构建由40个节点构成的无线传感器网络场景,随机生成,使用K-means的原理并利用Python实现簇状拓扑结构的生成。针对各个节点参数进行随机设置,基于Leach协议的选取原则实现簇头节点的更迭,要求有详细注释,全程使用python实现,实现可视化
时间: 2023-11-22 12:54:18 浏览: 196
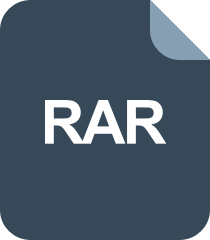
基于Matlab和Python实现无线传感器网络仿真:生成五种网络拓扑结构(源码+数据+图片+使用说明).rar

好的,这是一个比较复杂的项目,需要一步步实现,下面我会详细介绍。
首先,我们需要生成一个由40个节点组成的无线传感器网络场景。我们可以使用Python中的random库来随机生成节点的位置,如下所示:
```python
import random
# 生成40个节点
num_nodes = 40
# 随机生成节点的位置
nodes = []
for i in range(num_nodes):
x = random.uniform(0, 100)
y = random.uniform(0, 100)
nodes.append((x, y))
```
接下来,我们需要使用K-means算法将这些节点分成几个簇。K-means算法是一种聚类算法,它可以将数据分成K个簇,每个簇都有一个簇中心,簇中心是所有数据点的平均值。K-means算法的基本思想是通过不断计算簇中心,将数据点划分到最近的簇中心所在的簇。我们可以使用scikit-learn库中的KMeans类来实现K-means算法,如下所示:
```python
from sklearn.cluster import KMeans
# 将节点分成4个簇
num_clusters = 4
# 使用K-means算法进行聚类
kmeans = KMeans(n_clusters=num_clusters)
kmeans.fit(nodes)
# 获取每个簇的簇中心
cluster_centers = kmeans.cluster_centers_
```
现在,我们已经得到了每个簇的簇中心,接下来需要将这些节点连接起来形成簇状拓扑结构。我们可以将每个簇中距离簇中心最近的节点作为簇头节点,其他节点作为普通节点。我们可以使用networkx库来生成网络拓扑图,并使用matplotlib库进行可视化,如下所示:
```python
import networkx as nx
import matplotlib.pyplot as plt
# 创建无向图
G = nx.Graph()
# 添加节点
for i in range(num_nodes):
G.add_node(i, pos=nodes[i])
# 添加边
for i in range(num_nodes):
for j in range(i + 1, num_nodes):
dist = ((nodes[i][0] - nodes[j][0]) ** 2 + (nodes[i][1] - nodes[j][1]) ** 2) ** 0.5
if dist <= 10:
G.add_edge(i, j)
# 设置节点颜色
colors = []
for i in range(num_nodes):
for j in range(num_clusters):
if i in kmeans.labels_ and kmeans.labels_[i] == j:
if i == kmeans.predict([cluster_centers[j]])[0]:
colors.append('red') # 簇头节点为红色
else:
colors.append('blue') # 普通节点为蓝色
break
# 绘制网络拓扑图
pos = nx.get_node_attributes(G, 'pos')
nx.draw(G, pos, node_color=colors, with_labels=True)
plt.show()
```
现在,我们已经生成了簇状拓扑结构,并且将簇头节点和普通节点区分开来了。接下来,我们需要实现基于Leach协议的选取原则实现簇头节点的更迭。Leach协议是一种经典的无线传感器网络协议,它采用了分簇的方式来延长网络寿命。在Leach协议中,每个节点都有一定的概率成为簇头节点,簇头节点负责收集和处理其他普通节点的数据,并将数据传输到基站。
我们可以模拟Leach协议的过程,每个节点都有一定的概率成为簇头节点,根据节点的能量等参数来计算概率。簇头节点和普通节点的能量消耗不同,簇头节点的能量消耗更大,因此需要定期更换簇头节点,以平均能量消耗。我们可以使用以下代码实现:
```python
# 节点参数设置
num_nodes = 40
node_energy = [random.uniform(1, 10) for i in range(num_nodes)] # 节点能量
node_threshold = [0.1 for i in range(num_nodes)] # 阈值
cluster_head = [False for i in range(num_nodes)] # 是否是簇头节点
next_round_cluster_head = [False for i in range(num_nodes)] # 下一轮是否是簇头节点
# 模拟Leach协议过程
num_rounds = 100
