pytorch中注意力机制
时间: 2023-08-30 17:09:11 浏览: 47
在PyTorch中,注意力机制可以通过使用`torch.nn.MultiheadAttention`模块来实现。这个模块实现了多头自注意力机制,也就是在同一个序列中不同位置之间的注意力机制。
下面是一个使用`torch.nn.MultiheadAttention`模块的简单示例:
```python
import torch.nn as nn
# 定义输入维度和输出维度
input_dim = 64
output_dim = 128
# 定义注意力模块
attention = nn.MultiheadAttention(input_dim, num_heads=8, dropout=0.5)
# 定义输入张量,假设有batch_size个序列,每个序列长度为seq_len,维度为input_dim
input_tensor = torch.randn(batch_size, seq_len, input_dim)
# 过注意力模块
output_tensor, _ = attention(input_tensor, input_tensor, input_tensor)
# 输出张量的维度为(batch_size, seq_len, output_dim)
print(output_tensor.shape)
```
在上面的示例中,我们定义了一个`MultiheadAttention`模块,并将输入张量`input_tensor`通过这个模块。注意力模块的输出张量`output_tensor`的维度为`(batch_size, seq_len, output_dim)`,其中`output_dim`是我们在定义模块时指定的输出维度。
我们还可以通过调整`num_heads`参数来控制注意力头的数量,以及使用`dropout`参数来控制注意力模块的dropout率。
相关推荐
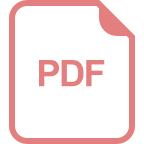
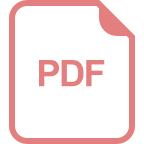
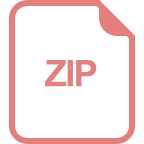














