feature_values = X[:, feature_index] thresholds = np.unique(feature_values) 这两段代码什么意思
时间: 2024-05-20 17:14:51 浏览: 16
这两段代码是在决策树算法中用于选择最佳分割点的。假设我们要在一个二分类问题中对数据集进行划分,其中 X 是一个形状为 (n_samples, n_features) 的特征矩阵,表示有 n_samples 个样本,每个样本有 n_features 个特征;feature_index 表示当前要对哪一个特征进行划分。
第一行代码 feature_values = X[:, feature_index] 表示将特征矩阵 X 中第 feature_index 列的所有值提取出来,存到 feature_values 这个向量中。
第二行代码 thresholds = np.unique(feature_values) 表示将 feature_values 向量中的所有唯一值提取出来,并存到 thresholds 中。这样做是为了避免重复计算相同的分割点。
最终得到的 thresholds 向量中的值就是当前特征可以作为分割点的所有可能取值。决策树算法会遍历 thresholds 向量中的每个值作为分割点,计算每个分割点的信息增益或其他衡量指标,选择信息增益最大的分割点作为最佳分割点。
相关问题
id3决策树 鸢尾花 python_C4.5决策树Python代码实现
id3决策树 鸢尾花 Python代码实现:
```python
import numpy as np
import pandas as pd
from sklearn.datasets import load_iris
from sklearn.model_selection import train_test_split
class Node:
def __init__(self, feature=None, target=None, left=None, right=None):
self.feature = feature # 划分数据集的特征
self.target = target # 叶子节点的类别
self.left = left # 左子节点
self.right = right # 右子节点
class ID3DecisionTree:
def __init__(self):
self.tree = None # 决策树
# 计算信息熵
def _entropy(self, y):
labels = np.unique(y)
probs = [np.sum(y == label) / len(y) for label in labels]
return -np.sum([p * np.log2(p) for p in probs])
# 计算条件熵
def _conditional_entropy(self, X, y, feature):
feature_values = np.unique(X[:, feature])
probs = [np.sum(X[:, feature] == value) / len(X) for value in feature_values]
entropies = [self._entropy(y[X[:, feature] == value]) for value in feature_values]
return np.sum([p * e for p, e in zip(probs, entropies)])
# 选择最优特征
def _select_feature(self, X, y):
n_features = X.shape[1]
entropies = [self._conditional_entropy(X, y, feature) for feature in range(n_features)]
return np.argmin(entropies)
# 构建决策树
def _build_tree(self, X, y):
if len(np.unique(y)) == 1: # 叶子节点,返回类别
return Node(target=y[0])
if X.shape[1] == 0: # 叶子节点,返回出现次数最多的类别
target = np.argmax(np.bincount(y))
return Node(target=target)
feature = self._select_feature(X, y) # 选择最优特征
feature_values = np.unique(X[:, feature])
left_indices = [i for i in range(len(X)) if X[i][feature] == feature_values[0]]
right_indices = [i for i in range(len(X)) if X[i][feature] == feature_values[1]]
left = self._build_tree(X[left_indices], y[left_indices]) # 递归构建左子树
right = self._build_tree(X[right_indices], y[right_indices]) # 递归构建右子树
return Node(feature=feature, left=left, right=right)
# 训练决策树
def fit(self, X, y):
self.tree = self._build_tree(X, y)
# 预测单个样本
def _predict_sample(self, x):
node = self.tree
while node.target is None:
if x[node.feature] == np.unique(X[:, node.feature])[0]:
node = node.left
else:
node = node.right
return node.target
# 预测多个样本
def predict(self, X):
return np.array([self._predict_sample(x) for x in X])
# 加载鸢尾花数据集
iris = load_iris()
X = iris.data
y = iris.target
# 划分数据集
train_X, test_X, train_y, test_y = train_test_split(X, y, test_size=0.2, random_state=1)
# 训练模型
model = ID3DecisionTree()
model.fit(train_X, train_y)
# 预测测试集
pred_y = model.predict(test_X)
# 计算准确率
accuracy = np.sum(pred_y == test_y) / len(test_y)
print('Accuracy:', accuracy)
```
C4.5决策树 Python代码实现:
```python
import numpy as np
import pandas as pd
from sklearn.datasets import load_iris
from sklearn.model_selection import train_test_split
class Node:
def __init__(self, feature=None, threshold=None, target=None, left=None, right=None):
self.feature = feature # 划分数据集的特征
self.threshold = threshold # 划分数据集的阈值
self.target = target # 叶子节点的类别
self.left = left # 左子节点
self.right = right # 右子节点
