文本用KMeans聚类并可视化
时间: 2024-04-30 13:20:07 浏览: 8
首先,需要准备好需要聚类的文本数据。然后,可以使用Python中的sklearn库中的KMeans算法进行聚类。
以下是一个简单的示例代码:
```python
from sklearn.feature_extraction.text import TfidfVectorizer
from sklearn.cluster import KMeans
import matplotlib.pyplot as plt
# 假设有一个包含文本数据的列表
text_data = ['This is the first document.',
'This is the second document.',
'And this is the third one.',
'Is this the first document?']
# 将文本数据转换为TF-IDF特征
vectorizer = TfidfVectorizer(stop_words='english')
X = vectorizer.fit_transform(text_data)
# 使用KMeans算法进行聚类
kmeans = KMeans(n_clusters=2, random_state=0).fit(X)
# 可视化聚类结果
plt.scatter(X[:, 0], X[:, 1], c=kmeans.labels_.astype(float))
plt.show()
```
在上述示例中,我们使用TF-IDF向量化器将文本数据转换为向量,并使用KMeans算法将这些向量聚类为2个簇。最后,我们使用matplotlib库将聚类结果可视化。
需要注意的是,文本数据的处理和聚类结果的可视化都需要根据具体的需求进行适当的调整。
相关推荐
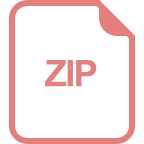
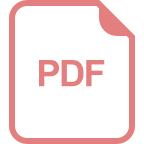
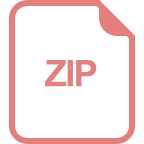














