python实现ga-pso优化算法
时间: 2023-05-14 21:02:56 浏览: 423
GA-PSO优化算法是一种将遗传算法(GA)和粒子群算法(PSO)结合起来的优化算法。它的核心思想是通过模拟生物的进化过程和粒子的搜索过程来寻求全局最优解。
在Python中实现GA-PSO优化算法需要先导入相应的库和模块,如numpy、matplotlib、random等。然后需要定义适应度函数、种群数量、变异率、迭代次数等参数,以及适应度函数的计算方法、群体位置的更新方法等。其中适应度函数的定义是算法实现的关键,应该根据实际问题选择适当的形式。
在GA-PSO优化算法中,遗传算法的作用是保持全局的搜索能力和探索性,而粒子群算法的作用则是在进化进程中保持局部的搜索能力和聚集性。因此,在Python中实现GA-PSO优化算法需要仔细考虑如何将两种算法结合起来,并根据具体问题不断进行优化和改进。
总的来说,在Python中实现GA-PSO优化算法需要具备一定的编程和算法技能,需要理解并掌握GA和PSO算法的原理及其实现过程。只有这样才能在实践中高效地解决实际问题,实现高质量的优化效果。
相关问题
ga-pso的代码python
ga-pso是遗传算法和粒子群优化算法的结合,它综合了两种算法的优点,使得优化的结果更加稳定和高效。
以下是一个简单的用Python实现ga-pso的代码示例:
```python
import numpy as np
# 设置参数
population_size = 50
max_iter = 100
c1 = 2
c2 = 2
w = 0.5
max_velocity = 0.2
# 初始化种群
def initialize_population(population_size):
population = []
for _ in range(population_size):
position = np.random.uniform(-5, 5, 2)
velocity = np.zeros(2)
pbest_position = position
pbest_value = fitness_function(position)
particle = {'position': position, 'velocity': velocity, 'pbest_position': pbest_position, 'pbest_value': pbest_value}
population.append(particle)
return population
# 适应度函数
def fitness_function(x):
return np.sum(x**2)
# 更新粒子速度和位置
def update_particle(particle, gbest_position):
new_velocity = w * particle['velocity'] + c1 * np.random.rand() * (particle['pbest_position'] - particle['position']) + c2 * np.random.rand() * (gbest_position - particle['position'])
new_velocity = np.clip(new_velocity, -max_velocity, max_velocity)
new_position = particle['position'] + new_velocity
new_value = fitness_function(new_position)
if new_value < particle['pbest_value']:
particle['pbest_position'] = new_position
particle['pbest_value'] = new_value
particle['position'] = new_position
particle['velocity'] = new_velocity
# 主函数
def ga_pso(population_size, max_iter):
population = initialize_population(population_size)
gbest_position = population[0]['position']
gbest_value = fitness_function(gbest_position)
for _ in range(max_iter):
for particle in population:
if particle['pbest_value'] < gbest_value:
gbest_position = particle['pbest_position']
gbest_value = particle['pbest_value']
update_particle(particle, gbest_position)
return gbest_position, gbest_value
# 运行算法
best_position, best_value = ga_pso(population_size, max_iter)
print('最优解:', best_position)
print('最优值:', best_value)
```
这是一个简单的用Python实现的ga-pso算法示例。在这个示例中,我们设置了种群大小为50,最大迭代次数为100,粒子的速度和位置更新规则使用了惯性权重w、加速系数c1和c2等参数。通过运行算法,我们可以得到一个优化问题的最优解和最优值。这个示例可以作为ga-pso算法的起点,根据具体的优化问题进行调整和改进。
GA-PSO python代码
下面是一个简单的 GA-PSO 算法的 Python 代码示例:
```python
import numpy as np
import random
class Particle:
def __init__(self, dim, pos_range, vel_range):
self.dim = dim
self.pos = np.random.uniform(*pos_range, size=dim)
self.vel = np.random.uniform(*vel_range, size=dim)
self.best_pos = self.pos.copy()
self.fitness = 0
self.best_fitness = 0
def update_position(self):
self.pos += self.vel
def update_velocity(self, omega, c1, c2, gbest_pos):
r1 = np.random.uniform(size=self.dim)
r2 = np.random.uniform(size=self.dim)
social = c1 * r1 * (gbest_pos - self.pos)
cognitive = c2 * r2 * (self.best_pos - self.pos)
self.vel = omega * self.vel + social + cognitive
class PSO:
def __init__(self, num_particles, dim, pos_range, vel_range, c1, c2, omega):
self.num_particles = num_particles
self.dim = dim
self.pos_range = pos_range
self.vel_range = vel_range
self.c1 = c1
self.c2 = c2
self.omega = omega
