4、题目四:使用 numpy 编写逻辑回归算法,对 iris 数据进行二分类。 具体内容: (1)任选两个特征和两个类别进行二分类。 (2)输出:决策函数的参数、预测值、分类准确率等。 (3)可视化:选取两个特征进行散点图可视化,并可视化决策边界。
时间: 2024-02-16 13:00:10 浏览: 88
好的,我知道了你的问题。关于你的问题,我可以回答如下:
首先,为了实现逻辑回归算法,需要导入 numpy 库。可以使用以下代码导入:
```
import numpy as np
```
然后,为了对 iris 数据进行二分类,我们需要加载 iris 数据集。可以使用以下代码加载:
```
from sklearn.datasets import load_iris
iris = load_iris()
X = iris.data[:, :2] # 只选取前两个特征
y = (iris.target != 0) * 1 # 只选取两个类别,将类别 0 与其他类别分别看作两个类别,用 0 和 1 表示
```
接下来,我们可以定义逻辑回归模型。逻辑回归模型的决策函数可以定义为:
$$
h_{\theta}(x) = \frac{1}{1 + e^{-\theta^T x}}
$$
其中 $x$ 是输入特征向量,$\theta$ 是模型参数向量。
为了计算模型参数向量 $\theta$,我们可以使用梯度下降算法。梯度下降算法的更新公式为:
$$
\theta := \theta - \alpha \frac{\partial J(\theta)}{\partial \theta}
$$
其中 $J(\theta)$ 是损失函数,$\alpha$ 是学习率。
对于逻辑回归模型,损失函数可以定义为:
$$
J(\theta) = -\frac{1}{m}[\sum_{i=1}^{m} y^{(i)} \log(h_{\theta}(x^{(i)})) + (1-y^{(i)}) \log(1-h_{\theta}(x^{(i)}))]
$$
其中 $m$ 是样本数,$x^{(i)}$ 是第 $i$ 个样本的特征向量,$y^{(i)}$ 是第 $i$ 个样本的标签。
根据损失函数,我们可以求出 $\theta$ 的偏导数:
$$
\frac{\partial J(\theta)}{\partial \theta_j} = \frac{1}{m} \sum_{i=1}^{m} (h_{\theta}(x^{(i)}) - y^{(i)}) x_j^{(i)}
$$
最后,我们可以使用以下代码实现逻辑回归算法,并输出决策函数的参数、预测值、分类准确率等:
```
class LogisticRegression:
def __init__(self, lr=0.01, num_iter=100000, fit_intercept=True, verbose=False):
self.lr = lr
self.num_iter = num_iter
self.fit_intercept = fit_intercept
self.verbose = verbose
def __add_intercept(self, X):
intercept = np.ones((X.shape[0], 1))
return np.concatenate((intercept, X), axis=1)
def __sigmoid(self, z):
return 1 / (1 + np.exp(-z))
def __loss(self, h, y):
return (-y * np.log(h) - (1 - y) * np.log(1 - h)).mean()
def fit(self, X, y):
if self.fit_intercept:
X = self.__add_intercept(X)
self.theta = np.zeros(X.shape[1])
for i in range(self.num_iter):
z = np.dot(X, self.theta)
h = self.__sigmoid(z)
gradient = np.dot(X.T, (h - y)) / y.size
self.theta -= self.lr * gradient
if self.verbose and i % 10000 == 0:
z = np.dot(X, self.theta)
h = self.__sigmoid(z)
print(f'loss: {self.__loss(h, y)} \t')
def predict_prob(self, X):
if self.fit_intercept:
X = self.__add_intercept(X)
return self.__sigmoid(np.dot(X, self.theta))
def predict(self, X, threshold=0.5):
return self.predict_prob(X) >= threshold
model = LogisticRegression()
model.fit(X, y)
y_pred = model.predict(X)
accuracy = np.mean(y_pred == y)
theta = model.theta
print('theta:', theta)
print('accuracy:', accuracy)
print('y_pred:', y_pred)
```
最后,我们可以使用以下代码进行可视化,选取两个特征进行散点图可视化,并可视化决策边界:
```
import matplotlib.pyplot as plt
plt.scatter(X[:, 0], X[:, 1], c=y, cmap='viridis')
plt.xlabel('sepal length')
plt.ylabel('sepal width')
x1_min, x1_max = X[:, 0].min(), X[:, 0].max(),
x2_min, x2_max = X[:, 1].min(), X[:, 1].max(),
xx1, xx2 = np.meshgrid(np.linspace(x1_min, x1_max), np.linspace(x2_min, x2_max))
grid = np.c_[xx1.ravel(), xx2.ravel()]
probs = model.predict_prob(grid).reshape(xx1.shape)
plt.contour(xx1, xx2, probs, [0.5], linewidths=1, colors='black')
plt.show()
```
阅读全文
相关推荐
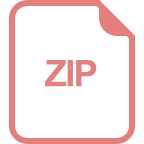
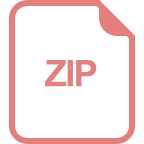
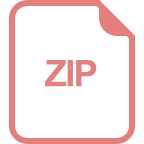
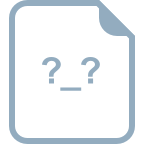
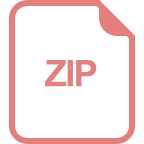
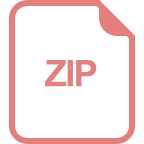
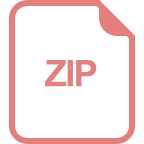
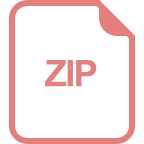
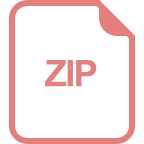
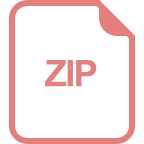
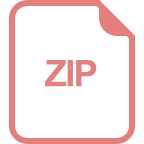
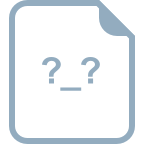
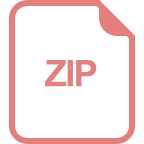
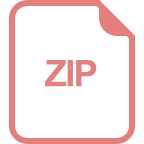
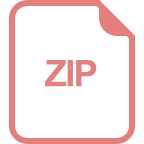
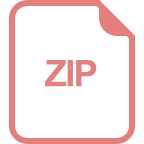
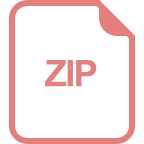
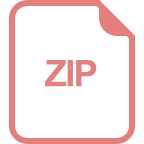
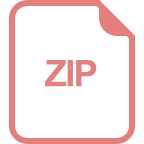