给我写一段目标检测的代码
时间: 2023-09-06 16:05:10 浏览: 91
### 回答1:
这是一段基于 Python 的目标检测代码:import cv2
import numpy as np # 读取图片文件
image = cv2.imread('image.jpg') # 转换为灰度图
gray = cv2.cvtColor(image, cv2.COLOR_BGR2GRAY) # 使用高斯滤波
blur = cv2.GaussianBlur(gray, (5, 5), 0) # 找出边缘
edged = cv2.Canny(blur, 30, 150) # 找出轮廓
contours, _ = cv2.findContours(edged, cv2.RETR_EXTERNAL, cv2.CHAIN_APPROX_NONE) # 遍历轮廓
for contour in contours:
# 获取轮廓的外接矩形
(x, y, w, h) = cv2.boundingRect(contour) # 画出外接矩形
cv2.rectangle(image, (x, y), (x+w, y+h), (0, 255, 0), 2) # 显示图片
cv2.imshow('Output', image)
cv2.waitKey(0)
### 回答2:
目标检测是计算机视觉中常见的任务之一,主要是识别和定位图像中的目标物体。以下是一段简单的目标检测代码示例:
```python
import cv2
# 加载训练好的目标检测模型
model = cv2.dnn.readNetFromTensorflow('frozen_inference_graph.pb', 'graph.pbtxt')
# 读取待检测的图像
image = cv2.imread('test_image.jpg')
# 执行目标检测
blob = cv2.dnn.blobFromImage(image, size=(300, 300), swapRB=True, crop=False)
model.setInput(blob)
output = model.forward()
# 解析检测结果
for detection in output[0, 0, :, :]:
score = float(detection[2])
if score > 0.5:
left = int(detection[3] * image.shape[1])
top = int(detection[4] * image.shape[0])
right = int(detection[5] * image.shape[1])
bottom = int(detection[6] * image.shape[0])
# 绘制检测框
cv2.rectangle(image, (left, top), (right, bottom), (0, 255, 0), 2)
# 显示检测结果
cv2.imshow('Object Detection', image)
cv2.waitKey(0)
cv2.destroyAllWindows()
```
上述代码中,首先加载了预训练的目标检测模型,模型文件为'frozen_inference_graph.pb',模型配置文件为'graph.pbtxt'。然后读取待检测的图像,通过模型进行目标检测。检测结果存储在output中,然后遍历每个检测到的目标,筛选出置信度大于0.5的目标,并获取其位置信息。最后,在原图像上绘制检测框并显示结果。
### 回答3:
目标检测是计算机视觉领域的一个重要任务,主要用于在图像或视频中识别和定位不同的目标物体。以下是一段基于深度学习模型的目标检测代码示例:
```python
# 导入必要的库
import cv2
import numpy as np
# 载入已经训练好的目标检测模型
net = cv2.dnn.readNetFromDarknet('yolo.cfg', 'yolo.weights')
# 加载类别标签
classes = []
with open('yolo.names', 'r') as f:
classes = [line.strip() for line in f.readlines()]
# 加载测试图像
image = cv2.imread('image.jpg')
# 获取图像的宽度和高度
height, width, _ = image.shape
# 生成用于模型输入的blob
blob = cv2.dnn.blobFromImage(image, 1/255.0, (416, 416), swapRB=True, crop=False)
# 将blob输入到模型中进行前向推理
net.setInput(blob)
output_layers_names = net.getUnconnectedOutLayersNames()
layers_outputs = net.forward(output_layers_names)
# 解析预测结果
boxes = []
confidences = []
class_ids = []
for output in layers_outputs:
for detection in output:
scores = detection[5:]
class_id = np.argmax(scores)
confidence = scores[class_id]
if confidence > 0.5:
center_x = int(detection[0] * width)
center_y = int(detection[1] * height)
w = int(detection[2] * width)
h = int(detection[3] * height)
x = int(center_x - w / 2)
y = int(center_y - h / 2)
boxes.append([x, y, w, h])
confidences.append(float(confidence))
class_ids.append(class_id)
# 使用非极大值抑制排除重叠框
indices = cv2.dnn.NMSBoxes(boxes, confidences, 0.5, 0.4)
# 在图像上绘制检测结果
font = cv2.FONT_HERSHEY_SIMPLEX
for i in indices:
i = i[0]
box = boxes[i]
x, y, w, h = box
label = f"{classes[class_ids[i]]}: {confidences[i]:.2f}"
cv2.rectangle(image, (x, y), (x+w, y+h), (0, 255, 0), 2)
cv2.putText(image, label, (x, y-10), font, 1, (0, 255, 0), 2)
# 显示结果图像
cv2.imshow("Object Detection", image)
cv2.waitKey(0)
cv2.destroyAllWindows()
```
以上代码使用YOLO算法进行目标检测,通过导入已经训练好的模型和类别标签,对输入图像进行分析并绘制检测结果。代码中还包括了非极大值抑制等后处理步骤,以提高检测结果的准确性和稳定性。请注意,以上代码只是一个简单示例,实际应用中可能需要根据具体的场景和需求进行更多的调整和优化。
相关推荐
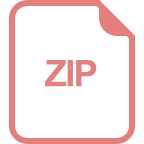
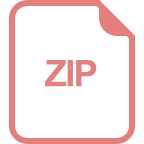
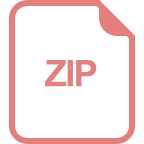