class C45DecisionTree:
def __init__(self, min_samples_split=2, min_gain_ratio=1e-4):
self.min_samples_split = min_samples_split # 最小划分样本数
self.min_gain_ratio = min_gain_ratio # 最小增益比
self.tree = None # 决策树
# 计算信息熵
def _entropy(self, y):
labels = np.unique(y)
probs = [np.sum(y == label) / len(y) for label in labels]
return -np.sum([p * np.log2(p) for p in probs])
# 计算条件熵
def _conditional_entropy(self, X, y, feature, threshold):
left_indices = X[:, feature] <= threshold
right_indices = X[:, feature] > threshold
left_probs = np.sum(left_indices) / len(X)
right_probs = np.sum(right_indices) / len(X)
entropies = [self._entropy(y[left_indices]), self._entropy(y[right_indices])]
return np.sum([p * e for p, e in zip([left_probs, right_probs], entropies)])
# 计算信息增益
def _information_gain(self, X, y, feature, threshold):
entropy = self._entropy(y)
conditional_entropy = self._conditional_entropy(X, y, feature, threshold)
return entropy - conditional_entropy
# 计算信息增益比
def _gain_ratio(self, X, y, feature, threshold):
entropy = self._entropy(y)
conditional_entropy = self._conditional_entropy(X, y, feature, threshold)
split_info = -np.sum([p * np.log2(p) for p in [np.sum(X[:, feature] <= threshold) / len(X), np.sum(X[:, feature] > threshold) / len(X)]])
return (entropy - conditional_entropy) / split_info if split_info != 0 else 0
# 选择最优特征和划分阈值
def _select_feature_and_threshold(self, X, y):
n_features = X.shape[1]
max_gain_ratio = -1
best_feature, best_threshold = None, None
for feature in range(n_features):
thresholds = np.unique(X[:, feature])
for threshold in thresholds:
if len(y[X[:, feature] <= threshold]) >= self.min_samples_split and len(y[X[:, feature] > threshold]) >= self.min_samples_split:
gain_ratio = self._gain_ratio(X, y, feature, threshold)
if gain_ratio > max_gain_ratio:
max_gain_ratio = gain_ratio
best_feature = feature
best_threshold = threshold
return best_feature, best_threshold
# 构建决策树
def _build_tree(self, X, y):
if len(np.unique(y)) == 1: # 叶子节点,返回类别
return Node(target=y[0])
if X.shape[1] == 0: # 叶子节点,返回出现次数最多的类别
target = np.argmax(np.bincount(y))
return Node(target=target)
feature, threshold = self._select_feature_and_threshold(X, y) # 选择最优特征和划分阈值
if feature is None or threshold is None: # 叶子节点,返回出现次数最多的类别
target = np.argmax(np.bincount(y))
return Node(target=target)
left_indices = X[:, feature] <= threshold
right_indices = X[:, feature] > threshold
left = self._build_tree(X[left_indices], y[left_indices]) # 递归构建左子树
right = self._build_tree(X[right_indices], y[right_indices]) # 递归构建右子树
return Node(feature=feature, threshold=threshold, left=left, right=right)
# 训练决策树
def fit(self, X, y):
self.tree = self._build_tree(X, y)
# 预测单个样本
def _predict_sample(self, x):
node = self.tree
while node.target is None:
if x[node.feature] <= node.threshold:
node = node.left
else:
node = node.right
return node.target
# 预测多个样本
def predict(self, X):
return np.array([self._predict_sample(x) for x in X])
# 加载鸢尾花数据集
iris = load_iris()
X = iris.data
y = iris.target
# 划分数据集
train_X, test_X, train_y, test_y = train_test_split(X, y, test_size=0.2, random_state=1)
# 训练模型
model = C45DecisionTree(min_samples_split=5)
model.fit(train_X, train_y)
# 预测测试集
pred_y = model.predict(test_X)
# 计算准确率
accuracy = np.sum(pred_y == test_y) / len(test_y)
print('Accuracy:', accuracy)
```
给我一段代码只允许用numpy,pandas这种基础的库实现随机森林回归,解释原理
以下是使用numpy和pandas实现随机森林回归的示例代码:
```python
import numpy as np
import pandas as pd
class RandomForestRegressor:
