class Pointnet2MSG(nn.Module): def __init__(self, input_channels=6, use_xyz=True): super(Pointnet2MSG, self).__init__() self.SA_modules = nn.ModuleList() channel_in = input_channels # print("channel_in=", channel_in) skip_channel_list = [input_channels] for k in range(cfg.RPN.SA_CONFIG.NPOINTS.__len__()): mlps = cfg.RPN.SA_CONFIG.MLPS[k].copy() channel_out = 0 # print("channel_in=", channel_in) for idx in range(mlps.__len__()): mlps[idx] = [channel_in] + mlps[idx] channel_out += mlps[idx][-1] mlps.append(channel_out) # print(mlps) print("channel_out", channel_out) self.SA_modules.append( nn.Sequential( PointnetSAModuleMSG( npoint=cfg.RPN.SA_CONFIG.NPOINTS[k], radii=cfg.RPN.SA_CONFIG.RADIUS[k], nsamples=cfg.RPN.SA_CONFIG.NSAMPLE[k], mlps=mlps[:2], use_xyz=use_xyz, bn=cfg.RPN.USE_BN, ), # SelfAttention(channel_out) SelfAttention(int(channel_out/2)) if k == 3 else SelfAttention(channel_out) ) ) skip_channel_list.append(channel_out) channel_in = channel_out想知道以上代码块中的 skip_channel_list存在的目的是什么?
时间: 2023-12-01 13:03:49 浏览: 66
在 Pointnet2MSG 模型中,skip_channel_list 的作用是记录每个 SA(Sampling and Aggregation)模块的输出通道数。这是因为在构建 Pointnet2MSG 模型时,每个 SA 模块的输出通道数会作为下一个 SA 模块的输入通道数。因此,skip_channel_list 中的每个元素都是一个 SA 模块的输出通道数,用于构建后续 SA 模块的输入通道数。在 forward 函数中,skip_channel_list 还被用于将 SA 模块的输出与对应的输入进行拼接。
相关问题
class Pointnet2MSG(nn.Module): def __init__(self, input_channels=6, use_xyz=True): super().__init__() self.SA_modules = nn.ModuleList() channel_in = input_channels skip_channel_list = [input_channels] for k in range(cfg.RPN.SA_CONFIG.NPOINTS.__len__()): mlps = cfg.RPN.SA_CONFIG.MLPS[k].copy() channel_out = 0 for idx in range(mlps.__len__()): mlps[idx] = [channel_in] + mlps[idx] channel_out += mlps[idx][-1] self.SA_modules.append( PointnetSAModuleMSG( npoint=cfg.RPN.SA_CONFIG.NPOINTS[k], radii=cfg.RPN.SA_CONFIG.RADIUS[k], nsamples=cfg.RPN.SA_CONFIG.NSAMPLE[k], mlps=mlps, use_xyz=use_xyz, bn=cfg.RPN.USE_BN ) ) skip_channel_list.append(channel_out) channel_in = channel_out这是我改进之前的类代码块,而这是我加入SA注意力机制后的代码块:class Pointnet2MSG(nn.Module): def __init__(self, input_channels=6, use_xyz=True): super().__init__() self.SA_modules = nn.ModuleList() channel_in = input_channels skip_channel_list = [input_channels] for k in range(cfg.RPN.SA_CONFIG.NPOINTS.__len__()): mlps = cfg.RPN.SA_CONFIG.MLPS[k].copy() channel_out = 0 for idx in range(mlps.__len__()): mlps[idx] = [channel_in] + mlps[idx] channel_out += mlps[idx][-1] mlps.append(channel_out) self.SA_modules.append( nn.Sequential( PointnetSAModuleMSG( npoint=cfg.RPN.SA_CONFIG.NPOINTS[k], radii=cfg.RPN.SA_CONFIG.RADIUS[k], nsamples=cfg.RPN.SA_CONFIG.NSAMPLE[k], mlps=mlps, use_xyz=use_xyz, bn=cfg.RPN.USE_BN, ), SelfAttention(channel_out) ) ) skip_channel_list.append(channel_out) channel_in = channel_out,我发现改进后的代码块对于mlps参数的计算非常混乱,请你帮我检查一下,予以更正并给出注释
在你加入SA注意力机制后的代码块中,你在计算mlps时,添加了一个长度为1的列表,这个列表的元素为channel_out,这样做的目的是为了在SA模块中使用channel_out作为输入特征的通道数。但是这样的话,你的mlps列表就不再是每一层的输出通道数了,而是包含了一个额外的元素channel_out。
为了解决这个问题,你可以在SA模块中获取mlps列表中除了最后一个元素以外的所有元素,并将它们作为SA模块的输入通道数,最后将SA模块的输出通道数设置为mlps列表中最后一个元素即可。
以下是修改后的代码块,已经添加了注释:
```
class Pointnet2MSG(nn.Module):
