以下代码的含义def generateRules(L, support_data, minConf=0.3,minlif=1.0): bigRuleList = [] for i in range(1, len(L)): for freqSet in L[i]: # 对于每一个频繁项集的集合freqSet H1 = [frozenset([item]) for item in freqSet] # 如果频繁项集中的元素个数大于2,需要进一步合并 if i > 1: rulesFromConseq(freqSet, H1, support_data, bigRuleList, minConf,minlif) else: calcConf(freqSet, H1, support_data, bigRuleList, minConf,minlif) return bigRuleList
时间: 2024-02-14 10:33:32 浏览: 18
这段代码是用来生成关联规则的。输入参数包括频繁项集列表L、支持度数据support_data、最小置信度minConf和最小提升度minlif。对于每个频繁项集freqSet,该函数生成所有可能的关联规则,其中antecedent是freqSet中的一个元素集合,consequent则是freqSet减去antecedent的结果集合。如果i大于1,即频繁项集中的元素个数大于2,那么需要进一步合并,通过调用rulesFromConseq函数来完成。否则,直接通过调用calcConf函数计算关联规则的置信度和提升度。最后,该函数返回所有的关联规则列表bigRuleList。
相关问题
解释以下代码含义dataset = load_dataset() L, support_data = apriori(dataset, min_support=0.01) bigRuleList = generateRules(L, support_data, minConf=0.3,minlif=1.0) print(bigRuleList) def find_rules_containing_item(rules_list, item): # Given a list of rules and an item, # returns a list of rules that contain the item return [rule for rule in rules_list if item in rule[0] or item in rule[1]] item_to_search = 'beef' rules_containing_item = find_rules_containing_item(bigRuleList, item_to_search) for rule in rules_containing_item: print(f"{rule[0]} ==> {rule[1]} 置信度: {rule[2]} 提升度: {rule[3]}")
这段代码是用来实现关联规则挖掘的。首先,通过load_dataset函数加载数据集。然后,通过调用apriori函数计算频繁项集和支持度数据,并将它们存储在变量L和support_data中。接下来,通过调用generateRules函数生成关联规则,并将它们存储在变量bigRuleList中。最后,通过调用find_rules_containing_item函数查找包含特定项的关联规则,并输出这些规则的前件和后件以及它们的置信度和提升度。
具体来说,find_rules_containing_item函数接受一个规则列表和一个项作为输入参数,返回一个包含所有包含该项的规则的列表。在这个例子中,项是'beef'。然后,通过for循环遍历rules_containing_item,输出每个规则的前件和后件,以及它们的置信度和提升度。
以下代码含义def calcConf(freqSet, H, support_data, bigRuleList, minConf,minlif): prunedH = [] for conseq in H: conf = support_data[freqSet] / support_data[freqSet - conseq] lift = support_data[freqSet] / (support_data[conseq] * support_data[freqSet - conseq]) if conf >= minConf and lift >= minlif: bigRuleList.append((freqSet - conseq, conseq, conf,lift)) prunedH.append(conseq) return prunedH
这段代码是用来计算关联规则的置信度和提升度的。输入参数包括频繁项集freqSet、可能的后件项集合H、支持度数据support_data、所有关联规则列表bigRuleList、最小置信度minConf和最小提升度minlif。对于每个后件项集合conseq,该函数计算关联规则的置信度及提升度,如果满足最小置信度和最小提升度的条件,就将该关联规则添加到bigRuleList中。同时,prunedH用于记录满足条件的后件项集合。最后,该函数返回满足条件的后件项集合列表prunedH。
相关推荐
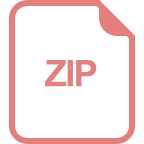
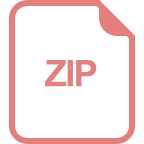
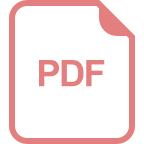