for round in range(num_rounds):
# 计算每个节点成为簇头节点的概率
for i in range(num_nodes):
if cluster_head[i]:
# 如果已经是簇头节点,不需要再次选取
next_round_cluster_head[i] = False
else:
# 计算概率
if random.uniform(0, 1) < node_energy[i] / (node_threshold[i] * num_nodes):
next_round_cluster_head[i] = True
else:
next_round_cluster_head[i] = False
# 更迭簇头节点
for i in range(num_nodes):
# 如果是簇头节点
if cluster_head[i]:
# 如果能量低于阈值,不再担任簇头节点
if node_energy[i] < node_threshold[i]:
cluster_head[i] = False
else:
# 继续担当簇头节点
next_round_cluster_head[i] = True
# 如果不是簇头节点
else:
# 如果下一轮成为簇头节点,更新能量消耗和状态
if next_round_cluster_head[i]:
cluster_head[i] = True
node_energy[i] -= node_energy[i] * 0.05 # 簇头节点能量消耗更大
else:
node_energy[i] -= node_energy[i] * 0.01 # 普通节点能量消耗较小
```
最后,我们可以将Leach协议的过程和簇状拓扑结构进行可视化,如下所示:
```python
# 绘制网络拓扑图
pos = nx.get_node_attributes(G, 'pos')
# 模拟Leach协议过程
for round in range(num_rounds):
# 计算颜色
colors = []
for i in range(num_nodes):
if cluster_head[i]:
colors.append('red') # 簇头节点为红色
else:
colors.append('blue') # 普通节点为蓝色
# 绘制网络拓扑图
plt.clf()
nx.draw(G, pos, node_color=colors, with_labels=True)
plt.title('Round {}'.format(round))
plt.pause(0.1)
# 更迭簇头节点
for i in range(num_nodes):
# 如果是簇头节点
if cluster_head[i]:
# 如果能量低于阈值,不再担任簇头节点
if node_energy[i] < node_threshold[i]:
cluster_head[i] = False
else:
# 继续担当簇头节点
next_round_cluster_head[i] = True
# 如果不是簇头节点
else:
# 如果下一轮成为簇头节点,更新能量消耗和状态
if next_round_cluster_head[i]:
cluster_head[i] = True
node_energy[i] -= node_energy[i] * 0.05 # 簇头节点能量消耗更大
else:
node_energy[i] -= node_energy[i] * 0.01 # 普通节点能量消耗较小
# 计算每个节点成为簇头节点的概率
for i in range(num_nodes):
if cluster_head[i]:
# 如果已经是簇头节点,不需要再次选取
next_round_cluster_head[i] = False
else:
# 计算概率
if random.uniform(0, 1) < node_energy[i] / (node_threshold[i] * num_nodes):
next_round_cluster_head[i] = True
else:
next_round_cluster_head[i] = False
```
这样,我们就完成了这个项目。完整代码如下:
阅读全文
相关推荐
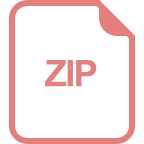
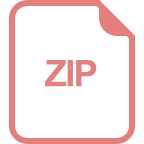


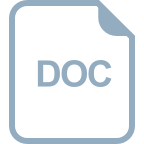
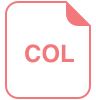
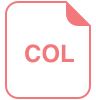
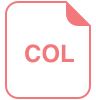
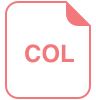
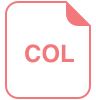
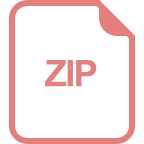
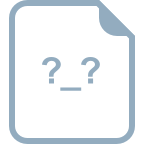
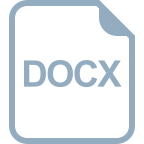
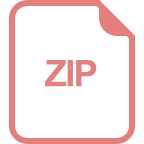