class SpiralIterator: def init(self, source, x=810, y=500, length=None): self.source = source self.row = np.shape(self.source)[0]#第一个元素是行数 self.col = np.shape(self.source)[1]#第二个元素是列数 if length: self.length = min(length, np.size(self.source)) else: self.length = np.size(self.source) if x: self.x = x else: self.x = self.row // 2 if y: self.y = y else: self.y = self.col // 2 self.i = self.x self.j = self.y self.iteSize = 0 geo_transform = dsm_data.GetGeoTransform() self.x_origin = geo_transform[0] self.y_origin = geo_transform[3] self.pixel_width = geo_transform[1] self.pixel_height = geo_transform[5] def hasNext(self): return self.iteSize < self.length # 不能取更多值了 def get(self): if self.hasNext(): # 还能再取一个值 # 先记录当前坐标的值 —— 准备返回 i = self.i j = self.j val = self.source[i][j] # 计算下一个值的坐标 relI = self.i - self.x # 相对坐标 relJ = self.j - self.y # 相对坐标 if relJ > 0 and abs(relI) < relJ: self.i -= 1 # 上 elif relI < 0 and relJ > relI: self.j -= 1 # 左 elif relJ < 0 and abs(relJ) > relI: self.i += 1 # 下 elif relI >= 0 and relI >= relJ: self.j += 1 # 右 #判断索引是否在矩阵内 x = self.x_origin + (j + 0.5) * self.pixel_width y = self.y_origin + (i + 0.5) * self.pixel_height z = val self.iteSize += 1 return x, y, z dsm_path = 'C:\sanwei\jianmo\Productions\Production_2\Production_2_DSM_part_2_2.tif' dsm_data = gdal.Open(dsm_path) dsm_array = dsm_data.ReadAsArray() spiral_iterator = SpiralIterator(dsm_array,x=810,y=500) while spiral_iterator.hasNext(): x, y, z = spiral_iterator.get() print(f'Value at ({x},{y}):{z}')这段代码怎么改可以在已知相机内外参矩阵前提下,利用共线方程将地面点坐标反算其在原始航片对应的像素行列号
时间: 2024-04-27 21:24:48 浏览: 107
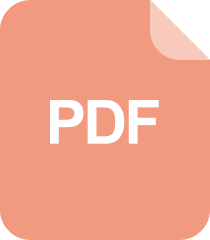
对Python中class和instance以及self的用法详解
这段代码与相机内外参矩阵无关,它是用来迭代遍历二维数组,并返回每个元素的坐标和值的。如果要将地面点坐标反算其在原始航片对应的像素行列号,则需要进行如下步骤:
1. 根据相机内外参矩阵,将地面点坐标转换为相机坐标系下的坐标。
2. 根据相机内参矩阵和相机坐标系下的坐标,计算出相应的像素坐标。
3. 根据像素坐标,计算出对应的像素行列号。
因为这个过程涉及到相机内外参矩阵的应用,所以无法直接在这段代码中实现。需要根据具体的相机模型和参数进行相应的计算。
阅读全文
相关推荐
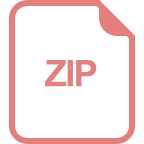
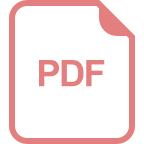



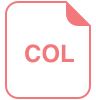
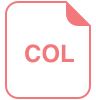
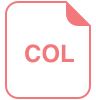
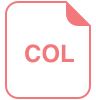
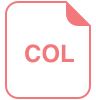

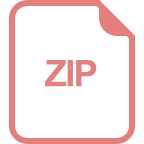
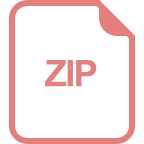
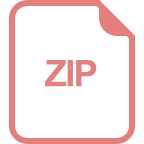
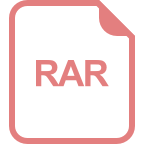
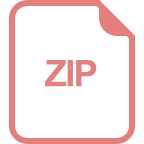