import cv2 import numpy as np import matplotlib.pyplot as plt from flask import Flask, request, jsonify app = Flask(name) @app.route('/', methods=['POST']) def predict(): # 读入图片 image = request.files.get('image') img = cv2.imdecode(np.fromstring(image.read(), np.uint8), cv2.IMREAD_COLOR) # 获取图片长宽 height, width = img.shape[:2] # 计算每个圆的半径 width = max(width, height) height = min(width, height) a = int(width / 12) / 2 b = int(height / 8) / 2 c = int(a) d = int(b) r = min(c, d) # 计算圆心坐标 centers = [] for j in range(8): for i in range(12): cx = 2 * r * j + r cy = 2 * r * i + r centers.append((cx, cy)) # 提取灰度值 gray_values = [] for i in range(96): x, y = centers[i][0], centers[i][1] mask = np.zeros_like(img) cv2.circle(mask, (x, y), r, (255, 255, 255), -1) masked_img = cv2.bitwise_and(img, mask) gray_img = cv2.cvtColor(masked_img, cv2.COLOR_RGB2GRAY) gray_value = np.mean(gray_img) gray_values.append(gray_value) # 拟合数据 x_values = gray_values[:16] # 16个用于训练的灰度值 x_prediction_values = gray_values[16:] # 80个用于预测的灰度值 y_values = [0.98, 0.93, 0.86, 0.79, 0.71, 0.64, 0.57, 0.50, 0.43, 0.36, 0.29, 0.21, 0.14, 0.07, 0.05, 0.01] # 16个液体浓度值 # 使用numpy的polyfit函数进行线性拟合 fit = np.polyfit(x_values, y_values, 1) # 使用拟合系数构建线性函数 lin_func = np.poly1d(fit) # 生成新的80个数据的x值 new_x = x_prediction_values # 预测新的80个数据的y值 new_y = lin_func(new_x) # 输出预测结果 result = list(new_y) row3 = result[:8] row4 = result[8:16] row5 = result[16:24] row6 = result[24:32] row7 = result[32:40] row8 = result[40:48] row9 = result[48:56] row10 = result[56:64] row11 = result[64:72] row12 = result[72:80] prediction_result = { "第三列": row3, "第四列": row4, "第五列": row5, "第六列": row6, "第七列": row7, "第八列": row8, "第九列": row9, "第十列": row10, "第十一列": row11, "第十二列": row12 } return jsonify(prediction_result) if name == 'main': app.run(debug=True) 请问上面这段代码如何在本地运行呀?
时间: 2023-12-28 11:05:36 浏览: 36
可以在本地安装Flask和OpenCV等相关库后,将代码保存为一个.py文件。在终端中进入该文件所在目录,运行以下命令即可启动Web应用程序:
```
export FLASK_APP=your_file_name.py
flask run
```
其中,`your_file_name.py`是你保存的文件名。在Windows系统中,使用`set`代替`export`。运行成功后,在浏览器中输入`http://localhost:5000/`即可访问该Web应用程序。
相关问题
import cv2 import numpy as np import matplotlib.pyplot as plt from flask import Flask, request, jsonify app = Flask(name) @app.route('/', methods=['POST']) def predict(): # 读入图片 image = request.files.get('image') img = cv2.imdecode(np.fromstring(image.read(), np.uint8), cv2.IMREAD_COLOR) # 获取图片长宽 height, width = img.shape[:2] # 计算每个圆的半径 width = max(width, height) height = min(width, height) a = int(width / 12) / 2 b = int(height / 8) / 2 c = int(a) d = int(b) r = min(c, d) # 计算圆心坐标 centers = [] for j in range(8): for i in range(12): cx = 2 * r * j + r cy = 2 * r * i + r centers.append((cx, cy)) # 提取灰度值 gray_values = [] for i in range(96): x, y = centers[i][0], centers[i][1] mask = np.zeros_like(img) cv2.circle(mask, (x, y), r, (255, 255, 255), -1) masked_img = cv2.bitwise_and(img, mask) gray_img = cv2.cvtColor(masked_img, cv2.COLOR_RGB2GRAY) gray_value = np.mean(gray_img) gray_values.append(gray_value) # 拟合数据 x_values = gray_values[:16] # 16个用于训练的灰度值 x_prediction_values = gray_values[16:] # 80个用于预测的灰度值 y_values = [0.98, 0.93, 0.86, 0.79, 0.71, 0.64, 0.57, 0.50, 0.43, 0.36, 0.29, 0.21, 0.14, 0.07, 0.05, 0.01] # 16个液体浓度值 # 使用numpy的polyfit函数进行线性拟合 fit = np.polyfit(x_values, y_values, 1) # 使用拟合系数构建线性函数 lin_func = np.poly1d(fit) # 生成新的80个数据的x值 new_x = x_prediction_values # 预测新的80个数据的y值 new_y = lin_func(new_x) # 输出预测结果 result = list(new_y) row3 = result[:8] row4 = result[8:16] row5 = result[16:24] row6 = result[24:32] row7 = result[32:40] row8 = result[40:48] row9 = result[48:56] row10 = result[56:64] row11 = result[64:72] row12 = result[72:80] prediction_result = { "第三列": row3, "第四列": row4, "第五列": row5, "第六列": row6, "第七列": row7, "第八列": row8, "第九列": row9, "第十列": row10, "第十一列": row11, "第十二列": row12 } return jsonify(prediction_result) if name == 'main': app.run(debug=True) 请问上面这段代码如何运行呀?
这段代码是一个使用 Flask 框架实现的简单的 Web API,可以接收客户端传递的图片数据,对图片进行处理,返回预测结果。如果要运行这段代码,可以按照以下步骤进行:
1. 安装必要的依赖库:这段代码依赖于 OpenCV、NumPy 和 Flask 库,需要先安装这些库,可以使用 pip 命令进行安装,如下所示:
```
pip install opencv-python numpy flask
```
2. 将代码保存为一个名为 `app.py` 的文件。
3. 在命令行中进入代码所在目录,执行 `python app.py` 命令,即可启动 Flask 应用。
4. 使用客户端工具(如 Postman)向该应用发送 POST 请求,请求路径为 `/`,请求参数中包含一个名为 `image` 的文件,该文件是待处理的图片数据。
5. 应用会对图片进行处理,并返回一个 JSON 格式的预测结果,包含了每列的液体浓度值。
需要注意的是,这段代码中的液体浓度预测算法是基于一定的模型和训练数据得到的,如果要使用该代码进行实际应用,需要根据具体情况进行调整和优化。
import cv2 import numpy as np import matplotlib.pyplot as plt def liquid_concentration_prediction(image_path): # 读入图片 img = cv2.imread(image_path) # 获取图片长宽 height, width = img.shape[:2] # 计算每个圆的半径 width = max(width, height) height = min(width, height) a = int(width / 12) / 2 b = int(height / 8) / 2 c = int(a) d = int(b) r = min(c, d) # 计算圆心坐标 centers = [] for j in range(8): for i in range(12): cx = 2 * r * j + r cy = 2 * r * i + r centers.append((cx, cy)) # 提取灰度值 gray_values = [] for i in range(96): x, y = centers[i][0], centers[i][1] mask = np.zeros_like(img) cv2.circle(mask, (x, y), r, (255, 255, 255), -1) masked_img = cv2.bitwise_and(img, mask) gray_img = cv2.cvtColor(masked_img, cv2.COLOR_RGB2GRAY) gray_value = np.mean(gray_img) gray_values.append(gray_value) # 拟合数据 x_values = gray_values[:16] # 16个用于训练的灰度值 x_prediction_values = gray_values[16:] # 80个用于预测的灰度值 y_values = [0.98, 0.93, 0.86, 0.79, 0.71, 0.64, 0.57, 0.50, 0.43, 0.36, 0.29, 0.21, 0.14, 0.07, 0.05, 0.01] # 16个液体浓度值 # 使用numpy的polyfit函数进行线性拟合 