python遗传算法代码
时间: 2023-09-01 20:12:37 浏览: 92
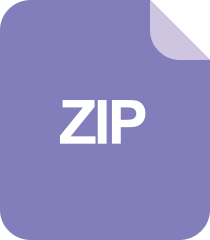
GeneticAlgorithmsWithPython-master_geneticalgorithm_遗传算法书中代码_
以下是一个简单的Python遗传算法代码示例:
```
import random
# 定义目标函数,这里为了演示,使用一个简单的函数
def fitness_function(x):
return x**2
# 生成初始种群
def generate_initial_population(population_size, chromosome_length):
population = []
for i in range(population_size):
chromosome = []
for j in range(chromosome_length):
chromosome.append(random.randint(0, 1))
population.append(chromosome)
return population
# 计算适应度值
def calculate_fitness(population):
fitness_values = []
for chromosome in population:
x = decode_chromosome(chromosome)
fitness_values.append(fitness_function(x))
return fitness_values
# 解码染色体
def decode_chromosome(chromosome):
x = 0
for i in range(len(chromosome)):
x += chromosome[i] * 2**i
return x
# 选择适应度高的个体
def selection(population, fitness_values):
selected_population = []
for i in range(len(population)):
selected_population.append(random.choices(population, weights=fitness_values)[0])
return selected_population
# 交叉操作
def crossover(parent1, parent2, crossover_rate):
if random.random() < crossover_rate:
crossover_point = random.randint(0, len(parent1)-1)
child1 = parent1[:crossover_point] + parent2[crossover_point:]
child2 = parent2[:crossover_point] + parent1[crossover_point:]
return child1, child2
else:
return parent1, parent2
# 变异操作
def mutation(chromosome, mutation_rate):
mutated_chromosome = []
for gene in chromosome:
if random.random() < mutation_rate:
mutated_chromosome.append(1 - gene)
else:
mutated_chromosome.append(gene)
return mutated_chromosome
# 主程序
population_size = 10
chromosome_length = 5
crossover_rate = 0.8
mutation_rate = 0.01
generations = 10
population = generate_initial_population(population_size, chromosome_length)
for i in range(generations):
fitness_values = calculate_fitness(population)
selected_population = selection(population, fitness_values)
new_population = []
for j in range(int(population_size/2)):
parent1 = selected_population[j*2]
parent2 = selected_population[j*2+1]
child1, child2 = crossover(parent1, parent2, crossover_rate)
child1 = mutation(child1, mutation_rate)
child2 = mutation(child2, mutation_rate)
new_population.append(child1)
new_population.append(child2)
population = new_population
# 输出最终结果
best_chromosome = max(population, key=lambda c: fitness_function(decode_chromosome(c)))
best_x = decode_chromosome(best_chromosome)
print("The best solution is x={}, f(x)={}".format(best_x, fitness_function(best_x)))
```
这个遗传算法代码实现的是一个求解 $f(x) = x^2$ 的最大值的问题。其中,generate_initial_population() 函数用来生成初始种群,calculate_fitness() 函数用来计算适应度值,selection() 函数用来进行选择操作,crossover() 函数用来进行交叉操作,mutation() 函数用来进行变异操作。最终,程序输出最优解。
阅读全文
相关推荐
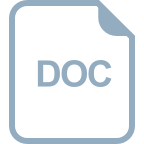
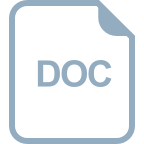

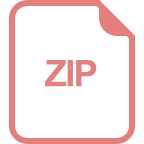
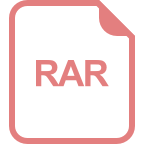
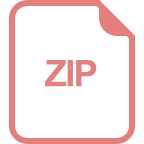
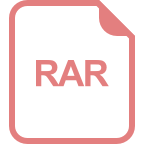
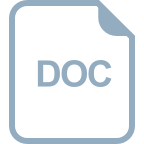
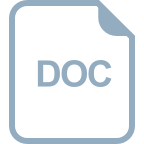






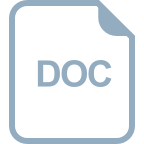
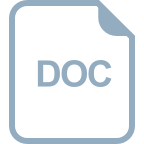
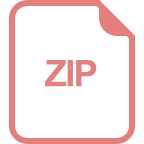