# Matrix operations --------------------------- A <- matrix(1:4,2,2) B <- matrix(2:5,2,2) A * B A %*% B solve(A) t(A) eA = eigen(A) eA$val eA$vec
时间: 2023-08-15 11:53:25 浏览: 53
这段代码介绍了 R 语言中的矩阵运算。
在 R 语言中,可以使用 * 运算符来进行矩阵的逐元素相乘。例如,在代码中,定义了两个 2 行 2 列的矩阵 A 和 B,使用 A * B 可以得到一个新的矩阵,它的每个元素都是 A 和 B 对应位置元素的乘积。
另外,可以使用 %*% 运算符来进行矩阵的矩阵乘法。例如,在代码中,使用 A %*% B 可以得到一个新的矩阵,它的每个元素都是 A 和 B 进行矩阵乘法的结果。
可以使用 solve() 函数来求解矩阵的逆矩阵。例如,在代码中,使用 solve(A) 可以得到矩阵 A 的逆矩阵。
可以使用 t() 函数来对矩阵进行转置操作。例如,在代码中,使用 t(A) 可以得到矩阵 A 的转置矩阵。
可以使用 eigen() 函数来计算矩阵的特征值和特征向量。例如,在代码中,使用 eigen(A) 可以得到矩阵 A 的特征值和特征向量,其中 eA$val 表示矩阵 A 的特征值,eA$vec 表示矩阵 A 的特征向量。
相关问题
'' Basic Operations example using TensorFlow library. Author: Aymeric Damien Project: https://github.com/aymericdamien/TensorFlow-Examples/ ''' from __future__ import print_function import tensorflow as tf # Basic constant operations # The value returned by the constructor represents the output # of the Constant op. a = tf.constant(2) b = tf.constant(3) # Launch the default graph. with tf.compat.v1.Session() as sess: print("a=2, b=3") print("Addition with constants: %i" % sess.run(a+b)) print("Multiplication with constants: %i" % sess.run(a*b)) # Basic Operations with variable as graph input # The value returned by the constructor represents the output # of the Variable op. (define as input when running session) # tf Graph input a = tf.placeholder(tf.int16) b = tf.placeholder(tf.int16) # Define some operations add = tf.add(a, b) mul = tf.multiply(a, b) # Launch the default graph. with tf.compat.v1.Session() as sess: # Run every operation with variable input print("Addition with variables: %i" % sess.run(add, feed_dict={a: 2, b: 3})) print("Multiplication with variables: %i" % sess.run(mul, feed_dict={a: 2, b: 3})) # ---------------- # More in details: # Matrix Multiplication from TensorFlow official tutorial # Create a Constant op that produces a 1x2 matrix. The op is # added as a node to the default graph. # # The value returned by the constructor represents the output # of the Constant op. matrix1 = tf.constant([[3., 3.]]) # Create another Constant that produces a 2x1 matrix. matrix2 = tf.constant([[2.],[2.]]) # Create a Matmul op that takes 'matrix1' and 'matrix2' as inputs. # The returned value, 'product', represents the result of the matrix # multiplication. product = tf.matmul(matrix1, matrix2) # To run the matmul op we call the session 'run()' method, passing 'product' # which represents the output of the matmul op. This indicates to the call # that we want to get the output of the matmul op back. # # All inputs needed by the op are run automatically by the session. They # typically are run in parallel. # # The call 'run(product)' thus causes the execution of threes ops in the # graph: the two constants and matmul. # # The output of the op is returned in 'result' as a numpy `ndarray` object. with tf.compat.v1.ession() as sess: result = sess.run(product) print(result) # ==> [[ 12.]]