def __init__(self, input_channels=6, use_xyz=True):
super().__init__()
self.SA_modules = nn.ModuleList()
channel_in = input_channels
skip_channel_list = [input_channels]
for k in range(cfg.RPN.SA_CONFIG.NPOINTS.__len__()):
mlps = cfg.RPN.SA_CONFIG.MLPS[k].copy()
channel_out = 0
for idx in range(mlps.__len__()):
mlps[idx] = [channel_in] + mlps[idx]
channel_out += mlps[idx][-1]
# 移除mlps列表中的最后一个元素,并将其作为SA模块的输出通道数
sa_channel_out = mlps.pop()
self.SA_modules.append(
nn.Sequential(
PointnetSAModuleMSG(
npoint=cfg.RPN.SA_CONFIG.NPOINTS[k],
radii=cfg.RPN.SA_CONFIG.RADIUS[k],
nsamples=cfg.RPN.SA_CONFIG.NSAMPLE[k],
mlps=mlps,
use_xyz=use_xyz,
bn=cfg.RPN.USE_BN,
),
SelfAttention(sa_channel_out, sa_channel_out // 8) # 通常将隐藏层大小设置为输出通道数的1/8
)
)
skip_channel_list.append(sa_channel_out)
channel_in = sa_channel_out
```
class SelfAttention(nn.Module): def __init__(self, in_channels, reduction=4): super(SelfAttention, self).__init__() self.avg_pool = nn.AdaptiveAvgPool1d(1) # print("in_channels", in_channels) print("in_channels:", in_channels) print("reduction:", reduction) self.fc1 = nn.Conv1d(in_channels, in_channels // reduction, 1, bias=False) self.relu = nn.ReLU(inplace=True) self.fc2 = nn.Conv1d(in_channels // reduction, in_channels, 1, bias=False) self.sigmoid = nn.Sigmoid() def forward(self, x): b, c, n = x.size() print("x.shape=", x.shape) y = self.avg_pool(x) print("y.shape=", y.shape) # print("channel_out", channel_out) y = self.fc1(y) y = self.relu(y) y = self.fc2(y) y = self.sigmoid(y) return x * y.expand_as(x) def get_model(input_channels=6, use_xyz=True): return Pointnet2MSG(input_channels=input_channels, use_xyz=use_xyz) class Pointnet2MSG(nn.Module): def __init__(self, input_channels=6, use_xyz=True): super(Pointnet2MSG, self).__init__() self.SA_modules = nn.ModuleList() channel_in = input_channels # print("channel_in=", channel_in) skip_channel_list = [input_channels] for k in range(cfg.RPN.SA_CONFIG.NPOINTS.__len__()): mlps = cfg.RPN.SA_CONFIG.MLPS[k].copy() channel_out = 0 # print("channel_in=", channel_in) for idx in range(mlps.__len__()): mlps[idx] = [channel_in] + mlps[idx] channel_out += mlps[idx][-1] mlps.append(channel_out) # print(mlps) print("channel_out", channel_out) self.SA_modules.append( nn.Sequential( PointnetSAModuleMSG( npoint=cfg.RPN.SA_CONFIG.NPOINTS[k], radii=cfg.RPN.SA_CONFIG.RADIUS[k], nsamples=cfg.RPN.SA_CONFIG.NSAMPLE[k], mlps=mlps[:2], use_xyz=use_xyz, bn=cfg.RPN.USE_BN, ), SelfAttention(channel_out) ) ) skip_channel_list.append(channel_out) channel_in = channel_out根据以上代码块,自注意力机制模块中forward函数所接受的x参数的值到底是什么?
根据代码块中的描述,自注意力机制模块中forward函数所接受的x参数应该是输入的特征张量,其形状为(b, c, n),其中b表示batch size,c表示通道数,n表示点云中点的数目。在代码块中,x首先通过avg_pool进行自适应平均池化操作得到y,然后经过fc1、relu、fc2和sigmoid层得到注意力权重,最后将输入特征张量x与注意力权重y相乘得到加权特征张量作为输出。
阅读全文
相关推荐
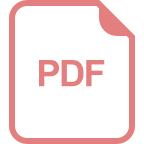
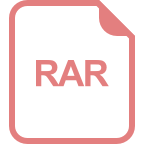
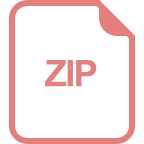








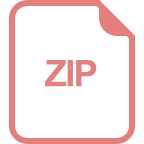
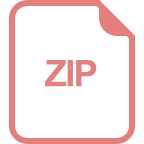
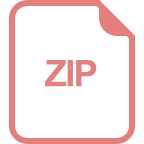
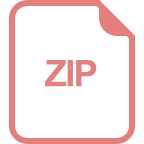
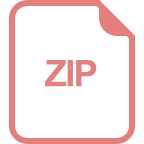