Change Detection From Synthetic Aperture Radar Images via Graph-Based Knowledge Supplement Network讲解
时间: 2024-04-06 19:35:16 浏览: 11
这是一篇关于利用合成孔径雷达图像进行变化检测的论文。在该论文中,作者提出了一种基于图形知识补充网络(Graph-Based Knowledge Supplement Network)的方法,该方法利用了合成孔径雷达(Synthetic Aperture Radar,SAR)图像的特殊性质,通过对SAR图像中的像元进行建图,从而实现对变化区域的检测。该方法利用了对SAR图像中像元之间的空间关系和相似性的建模,从而提高了变化检测的准确性和鲁棒性。论文中的方法通过在两个时间点拍摄的SAR图像之间进行对比,实现了变化检测。该方法在多个数据集上进行了测试,并取得了较好的检测效果。
相关问题
spotlight-mode synthetic aperture radar: a signal processing approach
聚焦模式合成孔径雷达(Spotlight-mode Synthetic Aperture Radar)是一种信号处理方法,用于合成孔径雷达图像的获取。
合成孔径雷达(SAR)是一种通过发射和接收多个脉冲信号来生成高分辨率雷达图像的技术。而聚焦模式是SAR中最常用的一种工作模式,它能够通过发射并记录不同距离上的脉冲信号来形成一个虚拟的大孔径天线,并利用这个大孔径天线获取高分辨率的雷达图像。
在聚焦模式中,合成孔径雷达通过发送连续的脉冲信号并接收散射回波。在接收到的回波信号中,每个脉冲的信号经过时延、多普勒频移等效应的影响,需要进行信号处理来恢复原始目标的真实信息。为了实现高分辨率图像的获取,需要对接收到的信号进行聚焦操作。
聚焦操作是通过将脉冲信号与一个特殊的函数进行卷积操作来实现的。这个特殊的函数即为合成孔径函数,它相当于虚拟的大孔径天线的响应。这样,在卷积操作后,可以得到高分辨率的图像。通过遍历不同位置的回波信号进行聚焦操作,最终可以得到整个目标区域的高分辨率图像。
聚焦模式合成孔径雷达技术在地质勘探、环境监测、灾害预警等领域具有广泛应用。它能够提供高分辨率、宽覆盖范围和强鲁棒性的雷达图像,对于获取地表特征、检测目标物体以及进行地理信息提取等方面有着重要的作用。在信号处理过程中,聚焦模式合成孔径雷达需要进行复杂的算法计算和优化,以提高图像质量和准确性。
总之,聚焦模式合成孔径雷达是一种基于信号处理的方法,通过使用虚拟的大孔径天线来获取高分辨率的雷达图像,广泛应用于多个领域。
smote和Ensemble-based methods如何结合
SMOTE 和 Ensemble-based 方法可以结合在一起来提高分类问题的性能。SMOTE (Synthetic Minority Over-sampling Technique) 是一种用于不平衡数据集上的过采样方法,它可以合成一些合理的少数类样本,从而增加少数类在训练集中的比例;而 Ensemble-based 方法则是通过组合多个基分类器的分类结果来提高分类性能。
结合起来的 SMOTE-Ensemble 方法可以通过以下步骤实现:
1. 对于不平衡数据集,首先使用 SMOTE 过采样来平衡数据集的类别分布。
2. 在过采样之后的新数据集上,使用 Ensemble-based 方法来构建多个基分类器。
3. 使用多数投票或加权投票等策略来组合基分类器的分类结果。
4. 在测试集上进行实验,评估 SMOTE-Ensemble 方法的性能。
通过这种结合方法,可以有效地提高分类问题的性能,特别是在不平衡数据集上的分类任务。
相关推荐
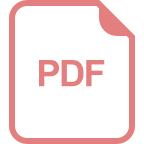
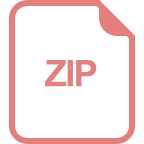
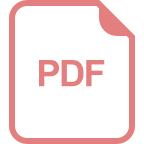












