读取Excel 文件“week2vest1.xsx”中的数据,并分别按行、按列计算平均值,并将计算得到的平均值写入文件“week2vest1.tx”中。
时间: 2023-03-20 14:02:52 浏览: 174
好的,我可以回答这个问题。您需要使用Python中的pandas库来读取Excel文件并计算平均值,然后将结果写入文本文件中。以下是可能的代码实现:
```python
import pandas as pd
# 读取Excel文件中的数据
df = pd.read_excel("week2vest1.xsx")
# 计算每行的平均值
row_means = df.mean(axis=1)
# 计算每列的平均值
col_means = df.mean(axis=0)
# 将计算得到的平均值写入文本文件中
with open("week2vest1.tx", "w") as f:
f.write("按行计算的平均值:\n")
f.write(row_means.to_string(index=False))
f.write("\n\n按列计算的平均值:\n")
f.write(col_means.to_string(index=False))
```
这个代码会在当前目录下创建一个名为“week2vest1.tx”的文本文件,其中包含按行计算的平均值和按列计算的平均值。
相关问题
excel预览前端报错XLSX.read Cannot read properties of undefined (reading 'read')
根据您提供的引用内容,报错"Cannot read properties of undefined (reading 'read')"是由于在使用XLSX.read方法时出现了错误。这个错误可能是由于在导入XLSX库时的语法问题导致的。您可以尝试解决这个问题的方法是将语法`import XLSX from 'xlsx'`改为`import * as XLSX from 'xlsx'`。这样可以正确导入XLSX库并解决报错问题。<span class="em">1</span><span class="em">2</span><span class="em">3</span>
#### 引用[.reference_title]
- *1* *2* [在使用xlsx.js导入文件报错Error: Cannot read properties of undefined (reading ‘read‘) at FileReader...](https://blog.csdn.net/weixin_47979372/article/details/123984292)[target="_blank" data-report-click={"spm":"1018.2226.3001.9630","extra":{"utm_source":"vip_chatgpt_common_search_pc_result","utm_medium":"distribute.pc_search_result.none-task-cask-2~all~insert_cask~default-1-null.142^v93^chatsearchT3_2"}}] [.reference_item style="max-width: 50%"]
- *3* [xlsx报错:Cannot read properties of undefined (reading ‘utils‘)解决方法(人力资源管理项目excel表格...](https://blog.csdn.net/Vest_er/article/details/128044692)[target="_blank" data-report-click={"spm":"1018.2226.3001.9630","extra":{"utm_source":"vip_chatgpt_common_search_pc_result","utm_medium":"distribute.pc_search_result.none-task-cask-2~all~insert_cask~default-1-null.142^v93^chatsearchT3_2"}}] [.reference_item style="max-width: 50%"]
[ .reference_list ]
阅读全文
相关推荐
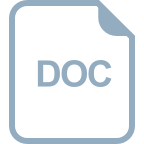
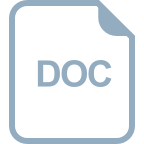
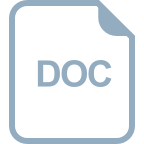
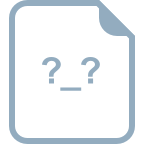
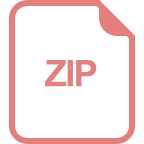
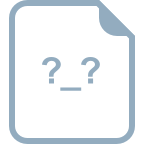
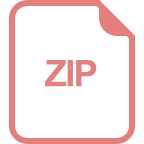
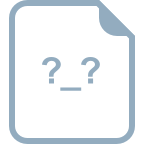
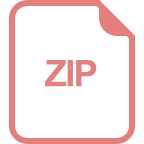
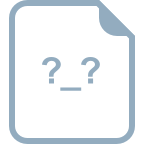
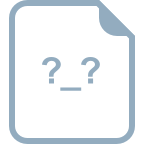
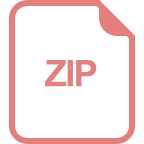
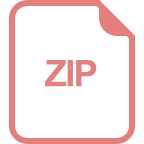
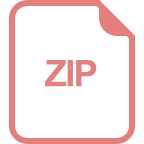
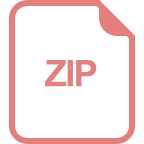
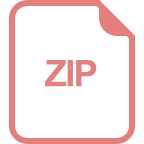
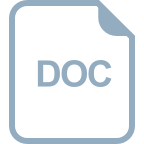
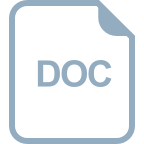