LAD要用那个python库实现
时间: 2024-06-07 10:08:30 浏览: 25
LAD可以使用多种Python库进行实现,具体选择哪个库取决于你的需求和偏好。以下是一些常用的Python库:
1. NLTK(Natural Language Toolkit):用于自然语言处理的Python库,包含了各种文本处理和分析工具。
2. SpaCy:另一个常用的自然语言处理Python库,速度较快且功能强大。
3. TextBlob:基于NLTK和Pattern库的Python库,提供了易于使用的API,可用于情感分析、文本分类、翻译等。
4. Gensim:用于文本处理和主题建模的Python库,可用于LAD中的主题识别和关键词提取。
5. Scikit-learn:用于机器学习的Python库,包含了各种文本分类和聚类算法,可用于LAD中的分类和聚类。
以上是一些常用的Python库,你可以根据你的具体需求选择适合自己的库进行实现LAD。
相关问题
python LAD库如何下载
要下载 Python LAD 库,可以通过以下步骤进行操作:
1. 打开命令行终端或 Anaconda Prompt。
2. 输入以下命令,使用 pip 安装 lad 命令行工具:
```
pip install lad-cli
```
3. 安装完成后,可以使用以下命令来下载 LAD 库:
```
lad download
```
4. 运行以上命令后,会要求输入 LAD 库的下载链接或关键字,根据提示输入即可。
5. 下载完成后,可以使用以下命令来查看 LAD 库的内容:
```
lad ls
```
6. 如果需要使用 LAD 库的功能,可以使用 Python 脚本进行调用。
```
import lad
# 调用 LAD 库中的函数进行操作
result = lad.some_function()
```
以上就是下载 Python LAD 库的基本步骤,希望对你有所帮助。
python LAD库有什么方法
LAD(Least Absolute Deviations)库是一个Python库,用于解决线性回归问题。它提供了一种解决线性回归问题的替代方法,该方法使用最小绝对偏差(LAD)而不是最小二乘误差(MSE)。
LAD库中有几个重要的方法,包括:
1. LAD.fit(X, y) - 训练模型并拟合数据,其中X是输入特征,y是输出变量。
2. LAD.predict(X) - 预测输出变量,其中X是输入特征。
3. LAD.score(X, y) - 计算模型的R-squared值,其中X是输入特征,y是真实输出变量。
4. LAD.coef_ - 返回模型的权重向量。
5. LAD.intercept_ - 返回模型的截距。
6. LAD.get_params() - 返回模型的参数。
这些方法可以帮助您使用LAD库进行线性回归分析。
相关推荐
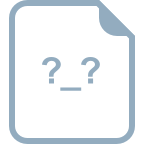
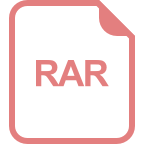












