双向A星算法Python实现
时间: 2023-05-18 09:04:11 浏览: 181
以下是双向A星算法的Python实现:
```python
import heapq
def astar(start, goal, neighbors_fn, heuristic_fn):
"""
A* algorithm implementation.
:param start: starting node
:param goal: goal node
:param neighbors_fn: function that returns the neighbors of a node
:param heuristic_fn: function that returns the heuristic value of a node
:return: path from start to goal
"""
# Initialize the open and closed sets
open_set = []
closed_set = set()
# Initialize the start and goal nodes
start_node = Node(start, None, 0, heuristic_fn(start, goal))
goal_node = Node(goal, None, 0, 0)
# Add the start node to the open set
heapq.heappush(open_set, start_node)
# Loop until the open set is empty
while open_set:
# Get the node with the lowest f value
current_node = heapq.heappop(open_set)
# Check if we have reached the goal
if current_node == goal_node:
path = []
while current_node:
path.append(current_node.state)
current_node = current_node.parent
return path[::-1]
# Add the current node to the closed set
closed_set.add(current_node)
# Get the neighbors of the current node
for neighbor in neighbors_fn(current_node.state):
# Create a new node for the neighbor
neighbor_node = Node(neighbor, current_node, current_node.g + 1, heuristic_fn(neighbor, goal))
# Check if the neighbor is already in the closed set
if neighbor_node in closed_set:
continue
# Check if the neighbor is already in the open set
if neighbor_node in open_set:
# Update the neighbor's g value if we found a better path
open_neighbor = open_set[open_set.index(neighbor_node)]
if neighbor_node.g < open_neighbor.g:
open_neighbor.g = neighbor_node.g
open_neighbor.parent = neighbor_node.parent
else:
# Add the neighbor to the open set
heapq.heappush(open_set, neighbor_node)
# If we reach this point, there is no path from start to goal
return None
class Node:
"""
A node in the search tree.
"""
def __init__(self, state, parent, g, h):
self.state = state
self.parent = parent
self.g = g
self.h = h
def __lt__(self, other):
return (self.g + self.h) < (other.g + other.h)
def __eq__(self, other):
return self.state == other.state
def bidirectional_astar(start, goal, neighbors_fn, heuristic_fn):
"""
Bidirectional A* algorithm implementation.
:param start: starting node
:param goal: goal node
:param neighbors_fn: function that returns the neighbors of a node
:param heuristic_fn: function that returns the heuristic value of a node
:return: path from start to goal
"""
# Initialize the open and closed sets for both directions
open_set_start = []
closed_set_start = set()
open_set_goal = []
closed_set_goal = set()
# Initialize the start and goal nodes for both directions
start_node_start = Node(start, None, 0, heuristic_fn(start, goal))
start_node_goal = Node(goal, None, 0, heuristic_fn(goal, start))
goal_node_start = Node(goal, None, 0, 0)
goal_node_goal = Node(start, None, 0, 0)
# Add the start and goal nodes to the open sets for both directions
heapq.heappush(open_set_start, start_node_start)
heapq.heappush(open_set_goal, start_node_goal)
# Loop until one of the open sets is empty
while open_set_start and open_set_goal:
# Get the node with the lowest f value from both directions
current_node_start = heapq.heappop(open_set_start)
current_node_goal = heapq.heappop(open_set_goal)
# Check if we have reached the goal from both directions
if current_node_start in closed_set_goal or current_node_goal in closed_set_start:
path = []
# Add the path from start to current_node_start
while current_node_start:
path.append(current_node_start.state)
current_node_start = current_node_start.parent
# Add the path from goal to current_node_goal
while current_node_goal:
path.append(current_node_goal.state)
current_node_goal = current_node_goal.parent
return path[::-1]
# Add the current nodes to the closed sets for both directions
closed_set_start.add(current_node_start)
closed_set_goal.add(current_node_goal)
# Get the neighbors of the current nodes for both directions
for neighbor_start in neighbors_fn(current_node_start.state):
# Create a new node for the neighbor from the start direction
neighbor_node_start = Node(neighbor_start, current_node_start, current_node_start.g + 1, heuristic_fn(neighbor_start, goal))
# Check if the neighbor from the start direction is already in the closed set from the goal direction
if neighbor_node_start in closed_set_goal:
continue
# Check if the neighbor from the start direction is already in the open set from the start direction
if neighbor_node_start in open_set_start:
# Update the neighbor's g value if we found a better path
open_neighbor_start = open_set_start[open_set_start.index(neighbor_node_start)]
if neighbor_node_start.g < open_neighbor_start.g:
open_neighbor_start.g = neighbor_node_start.g
open_neighbor_start.parent = neighbor_node_start.parent
else:
# Add the neighbor from the start direction to the open set from the start direction
heapq.heappush(open_set_start, neighbor_node_start)
for neighbor_goal in neighbors_fn(current_node_goal.state):
# Create a new node for the neighbor from the goal direction
neighbor_node_goal = Node(neighbor_goal, current_node_goal, current_node_goal.g + 1, heuristic_fn(neighbor_goal, start))
# Check if the neighbor from the goal direction is already in the closed set from the start direction
if neighbor_node_goal in closed_set_start:
continue
# Check if the neighbor from the goal direction is already in the open set from the goal direction
if neighbor_node_goal in open_set_goal:
# Update the neighbor's g value if we found a better path
open_neighbor_goal = open_set_goal[open_set_goal.index(neighbor_node_goal)]
if neighbor_node_goal.g < open_neighbor_goal.g:
open_neighbor_goal.g = neighbor_node_goal.g
open_neighbor_goal.parent = neighbor_node_goal.parent
else:
# Add the neighbor from the goal direction to the open set from the goal direction
heapq.heappush(open_set_goal, neighbor_node_goal)
# If we reach this point, there is no path from start to goal
return None
```
希望这个实现对你有所帮助!
相关推荐
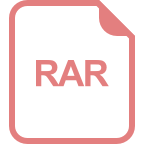














