卷积神经网络实现手写数据集的识别完整代码
时间: 2023-12-04 13:05:58 浏览: 72
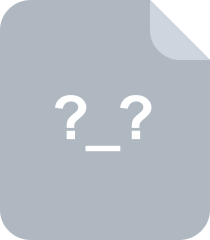
卷积神经网络实现手写数字识别代码

以下是使用TensorFlow实现手写数字识别的卷积神经网络完整代码:
```
import tensorflow as tf
from tensorflow.examples.tutorials.mnist import input_data
# Load the MNIST dataset
mnist = input_data.read_data_sets("MNIST_data/", one_hot=True)
# Define the hyperparameters
learning_rate = 0.001
training_iters = 20000
batch_size = 128
display_step = 10
# Define the network parameters
n_input = 784 # MNIST data input (img shape: 28*28)
n_classes = 10 # MNIST total classes (0-9 digits)
dropout = 0.75 # Dropout, probability to keep units
# Define the placeholders for inputs and labels
x = tf.placeholder(tf.float32, [None, n_input])
y = tf.placeholder(tf.float32, [None, n_classes])
keep_prob = tf.placeholder(tf.float32) # dropout (keep probability)
# Define the conv2d function
def conv2d(name, l_input, w, b, s):
return tf.nn.relu(tf.nn.bias_add(tf.nn.conv2d(l_input, w, strides=[1, s, s, 1], padding='SAME'), b), name=name)
# Define the maxpool2d function
def max_pool(name, l_input, k, s):
return tf.nn.max_pool(l_input, ksize=[1, k, k, 1], strides=[1, s, s, 1], padding='SAME', name=name)
# Define the normalization function
def norm(name, l_input, lsize=4):
return tf.nn.lrn(l_input, lsize, bias=1.0, alpha=0.001 / 9.0, beta=0.75, name=name)
# Define the network
def alex_net(_X, _weights, _biases, _dropout):
# Reshape input picture
_X = tf.reshape(_X, shape=[-1, 28, 28, 1])
# Convolution Layer 1
conv1 = conv2d('conv1', _X, _weights['wc1'], _biases['bc1'], 4)
# Max Pooling (down-sampling)
pool1 = max_pool('pool1', conv1, k=3, s=2)
# Apply Normalization
norm1 = norm('norm1', pool1, lsize=4)
# Apply Dropout
norm1 = tf.nn.dropout(norm1, _dropout)
# Convolution Layer 2
conv2 = conv2d('conv2', norm1, _weights['wc2'], _biases['bc2'], 1)
# Max Pooling (down-sampling)
pool2 = max_pool('pool2', conv2, k=3, s=2)
# Apply Normalization
norm2 = norm('norm2', pool2, lsize=4)
# Apply Dropout
norm2 = tf.nn.dropout(norm2, _dropout)
# Convolution Layer 3
conv3 = conv2d('conv3', norm2, _weights['wc3'], _biases['bc3'], 1)
# Max Pooling (down-sampling)
pool3 = max_pool('pool3', conv3, k=3, s=2)
# Apply Normalization
norm3 = norm('norm3', pool3, lsize=4)
# Apply Dropout
norm3 = tf.nn.dropout(norm3, _dropout)
# Fully connected layer 1
dense1 = tf.reshape(norm3, [-1, _weights['wd1'].get_shape().as_list()[0]]) # Reshape conv3 output to fit dense layer input
dense1 = tf.nn.relu(tf.matmul(dense1, _weights['wd1']) + _biases['bd1'], name='fc1') # Relu activation
# Apply Dropout
dense1 = tf.nn.dropout(dense1, _dropout)
# Fully connected layer 2
dense2 = tf.nn.relu(tf.matmul(dense1, _weights['wd2']) + _biases['bd2'], name='fc2') # Relu activation