self.particles = [Particle(dim, pos_range, vel_range) for i in range(num_particles)]
self.gbest_pos = np.zeros(dim)
self.gbest_fitness = 0
def evaluate_fitness(self, fitness_func):
for particle in self.particles:
particle.fitness = fitness_func(particle.pos)
if particle.fitness > particle.best_fitness:
particle.best_pos = particle.pos.copy()
particle.best_fitness = particle.fitness
if particle.fitness > self.gbest_fitness:
self.gbest_pos = particle.pos.copy()
self.gbest_fitness = particle.fitness
def update_particles(self):
for particle in self.particles:
particle.update_velocity(self.omega, self.c1, self.c2, self.gbest_pos)
particle.update_position()
class GA:
def __init__(self, num_individuals, dim, bounds, mutation_rate, fitness_func):
self.num_individuals = num_individuals
self.dim = dim
self.bounds = bounds
self.mutation_rate = mutation_rate
self.fitness_func = fitness_func
self.individuals = []
for i in range(num_individuals):
individual = np.random.uniform(*bounds, size=dim)
self.individuals.append(individual)
def evaluate_fitness(self):
fitness = []
for individual in self.individuals:
fitness.append(self.fitness_func(individual))
return fitness
def selection(self, fitness):
total_fitness = sum(fitness)
probabilities = [f / total_fitness for f in fitness]
indices = list(range(self.num_individuals))
selected_indices = random.choices(indices, weights=probabilities, k=self.num_individuals)
selected_individuals = [self.individuals[i] for i in selected_indices]
return selected_individuals
def crossover(self, parents):
children = []
for i in range(0, self.num_individuals, 2):
parent1, parent2 = parents[i], parents[i+1]
split_index = random.randint(1, self.dim - 1)
child1 = np.concatenate((parent1[:split_index], parent2[split_index:]))
child2 = np.concatenate((parent2[:split_index], parent1[split_index:]))
children.append(child1)
children.append(child2)
return children
def mutation(self, children):
for i in range(self.num_individuals):
if random.random() < self.mutation_rate:
children[i] = np.random.uniform(*self.bounds, size=self.dim)
return children
class GAPSO:
def __init__(self, num_particles, dim, pos_range, vel_range, c1, c2, omega,
num_individuals, bounds, mutation_rate, fitness_func):
self.num_particles = num_particles
self.dim = dim
self.pos_range = pos_range
self.vel_range = vel_range
self.c1 = c1
self.c2 = c2
self.omega = omega
self.num_individuals = num_individuals
self.bounds = bounds
self.mutation_rate = mutation_rate
self.fitness_func = fitness_func
self.pso = PSO(num_particles, dim, pos_range, vel_range, c1, c2, omega)
self.ga = GA(num_individuals, dim, bounds, mutation_rate, fitness_func)
def run(self, num_iterations):
for i in range(num_iterations):
self.pso.evaluate_fitness(self.fitness_func)
fitness = self.ga.evaluate_fitness()
parents = self.ga.selection(fitness)
children = self.ga.crossover(parents)
children = self.ga.mutation(children)
self.ga.individuals = self.pso.particles + children
self.pso.num_particles = len(self.ga.individuals)
self.pso.particles = self.ga.individuals
self.pso.update_particles()
return self.pso.gbest_pos
```
在上面的代码中,`Particle` 类表示一个粒子,包含位置、速度、适应度、最佳位置和最佳适应度等属性,以及更新位置和速度的方法。`PSO` 类表示一个 PSO 算法,包含多个粒子,以及初始化、评估适应度和更新粒子的方法。`GA` 类表示一个简单的遗传算法,包含多个个体,以及评估适应度、选择、交叉和变异的方法。`GAPSO` 类表示一个 GA-PSO 算法,包含一个 PSO 和一个 GA,以及运行算法的方法。
相关推荐
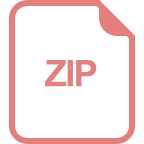
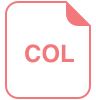
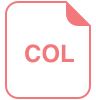










