解释这行代码self.gate = nn.Linear(input_size+hidden_size, cell_size)
时间: 2024-06-02 21:12:17 浏览: 118
这行代码是在定义一个门控循环单元(GRU)的模型中使用的。其中,`nn.Linear`是一个PyTorch中的线性层,用于将输入向量进行线性变换。在这里,`input_size+hidden_size`表示输入向量和上一个时刻的隐藏状态向量的长度之和,`cell_size`则表示门控循环单元中的状态向量的长度。因此,`self.gate`是一个将输入向量和隐藏状态向量拼接后进行线性变换得到状态向量的层。
相关问题
mogrifier lstm的python代码
以下是一个使用Mogrifier LSTM的简单示例代码:
```python
import torch
import torch.nn as nn
class MogrifierLSTM(nn.Module):
def __init__(self, input_size, hidden_size, num_layers):
super(MogrifierLSTM, self).__init__()
self.hidden_size = hidden_size
self.num_layers = num_layers
self.input_gate = nn.Linear(input_size, hidden_size)
self.forget_gate = nn.Linear(input_size, hidden_size)
self.output_gate = nn.Linear(input_size, hidden_size)
self.cell_gate = nn.Linear(input_size, hidden_size)
self.mogrify_input = nn.Linear(hidden_size, hidden_size)
self.mogrify_forget = nn.Linear(hidden_size, hidden_size)
self.mogrify_output = nn.Linear(hidden_size, hidden_size)
self.mogrify_cell = nn.Linear(hidden_size, hidden_size)
def forward(self, input):
batch_size = input.size(0)
hidden = (torch.zeros(self.num_layers, batch_size, self.hidden_size),
torch.zeros(self.num_layers, batch_size, self.hidden_size))
for i in range(self.num_layers):
hx, cx = hidden
ix = self.input_gate(input) + self.mogrify_input(cx[i])
fx = self.forget_gate(input) + self.mogrify_forget(cx[i])
ox = self.output_gate(input) + self.mogrify_output(cx[i])
gx = self.cell_gate(input) + self.mogrify_cell(cx[i])
input_gate = torch.sigmoid(ix)
forget_gate = torch.sigmoid(fx)
output_gate = torch.sigmoid(ox)
cell_gate = torch.tanh(gx)
cy = (forget_gate * cx[i]) + (input_gate * cell_gate)
hy = output_gate * torch.tanh(cy)
input = hy
hidden = (hy.unsqueeze(0), cy.unsqueeze(0))
return hidden
# 使用示例
input_size = 10
hidden_size = 20
num_layers = 2
seq_length = 5
batch_size = 3
# 创建Mogrifier LSTM模型
model = MogrifierLSTM(input_size, hidden_size, num_layers)
# 创建随机输入张量
input = torch.randn(seq_length, batch_size, input_size)
# 前向传播
output = model(input)
print(output)
```
请注意,这只是一个简单的示例代码,实际应用中可能需要进行更多的调整和优化。
为我绘制LSTM+PyTorch文本分类的神经网络结构图,尽可能详细一点细节一点
在PyTorch中,使用LSTM(长短时记忆网络)进行文本分类通常涉及以下步骤和神经网络结构:
1. **数据预处理**:
- 文本被编码成整数序列(例如使用Tokenizer,如`torchtext.data.Field`)
- 序列填充到固定长度,以便所有样本输入有相同的维度
2. **嵌入层(Embedding Layer)**:
- 创建一个词嵌入矩阵,将每个整数索引映射到一个低维向量空间。这可以是预训练的Word2Vec、GloVe或随机初始化。
3. **长短期记忆层(LSTM Layer)**:
- LSTM层用于捕捉文本中的长期依赖性。它包含两个门控单元:输入门(input gate)、遗忘门(forget gate)、输出门(output gate)以及细胞状态(cell state)。每一步都接收上一时刻的隐藏状态和当前输入,并更新内部状态。
4. **全连接层(Linear Layer)**:
- 经过LSTM层后,最后的时间步的隐藏状态会被送入一个全连接层,将其转化为文本分类所需的类别得分。
5. **dropout层(Dropout Layer)**:
- 可能会在这几个层之间添加dropout层,防止过拟合,减少模型对某些特征的过度依赖。
6. **激活函数(Activation Function)**:
- 最后的线性层通常使用softmax激活,因为它会产生每个类别的概率分布。
7. **损失函数(Loss Function)**:
- 对于二分类任务,可能是交叉熵损失;对于多分类任务,就是多类 softmax 损失。
8. **优化器(Optimizer)**:
- PyTorch中常用的有Adam、SGD等,设置合适的学习率和迭代次数。
9. **前向传播和反向传播(Forward and Backward Passes)**:
- 训练过程中,计算预测值与真实标签之间的差异,然后通过反向传播更新权重。
下面是一个简化的伪代码示例:
```python
import torch.nn as nn
import torch.nn.functional as F
class TextClassifier(nn.Module):
def __init__(self, vocab_size, embed_dim, hidden_dim, num_classes, dropout=0.5):
super().__init__()
self.embedding = nn.Embedding(vocab_size, embed_dim)
self.lstm = nn.LSTM(embed_dim, hidden_dim, bidirectional=True)
self.dropout = nn.Dropout(dropout)
self.fc = nn.Linear(2*hidden_dim, num_classes) # 因为是双向LSTM,乘以2
def forward(self, input_ids, lengths):
embedded = self.embedding(input_ids)
packed_embedded = nn.utils.rnn.pack_padded_sequence(embedded, lengths)
lstm_out, _ = self.lstm(packed_embedded)
lstm_out, _ = nn.utils.rnn.pad_packed_sequence(lstm_out) # 解包并合并方向
lstm_out = self.dropout(lstm_out[:, -1, :]) # 取最后一个时间步
output = self.fc(lstm_out)
return F.softmax(output, dim=1)
# 使用模型
model = TextClassifier(...).to(device)
optimizer = Adam(model.parameters(), lr=0.001)
criterion = nn.CrossEntropyLoss()
for inputs, labels in dataloader:
inputs, labels = inputs.to(device), labels.to(device)
outputs = model(inputs, lengths)
loss = criterion(outputs, labels)
optimizer.zero_grad()
loss.backward()
optimizer.step()
```
阅读全文
相关推荐
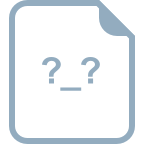
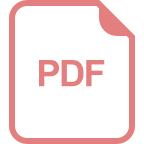
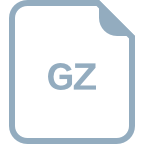
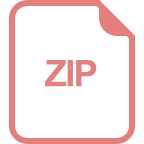
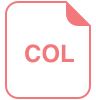
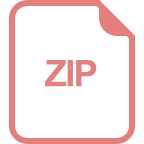