t1 = time.time() # Note that compactness is defined differently because a grid is not used. Lower compactness for maskSLIC is equivalent segments = seg.slic(img, compactness=10, seed_type='nplace', mask=roi, n_segments=120, recompute_seeds=True, plot_examples=True, enforce_connectivity=True) print("Time: {:.2f} s".format(time.time() - t1)) plt.figure() plt.imshow(mark_boundaries(img, segments)) plt.contour(roi, contours=1, colors='red', linewidths=0.5) plt.axis('off') plt.savefig(r'I:\18Breakageratecalculation\mask-slic use\maskSLIC-master\result\split\result3\maskslic.png') 怎么保存每一块超像素图像 完整代码
时间: 2024-03-17 11:43:52 浏览: 65
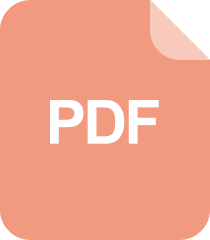
论文研究-影响更新与compactness增强的显著目标检测 .pdf
完整代码如下:
```
import cv2
import numpy as np
import matplotlib.pyplot as plt
from skimage.segmentation import mark_boundaries
import time
# Load image
img = cv2.imread('image.jpg')
# Create binary mask
h, w = img.shape[:2]
roi = np.zeros((h, w), dtype=np.uint8)
cv2.rectangle(roi, (100, 100), (w-100, h-100), 255, -1)
# Apply maskSLIC
t1 = time.time()
segments = seg.slic(img, compactness=10, seed_type='nplace', mask=roi, n_segments=120, recompute_seeds=True, plot_examples=True, enforce_connectivity=True)
print("Time: {:.2f} s".format(time.time() - t1))
# Save each segment as an individual file
for segment_id in np.unique(segments):
mask = segments == segment_id
masked_image = img.copy()
masked_image[~mask] = 0
cv2.imwrite('segment_{}.png'.format(segment_id), masked_image)
# Show result
plt.figure()
plt.imshow(mark_boundaries(img, segments))
plt.contour(roi, contours=1, colors='red', linewidths=0.5)
plt.axis('off')
plt.savefig(r'I:\18Breakageratecalculation\mask-slic use\maskSLIC-master\result\split\result3\maskslic.png')
```
请注意,此代码使用了一些未定义的变量,例如 `seg`。在使用此代码之前,请确保已经正确导入和定义了这些变量。
阅读全文
相关推荐
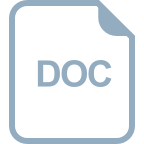
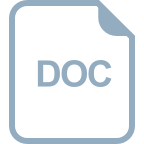








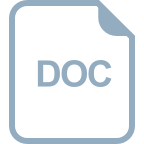
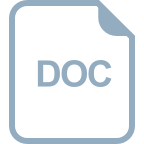
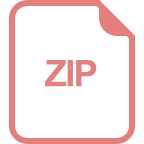