# Apply Dropout
dense2 = tf.nn.dropout(dense2, _dropout)
# Output, class prediction
out = tf.matmul(dense2, _weights['out']) + _biases['out']
return out
# Define the weights and biases
weights = {
'wc1': tf.Variable(tf.random_normal([11, 11, 1, 96])),
'wc2': tf.Variable(tf.random_normal([5, 5, 96, 256])),
'wc3': tf.Variable(tf.random_normal([3, 3, 256, 384])),
'wd1': tf.Variable(tf.random_normal([4*4*384, 1024])),
'wd2': tf.Variable(tf.random_normal([1024, 1024])),
'out': tf.Variable(tf.random_normal([1024, n_classes]))
}
biases = {
'bc1': tf.Variable(tf.random_normal([96])),
'bc2': tf.Variable(tf.random_normal([256])),
'bc3': tf.Variable(tf.random_normal([384])),
'bd1': tf.Variable(tf.random_normal([1024])),
'bd2': tf.Variable(tf.random_normal([1024])),
'out': tf.Variable(tf.random_normal([n_classes]))
}
# Construct the model
pred = alex_net(x, weights, biases, keep_prob)
# Define the loss function and optimizer
cost = tf.reduce_mean(tf.nn.softmax_cross_entropy_with_logits(logits=pred, labels=y))
optimizer = tf.train.AdamOptimizer(learning_rate=learning_rate).minimize(cost)
# Evaluate the model
correct_pred = tf.equal(tf.argmax(pred, 1), tf.argmax(y, 1))
accuracy = tf.reduce_mean(tf.cast(correct_pred, tf.float32))
# Initialize the variables
init = tf.global_variables_initializer()
# Launch the graph
with tf.Session() as sess:
sess.run(init)
step = 1
# Keep training until reach max iterations
while step * batch_size < training_iters:
batch_x, batch_y = mnist.train.next_batch(batch_size)
# Run optimization op (backprop)
sess.run(optimizer, feed_dict={x: batch_x, y: batch_y, keep_prob: dropout})
if step % display_step == 0:
# Calculate batch loss and accuracy
loss, acc = sess.run([cost, accuracy], feed_dict={x: batch_x, y: batch_y, keep_prob: 1.})
print("Iter " + str(step*batch_size) + ", Minibatch Loss= " + "{:.6f}".format(loss) + ", Training Accuracy= " + "{:.5f}".format(acc))
step += 1
print("Optimization Finished!")
# Calculate accuracy for 256 mnist test images
print("Testing Accuracy:", sess.run(accuracy, feed_dict={x: mnist.test.images[:256], y: mnist.test.labels[:256], keep_prob: 1.}))
```
注意:这里的代码使用的是AlexNet模型,如果需要使用其他模型,需要相应地修改网络结构。
阅读全文
相关推荐
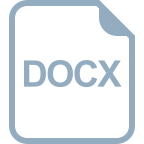
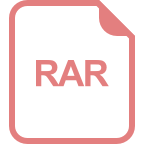
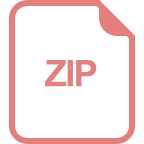
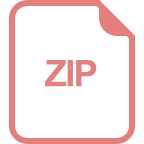


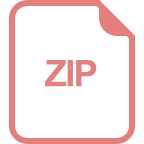
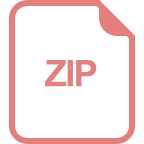
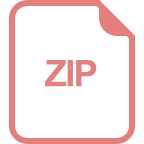
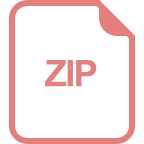
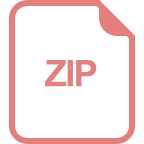
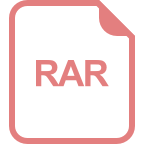
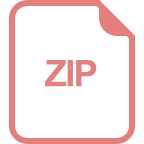
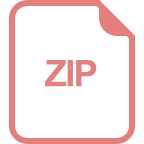
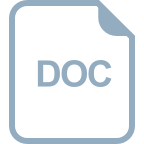
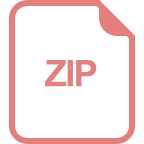