分别使用keras-gpu和pytorch搭建cnn-GRU-attention预测负荷
时间: 2023-08-08 14:06:32 浏览: 207
使用 Keras-GPU 搭建 CNN-GRU-Attention 模型:
首先导入必要的库:
```
import numpy as np
import pandas as pd
import keras.backend as K
from keras.models import Model
from keras.layers import Input, Dense, Embedding, Conv1D, MaxPooling1D, GRU, Bidirectional, TimeDistributed, Flatten, Dropout, Lambda
```
接着加载数据:
```
# 加载数据
data = pd.read_csv('data.csv')
# 分割特征和标签
X = data.iloc[:, :-1].values
y = data.iloc[:, -1].values
# 将标签转换为one-hot编码
y = pd.get_dummies(y).values
```
构建模型:
```
def cnn_gru_att():
input_layer = Input(shape=(X.shape[1],))
# embedding层
emb = Embedding(input_dim=VOCAB_SIZE, output_dim=EMB_SIZE)(input_layer)
# CNN层
conv1 = Conv1D(filters=64, kernel_size=3, activation='relu', padding='same')(emb)
pool1 = MaxPooling1D(pool_size=2)(conv1)
conv2 = Conv1D(filters=128, kernel_size=3, activation='relu', padding='same')(pool1)
pool2 = MaxPooling1D(pool_size=2)(conv2)
conv3 = Conv1D(filters=256, kernel_size=3, activation='relu', padding='same')(pool2)
pool3 = MaxPooling1D(pool_size=2)(conv3)
# GRU层
gru = Bidirectional(GRU(units=128, return_sequences=True))(pool3)
# Attention层
attention = TimeDistributed(Dense(1, activation='tanh'))(gru)
attention = Flatten()(attention)
attention = Lambda(lambda x: K.softmax(x))(attention)
attention = RepeatVector(256)(attention)
attention = Permute([2, 1])(attention)
# 加权求和
sent_representation = Multiply()([gru, attention])
sent_representation = Lambda(lambda xin: K.sum(xin, axis=-2), output_shape=(256,))(sent_representation)
# 全连接层
fc1 = Dense(units=256, activation='relu')(sent_representation)
fc2 = Dense(units=128, activation='relu')(fc1)
output_layer = Dense(units=NUM_CLASSES, activation='softmax')(fc2)
model = Model(inputs=input_layer, outputs=output_layer)
return model
```
使用 PyTorch 搭建 CNN-GRU-Attention 模型:
首先导入必要的库:
```
import torch
import torch.nn as nn
import torch.nn.functional as F
```
接着定义模型:
```
class CNN_GRU_ATT(nn.Module):
def __init__(self, vocab_size, emb_size, num_filters, kernel_sizes, hidden_size, num_classes, dropout_rate):
super(CNN_GRU_ATT, self).__init__()
# embedding层
self.embedding = nn.Embedding(vocab_size, emb_size)
# CNN层
self.convs = nn.ModuleList([nn.Conv1d(in_channels=emb_size, out_channels=num_filters, kernel_size=ks) for ks in kernel_sizes])
# GRU层
self.gru = nn.GRU(input_size=num_filters*len(kernel_sizes), hidden_size=hidden_size, bidirectional=True, batch_first=True)
# Attention层
self.attention_layer = nn.Linear(hidden_size*2, 1)
# 全连接层
self.fc1 = nn.Linear(hidden_size*2, hidden_size)
self.fc2 = nn.Linear(hidden_size, num_classes)
# Dropout层
self.dropout = nn.Dropout(dropout_rate)
def forward(self, x):
# embedding层
embedded = self.embedding(x)
# CNN层
conv_outputs = []
for conv in self.convs:
conv_output = F.relu(conv(embedded.transpose(1, 2)))
pooled_output = F.max_pool1d(conv_output, conv_output.size(2)).squeeze(2)
conv_outputs.append(pooled_output)
cnn_output = torch.cat(conv_outputs, dim=1)
# GRU层
gru_output, _ = self.gru(cnn_output.unsqueeze(0))
gru_output = gru_output.squeeze(0)
# Attention层
attention_weights = F.softmax(self.attention_layer(gru_output), dim=0)
attention_output = (gru_output * attention_weights).sum(dim=0)
# 全连接层
fc1_output = self.dropout(F.relu(self.fc1(attention_output)))
fc2_output = self.fc2(fc1_output)
return fc2_output
```
以上是使用 Keras-GPU 和 PyTorch 搭建 CNN-GRU-Attention 模型的示例代码,需要根据具体的任务修改模型参数和数据处理方式。
阅读全文
相关推荐
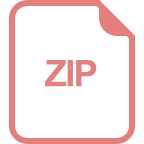
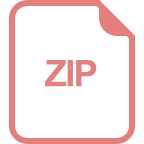
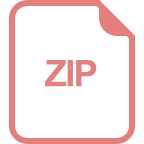
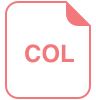
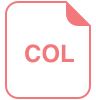
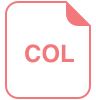
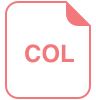
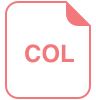
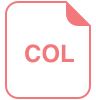
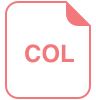
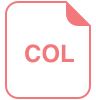
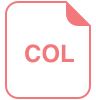
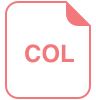
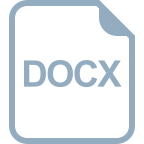
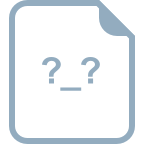