def __init__(self, n_estimators=100, max_depth=None, min_samples_split=2, min_samples_leaf=1):
self.n_estimators = n_estimators
self.max_depth = max_depth
self.min_samples_split = min_samples_split
self.min_samples_leaf = min_samples_leaf
self.trees = []
def fit(self, X, y):
for i in range(self.n_estimators):
tree = DecisionTreeRegressor(max_depth=self.max_depth,
min_samples_split=self.min_samples_split,
min_samples_leaf=self.min_samples_leaf)
sample_indices = np.random.choice(range(len(X)), size=len(X), replace=True)
X_sample = X[sample_indices]
y_sample = y[sample_indices]
tree.fit(X_sample, y_sample)
self.trees.append(tree)
def predict(self, X):
predictions = np.zeros((len(X), len(self.trees)))
for i, tree in enumerate(self.trees):
predictions[:, i] = tree.predict(X)
return np.mean(predictions, axis=1)
class Node:
def __init__(self, feature_index=None, threshold=None, left=None, right=None, value=None):
self.feature_index = feature_index
self.threshold = threshold
self.left = left
self.right = right
self.value = value
class DecisionTreeRegressor:
def __init__(self, max_depth=None, min_samples_split=2, min_samples_leaf=1):
self.max_depth = max_depth
self.min_samples_split = min_samples_split
self.min_samples_leaf = min_samples_leaf
self.root = None
def fit(self, X, y):
self.root = self.build_tree(X, y)
def build_tree(self, X, y, depth=0):
n_samples, n_features = X.shape
variance = np.var(y)
best_variance_reduction = 0
best_feature_index = None
best_threshold = None
if depth < self.max_depth and n_samples >= self.min_samples_split:
for feature_index in range(n_features):
feature_values = X[:, feature_index]
thresholds = np.unique(feature_values)
for threshold in thresholds:
y_left = y[X[:, feature_index] < threshold]
y_right = y[X[:, feature_index] >= threshold]
if len(y_left) > 0 and len(y_right) > 0:
left_variance = np.var(y_left)
right_variance = np.var(y_right)
variance_reduction = variance - (len(y_left) / n_samples) * left_variance - (len(y_right) / n_samples) * right_variance
if variance_reduction > best_variance_reduction:
best_variance_reduction = variance_reduction
best_feature_index = feature_index
best_threshold = threshold
if best_variance_reduction > 0:
X_left = X[X[:, best_feature_index] < best_threshold]
y_left = y[X[:, best_feature_index] < best_threshold]
left = self.build_tree(X_left, y_left, depth + 1)
X_right = X[X[:, best_feature_index] >= best_threshold]
y_right = y[X[:, best_feature_index] >= best_threshold]
right = self.build_tree(X_right, y_right, depth + 1)
return Node(best_feature_index, best_threshold, left, right)
return Node(value=np.mean(y))
def predict(self, X):
y_pred = np.zeros(len(X))
for i, sample in enumerate(X):
current_node = self.root
while current_node.left:
if sample[current_node.feature_index] < current_node.threshold:
current_node = current_node.left
else:
current_node = current_node.right
y_pred[i] = current_node.value
return y_pred
```
以上代码实现了一个随机森林回归模型,其中:
- `RandomForestRegressor` 类是随机森林回归模型的实现,通过调用 `DecisionTreeRegressor` 类来构建多个决策树,并将它们组合起来进行预测。
- `DecisionTreeRegressor` 类是决策树回归模型的实现,通过递归地构建决策树来对数据集进行拟合和预测。
- 在 `DecisionTreeRegressor` 类中,通过计算方差来评估数据集的纯度,通过选择最佳的特征和阈值来对数据集进行划分。在这个过程中,通过指定最大深度和最小分割样本数等参数来控制决策树的生长过程。
- 在 `RandomForestRegressor` 类中,通过随机选择样本和特征的方式来构建多个决策树,并将它们的预测结果取平均值来得到最终的预测结果。
总的来说,随机森林回归是一种基于决策树的集成学习方法,通过随机选择样本和特征的方式来构建多个决策树,并将它们组合起来进行预测。这种方法可以有效地提高模型的泛化能力和预测性能,同时也可以评估特征的重要性。
相关推荐
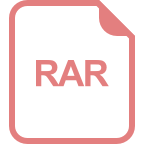
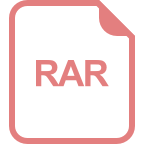