这是一个使用 TensorFlow 库进行基本操作的例子。首先定义了两个常量 a 和 b,并使用 Session 运行了加法和乘法操作。然后定义了两个占位符 a 和 b,并定义了加法和乘法操作。最后,使用 Session 运行了矩阵乘法操作,并打印出结果。需要注意的是,使用 TensorFlow 2.0 及以上版本时,Session 已经被删除,需要使用 Eager Execution 模式代替。
代码怎么样'' Basic Operations example using TensorFlow library. Author: Aymeric Damien Project: https://github.com/aymericdamien/TensorFlow-Examples/ ''' from __future__ import print_function import tensorflow as tf # Basic constant operations # The value returned by the constructor represents the output # of the Constant op. a = tf.constant(2) b = tf.constant(3) # Launch the default graph. with tf.compat.v1.Session() as sess: print("a=2, b=3") print("Addition with constants: %i" % sess.run(a+b)) print("Multiplication with constants: %i" % sess.run(a*b)) # Basic Operations with variable as graph input # The value returned by the constructor represents the output # of the Variable op. (define as input when running session) # tf Graph input a = tf.placeholder(tf.int16) b = tf.placeholder(tf.int16) # Define some operations add = tf.add(a, b) mul = tf.multiply(a, b) # Launch the default graph. with tf.compat.v1.Session() as sess: # Run every operation with variable input print("Addition with variables: %i" % sess.run(add, feed_dict={a: 2, b: 3})) print("Multiplication with variables: %i" % sess.run(mul, feed_dict={a: 2, b: 3})) # ---------------- # More in details: # Matrix Multiplication from TensorFlow official tutorial # Create a Constant op that produces a 1x2 matrix. The op is # added as a node to the default graph. # # The value returned by the constructor represents the output # of the Constant op. matrix1 = tf.constant([[3., 3.]]) # Create another Constant that produces a 2x1 matrix. matrix2 = tf.constant([[2.],[2.]]) # Create a Matmul op that takes 'matrix1' and 'matrix2' as inputs. # The returned value, 'product', represents the result of the matrix # multiplication. product = tf.matmul(matrix1, matrix2) # To run the matmul op we call the session 'run()' method, passing 'product' # which represents the output of the matmul op. This indicates to the call # that we want to get the output of the matmul op back. # # All inputs needed by the op are run automatically by the session. They # typically are run in parallel. # # The call 'run(product)' thus causes the execution of threes ops in the # graph: the two constants and matmul. # # The output of the op is returned in 'result' as a numpy `ndarray` object. with tf.compat.v1.ession() as sess: result = sess.run(product) print(result) # ==> [[ 12.]]
这是一个使用 TensorFlow 库进行基本操作的代码示例,其中包含两个部分。第一部分是使用常量进行的加法和乘法运算,第二部分是使用变量作为图形输入进行的加法和乘法运算。第三部分是一个来自 TensorFlow 官方教程的矩阵乘法示例。这些代码演示了 TensorFlow 的基本操作,如常量和变量的定义以及在会话中运行操作的方法。
阅读全文
相关推荐
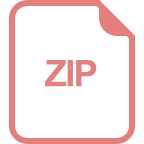
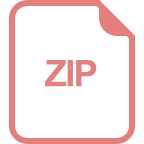
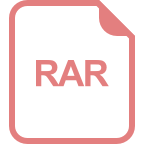
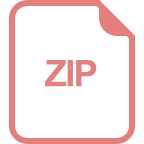
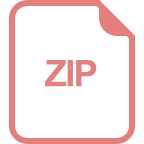
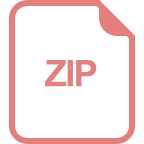
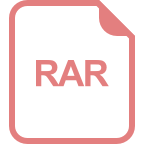
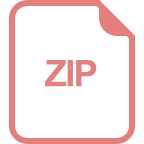
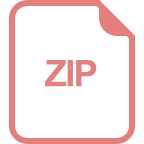
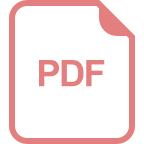
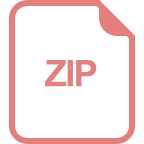
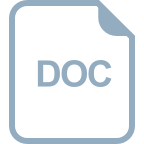

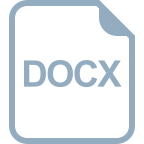