from sklearn.metrics import classification_report, confusion_matrix y_pred =knn_model.predict(X_test) print(classification_report(y_test,y_pred))分别解释各行代码
时间: 2023-12-04 15:03:19 浏览: 75
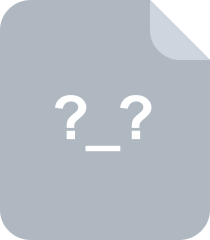
knn分类模型,代码解析
这段代码主要是使用 scikit-learn 库中的 classification_report 和 confusion_matrix 函数,对训练好的 k 近邻分类器模型在测试数据上的性能进行评估,并输出评估结果。
- `from sklearn.metrics import classification_report, confusion_matrix`:导入 scikit-learn 库中的 classification_report 和 confusion_matrix 函数,用于评估分类器模型的性能。
- `y_pred = knn_model.predict(X_test)`:使用训练好的 k 近邻分类器模型对测试数据 X_test 做出预测,将预测结果保存在 y_pred 变量中。
- `print(classification_report(y_test, y_pred))`:使用 classification_report 函数对分类器模型在测试数据上的性能进行评估,并将评估结果打印输出。classification_report 函数会输出每个类别的精确率、召回率、F1 值以及支持样本数等指标,用于评估分类器模型的性能。其中,y_test 是测试数据的真实标签,y_pred 是分类器模型预测的标签。
阅读全文
相关推荐
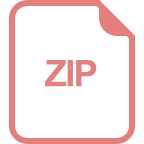




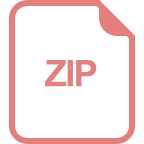
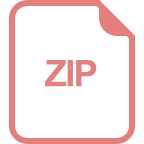
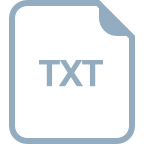
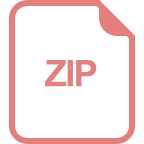
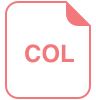
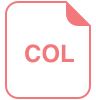
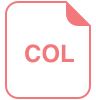




