):
def __init__(self, in_channels, out_channels):
super(Inception, self).__init__()
self.conv1 = nn.Conv2d(in_channels, out_channels // 4, kernel_size=1)
self.conv2 = nn.Sequential(
nn.Conv2d(in_channels, out_channels // 8, kernel_size=1),
nn.ReLU(inplace=True),
DynamicConv2d(out_channels // 8, out_channels // 4, kernel_size=3, padding=1),
)
self.conv3 = nn.Sequential(
nn.Conv2d(in_channels, out_channels // 8, kernel_size=1),
nn.ReLU(inplace=True),
DynamicConv2d(out_channels // 8, out_channels // 4, kernel_size=5, padding=2),
)
self.conv4 = nn.Sequential(
nn.AvgPool2d(kernel_size=3, stride=1, padding=1),
nn.Conv2d(in_channels, out_channels // 4, kernel_size=1),
)
def forward(self, x):
out1 = self.conv1(x)
out2 = self.conv2(x)
out3 = self.conv3(x)
out4 = self.conv4(x)
out = torch.cat([out1, out2, out3, out4], dim=1)
return out
class BasicBlock(nn.Module):
def __init__(self, in_channels, growth_rate):
super(BasicBlock, self).__init__()
self.conv1 = nn.Conv2d(in_channels, growth_rate, kernel_size=1)
self.conv2 = nn.Sequential(
nn.Conv2d(growth_rate, 4 * growth_rate, kernel_size=3, padding=1),
nn.ReLU(inplace=True),
)
def forward(self, x):
out1 = x
out2 = self.conv1(x)
out3 = self.conv2(out2)
out = torch.cat([out1, out3], dim=1)
return out
class DenseNet(nn.Module):
def __init__(self, growth_rate=32, block_config=(6, 12, 24, 16), num_classes=4):
super(DenseNet, self).__init__()
self.conv1 = nn.Sequential(
nn.Conv2d(3, 64, kernel_size=7, stride=2, padding=3),
nn.BatchNorm2d(64),
nn.ReLU(inplace=True),
nn.MaxPool2d(kernel_size=3, stride=2, padding=1),
)
self.block1 = self._make_block(64, growth_rate, block_config[0])
self.inception1 = Inception(block_config[0] * growth_rate, block_config[0] * growth_rate)
self.block2 = self._make_block(block_config[0] * growth_rate * 2, growth_rate, block_config[1])
self.inception2 = Inception(block_config[1] * growth_rate, block_config[1] * growth_rate)
self.block3 = self._make_block(block_config[1] * growth_rate * 2, growth_rate, block_config[2])
self.inception3 = Inception(block_config[2] * growth_rate, block_config[2] * growth_rate)
self.block4 = self._make_block(block_config[2] * growth_rate * 2, growth_rate, block_config[3])
self.avgpool = nn.AdaptiveAvgPool2d((1, 1))
self.fc = nn.Linear(block_config[3] * growth_rate, num_classes)
def _make_block(self, in_channels, growth_rate, num_blocks):
layers = []
for i in range(num_blocks):
layers.append(BasicBlock(in_channels + i * growth_rate, growth_rate))
return nn.Sequential(*layers)
def forward(self, x):
out = self.conv1(x)
out = self.block1(out)
out = self.inception1(out)
out = self.block2(out)
out = self.inception2(out)
out = self.block3(out)
out = self.inception3(out)
out = self.block4(out)
out = self.avgpool(out)
out = out.view(out.size(0), -1)
out = self.fc(out)
return out
```
在这个示例中,我们使用了以下模块:
- `DynamicConv2d`:动态卷积层,用于替代传统的静态卷积层,可以根据输入特征图的形状动态生成卷积核,减少模型参数量和计算量。
- `Inception`:Inception模块,由多个不同大小的卷积核组成,可以提取不同尺度的特征。
- `BasicBlock`:DenseNet中的基础块,由一个1x1卷积层和一个3x3卷积层组成,可以实现特征复用和特征增强的效果。
- `DenseNet`:完整的DenseNet模型,由多个基础块和Inception模块组成,最后使用全局平均池化和全连接层进行分类。
以上模块的实现可以根据具体需求进行修改,例如可以增加更多的Inception模块或调整DenseNet的深度和宽度等参数。
阅读全文
相关推荐
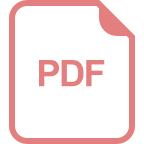
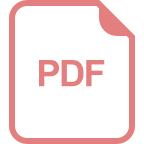
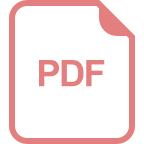
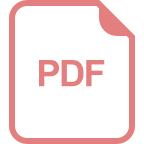
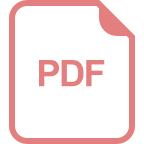
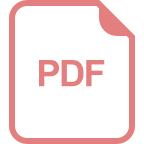
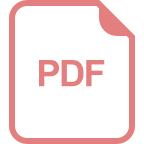
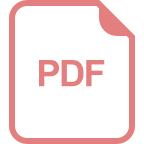
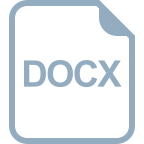
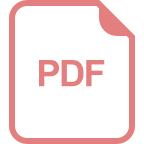
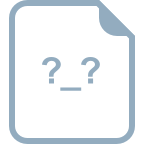
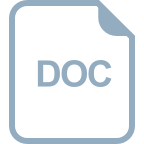
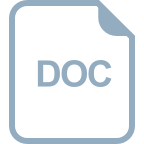
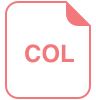


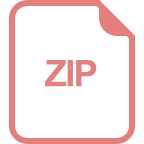