fit = np.polyfit(x_values, y_values, 1) # 使用拟合系数构建线性函数 lin_func = np.poly1d(fit) # 生成新的80个数据的x值 new_x = x_prediction_values # 预测新的80个数据的y值 new_y = lin_func(new_x) # 输出预测结果 result = list(new_y) row3 = result[:8] row4 = result[8:16] row5 = result[16:24] row6 = result[24:32] row7 = result[32:40] row8 = result[40:48] row9 = result[48:56] row10 = result[56:64] row11 = result[64:72] row12 = result[72:80] print("第三列:", row3) print("第四列:", row4) print("第五列:", row5) print("第六列:", row6) print("第七列:", row7) print("第八列:", row8) print("第九列:", row9) print("第十列:", row10) print("第十一列:", row11) print("第十二列:", row12) 请把上面的代码用Flask框架生成一个网址
好的,以下是用 Flask 框架生成一个可以预测液体浓度的网址的代码:
```python
import cv2
import numpy as np
import matplotlib.pyplot as plt
from flask import Flask, request, jsonify
app = Flask(__name__)
@app.route('/', methods=['POST'])
def predict():
# 读入图片
image = request.files.get('image')
img = cv2.imdecode(np.fromstring(image.read(), np.uint8), cv2.IMREAD_COLOR)
# 获取图片长宽
height, width = img.shape[:2]
# 计算每个圆的半径
width = max(width, height)
height = min(width, height)
a = int(width / 12) / 2
b = int(height / 8) / 2
c = int(a)
d = int(b)
r = min(c, d)
# 计算圆心坐标
centers = []
for j in range(8):
for i in range(12):
cx = 2 * r * j + r
cy = 2 * r * i + r
centers.append((cx, cy))
# 提取灰度值
gray_values = []
for i in range(96):
x, y = centers[i][0], centers[i][1]
mask = np.zeros_like(img)
cv2.circle(mask, (x, y), r, (255, 255, 255), -1)
masked_img = cv2.bitwise_and(img, mask)
gray_img = cv2.cvtColor(masked_img, cv2.COLOR_RGB2GRAY)
gray_value = np.mean(gray_img)
gray_values.append(gray_value)
# 拟合数据
x_values = gray_values[:16] # 16个用于训练的灰度值
x_prediction_values = gray_values[16:] # 80个用于预测的灰度值
y_values = [0.98, 0.93, 0.86, 0.79, 0.71, 0.64, 0.57, 0.50, 0.43, 0.36, 0.29, 0.21, 0.14, 0.07, 0.05, 0.01] # 16个液体浓度值
# 使用numpy的polyfit函数进行线性拟合
fit = np.polyfit(x_values, y_values, 1)
# 使用拟合系数构建线性函数
lin_func = np.poly1d(fit)
# 生成新的80个数据的x值
new_x = x_prediction_values
# 预测新的80个数据的y值
new_y = lin_func(new_x)
# 输出预测结果
result = list(new_y)
row3 = result[:8]
row4 = result[8:16]
row5 = result[16:24]
row6 = result[24:32]
row7 = result[32:40]
row8 = result[40:48]
row9 = result[48:56]
row10 = result[56:64]
row11 = result[64:72]
row12 = result[72:80]
prediction_result = {
"第三列": row3,
"第四列": row4,
"第五列": row5,
"第六列": row6,
"第七列": row7,
"第八列": row8,
"第九列": row9,
"第十列": row10,
"第十一列": row11,
"第十二列": row12
}
return jsonify(prediction_result)
if __name__ == '__main__':
app.run(debug=True)
```
启动 Flask 应用程序后,你可以向 `http://localhost:5000` 发送 POST 请求,请求体中包含一个名为 `image` 的文件,表示要预测的图片。应用程序将返回一个 JSON 对象,其中键值对表示预测结果。
相关推荐
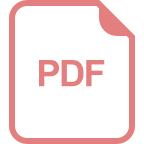
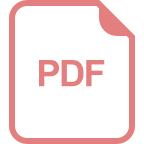













