pycharm中使用object_points = np.append(object_points, [object_points[0]], axis = 0)前7个数组没问题填充第8数组时整个数组长度变为0为什么
时间: 2024-05-06 12:14:52 浏览: 54
这个问题可能是由于您在使用`np.append()`函数时没有指定正确的参数导致的。具体来说,`np.append()`函数的第一个参数是要附加的数组,第二个参数是要附加到数组的值,第三个参数是指定附加的轴。在您的代码中,您使用了`axis=0`参数,这意味着您要将一个形状相同的数组附加到`object_points`数组的第一维。但是,您的代码中使用了`[object_points[0]]`作为要附加的值,这是一个形状为`(1, 3)`的数组,它的第一维长度为1,与`object_points`数组的长度不同。
解决方案是将要附加的数组的形状与`object_points`数组的形状相同。您可以使用`np.array()`函数将`[object_points[0]]`转换为形状为`(1, 3)`的数组,然后再将其附加到`object_points`数组中。修改后的代码如下:
```
object_points = np.append(object_points, np.array([object_points[0]]), axis=0)
```
这应该可以解决您遇到的问题。
相关问题
pycharm中使用object_points = np.append(object_points, [object_points[0]], axis = 0)填充第8数组时整个数组长度变为0为什么
可能是因为在使用 `np.append()` 函数时,未正确指定 `axis` 参数。如果 `axis=0`,则表示在第一个维度上添加元素,即在行方向上添加元素;如果 `axis=1`,则表示在第二个维度上添加元素,即在列方向上添加元素。如果 `axis` 参数设置不正确,就会导致数组长度变为0。请检查代码中 `axis` 参数是否正确设置。
pycharm安装opencv_python_headless==4.5.5.62
可以通过以下命令在 PyCharm 中安装 opencv_python_headless==4.5.5.62:
pip install opencv_python_headless==4.5.5.62
请确保您的 PyCharm 环境已经正确配置了 Python 和 pip。
相关推荐
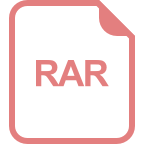
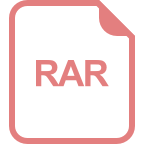













