对刚才第三个策略举个代码例子,如何通过注意力机制给不同的时间步的特征赋予不同的权重,采用batchfirst=True
时间: 2024-09-12 15:07:32 浏览: 41
在深度学习模型中,特别是在序列模型如循环神经网络(RNN)、长短期记忆网络(LSTM)或Transformer模型中,注意力机制(Attention Mechanism)被广泛用于为序列的不同部分分配不同的权重。这可以帮助模型更好地关注于序列中重要的部分,以进行有效的信息编码或翻译任务。
以下是一个简单的例子,使用PyTorch框架来展示如何在编码器-解码器模型中使用注意力机制来给不同的时间步的特征赋予不同的权重。这个例子假设你已经有一个已经训练好的编码器模型,它能够输出每个时间步的状态向量。
```python
import torch
import torch.nn as nn
import torch.nn.functional as F
class Attention(nn.Module):
def __init__(self, hidden_size):
super(Attention, self).__init__()
self.hidden_size = hidden_size
self.att_weights = nn.Parameter(torch.Tensor(1, hidden_size))
nn.init.xavier_uniform_(self.att_weights.data)
def forward(self, encoder_outputs):
# encoder_outputs: [batch_size, seq_len, hidden_size]
# 计算注意力分数
attention_scores = torch.matmul(self.att_weights, encoder_outputs.transpose(1, 2))
# attention_scores: [batch_size, 1, seq_len]
# 应用softmax激活函数得到权重
attention_weights = F.softmax(attention_scores.squeeze(1), dim=1).unsqueeze(1)
# attention_weights: [batch_size, 1, seq_len]
# 应用权重得到加权的上下文向量
context = torch.matmul(attention_weights, encoder_outputs)
# context: [batch_size, 1, hidden_size]
return context, attention_weights
# 假设hidden_size为256
attention_layer = Attention(hidden_size=256)
batch_size = 10
seq_len = 20
hidden_size = 256
# 生成一些假的编码器输出
encoder_outputs = torch.rand(batch_size, seq_len, hidden_size)
# 使用注意力机制
context, attention_weights = attention_layer(encoder_outputs)
print("Context Vector:", context.shape) # 应该是 [batch_size, 1, hidden_size]
print("Attention Weights:", attention_weights.shape) # 应该是 [batch_size, 1, seq_len]
```
在这个例子中,我们首先定义了一个`Attention`类,它计算了一个简单的注意力分数,然后使用softmax函数来得到每个时间步的注意力权重。最后,这些权重被用来计算一个加权的上下文向量,它代表了整个序列的一个加权表示。
阅读全文
相关推荐
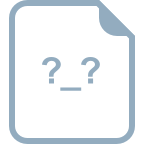
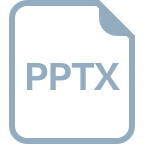
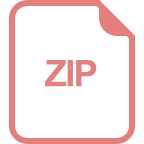
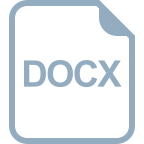
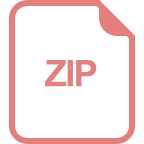
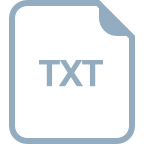
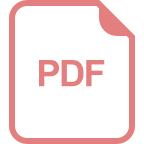
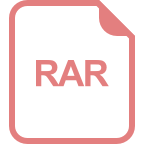
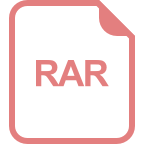
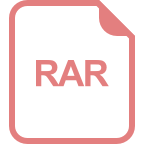
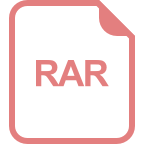
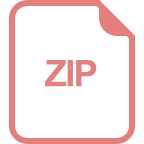
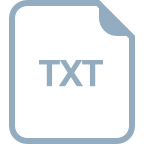
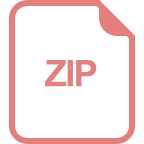
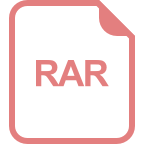
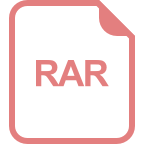
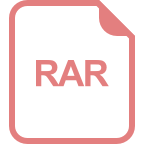
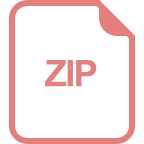