使用 Python 和 torch 库实现逻辑回归
时间: 2024-04-10 18:34:56 浏览: 96
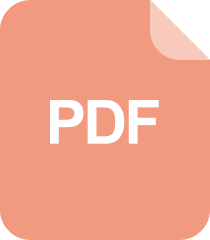
PyTorch线性回归和逻辑回归实战示例
要使用 Python 和 torch 库实现逻辑回归,你可以按照以下步骤进行操作:
1. 导入所需的库:
```python
import torch
import torch.nn as nn
import torch.optim as optim
```
2. 准备数据:
```python
# 假设你有输入特征 x 和对应的标签 y
x = torch.tensor([[1.0], [2.0], [3.0], [4.0]]) # 输入特征
y = torch.tensor([[0], [0], [1], [1]]) # 对应的标签
```
3. 定义逻辑回归模型:
```python
class LogisticRegression(nn.Module):
def __init__(self):
super(LogisticRegression, self).__init__()
self.linear = nn.Linear(1, 1) # 输入维度为1,输出维度为1
def forward(self, x):
out = torch.sigmoid(self.linear(x))
return out
model = LogisticRegression()
```
4. 定义损失函数和优化器:
```python
criterion = nn.BCELoss() # 二分类交叉熵损失函数
optimizer = optim.SGD(model.parameters(), lr=0.01) # 使用随机梯度下降优化器,学习率为0.01
```
5. 训练模型:
```python
num_epochs = 1000 # 迭代次数
for epoch in range(num_epochs):
# 前向传播
outputs = model(x)
loss = criterion(outputs, y)
# 反向传播和优化
optimizer.zero_grad()
loss.backward()
optimizer.step()
if (epoch+1) % 100 == 0:
print('Epoch [{}/{}], Loss: {:.4f}'.format(epoch+1, num_epochs, loss.item()))
```
6. 使用模型进行预测:
```python
model.eval() # 设置模型为评估模式
with torch.no_grad():
predicted = model(x)
predicted = predicted.round() # 四舍五入取整
print('Predicted:', predicted)
```
这样就完成了使用 Python 和 torch 库实现逻辑回归的过程。请注意,这只是一个简单的示例,实际应用中可能需要根据具体情况进行调整和扩展。
阅读全文
相关推荐
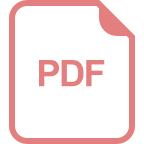
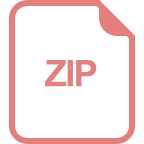
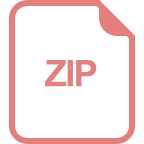
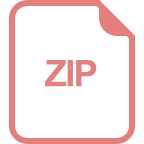
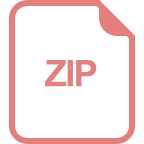
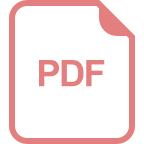
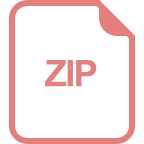
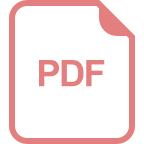
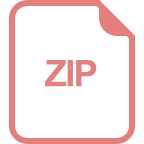