model = model.to("cuda:0") # 从测试集中挑选4个样本 test_examples = dev_dataset["document"][:4] inputs = tokenizer( test_examples, padding="max_length", truncation=True, max_length=max_input_length, return_tensors="pt", )这是什么意思
时间: 2024-02-23 07:56:47 浏览: 164
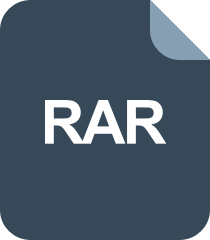
cuda-example.rar_cuda examples_cuda 例子_cuda学习
这段代码是为了在GPU上运行模型,同时用测试集中的前4个样本进行推理测试。其中,"model = model.to("cuda:0")" 是将模型转移到GPU设备上,以便在GPU上运行;"test_examples = dev_dataset["document"][:4]" 是从测试集中获取前4个样本;而 "inputs = tokenizer(test_examples, padding="max_length", truncation=True, max_length=max_input_length, return_tensors="pt")" 则是使用tokenizer将样本进行处理,使其符合模型输入的格式要求,其中包括对样本进行padding、截断、转换为PyTorch张量等操作。最终,我们可以使用这些处理后的样本输入到模型中进行推理。
阅读全文
相关推荐
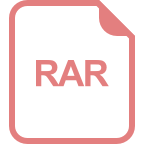
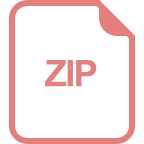
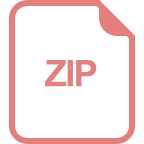
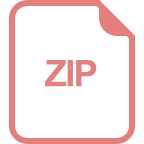
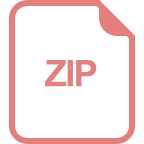
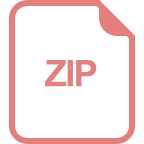
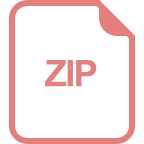
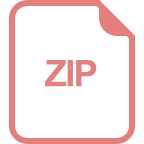
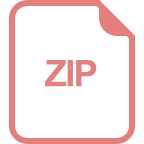
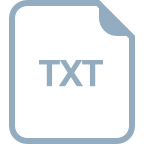
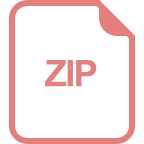
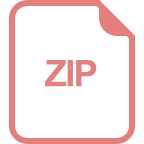
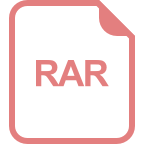
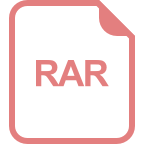
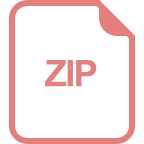
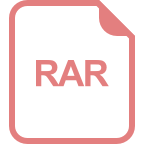