遥感影像数据光谱特征,植被指数特征,纹理特征,树高特征,提取好后,利用随机森林分类代码
时间: 2023-07-28 11:09:43 浏览: 90
以下是使用Python中的scikit-learn库实现随机森林分类的代码,假设已经提取好了遥感影像数据的光谱特征、植被指数特征、纹理特征和树高特征,分别存储在X中,对应的分类标签存储在y中。
```python
from sklearn.ensemble import RandomForestClassifier
from sklearn.model_selection import train_test_split
# 将数据集拆分为训练集和测试集,其中测试集占20%
X_train, X_test, y_train, y_test = train_test_split(X, y, test_size=0.2, random_state=42)
# 定义随机森林分类器
rf_clf = RandomForestClassifier(n_estimators=100, random_state=42)
# 训练分类器
rf_clf.fit(X_train, y_train)
# 在测试集上进行预测
y_pred = rf_clf.predict(X_test)
# 计算准确率
accuracy = rf_clf.score(X_test, y_test)
print("随机森林分类器的准确率为:", accuracy)
```
其中,`n_estimators`参数指定了随机森林中决策树的数量,`random_state`参数指定了随机数种子,可以保证每次运行结果相同。最后输出的准确率即为分类器在测试集上的准确率。
相关问题
遥感影像光谱特征,植被指数特征,纹理特征,树高特征提取随机森林分类
随机森林是一种常用的分类算法,可以用于遥感影像分类。在遥感影像分类中,常用的特征包括光谱特征、植被指数特征、纹理特征和树高特征。
光谱特征是指在不同波段下,物体反射或辐射的强度和能量分布情况。通常使用多光谱影像进行分类,其中包括红、绿、蓝、近红外等波段。在随机森林分类中,可以将多光谱影像的各个波段作为特征进行提取。
植被指数特征是指将不同波段下的光谱信息进行组合,计算出反映植被生长情况的指数。例如,常用的归一化植被指数(NDVI)、归一化差异植被指数(NDWI)等。这些指数可以反映出不同植被类型的生长情况,可以作为分类的特征之一。
纹理特征是指图像中像素间的空间关系和灰度变化规律。在遥感影像分类中,通常使用灰度共生矩阵(GLCM)、灰度差值矩阵(GDV)等方法进行纹理特征的提取。这些特征可以反映出地物表面的纹理特征,例如草地、森林等不同地物类型的纹理特征不同。
树高特征是指树木高度的信息。在遥感影像中,可以使用数字高程模型(DEM)或激光雷达数据等获取地形高程信息,从而计算出地表高度。再结合遥感影像中的植被信息,可以计算出树高信息。这些特征可以反映出不同地物类型的高度差异,例如森林、草地等地物类型的高度差异较大。
以上这些特征可以作为随机森林分类的输入特征,通过训练得到分类器,从而实现遥感影像分类。在实际应用中,还需要结合地物类型的先验知识和实地调查数据进行分类结果的验证和修正。
遥感影像数据光谱特征,植被指数特征,纹理特征,树高特征随机森林分类代码
以下是一个使用随机森林对遥感影像数据进行分类的Python代码示例,其中包括光谱特征、植被指数特征、纹理特征和树高特征。
```python
import numpy as np
import pandas as pd
from sklearn.ensemble import RandomForestClassifier
from sklearn.metrics import accuracy_score
# 读取数据
data = pd.read_csv("data.csv")
# 将数据拆分为特征和标签
X = data.iloc[:, :-1].values
y = data.iloc[:, -1].values
# 划分训练集和测试集
from sklearn.model_selection import train_test_split
X_train, X_test, y_train, y_test = train_test_split(X, y, test_size=0.2, random_state=0)
# 使用随机森林模型进行分类
classifier = RandomForestClassifier(n_estimators=100, random_state=0)
classifier.fit(X_train, y_train)
# 预测测试集结果
y_pred = classifier.predict(X_test)
# 输出准确率
print("Accuracy:", accuracy_score(y_test, y_pred))
```
在这个示例中,我们使用了一个包含光谱特征、植被指数特征、纹理特征和树高特征的数据集。我们首先将数据拆分为特征和标签,然后将其划分为训练集和测试集。我们使用sklearn中的随机森林模型进行分类,并使用训练数据进行拟合。最后,我们用测试集数据进行预测,并输出准确率。
相关推荐
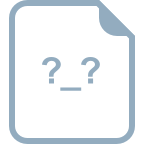
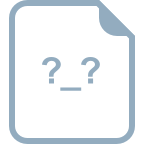
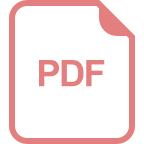











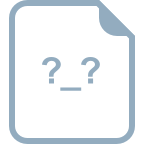
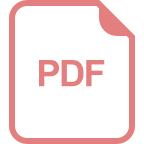