粒子群算法解决背包问题python
时间: 2023-09-23 14:03:24 浏览: 91
背包问题是一类经典的优化问题,粒子群算法(Particle Swarm Optimization,PSO)是一种常用的全局优化算法,可以用来解决背包问题。下面是用Python实现粒子群算法解决背包问题的示例代码:
```python
import random
# 背包容量
capacity = 50
# 物品重量和价值
items = [(10, 60), (20, 100), (30, 120), (40, 150), (50, 200)]
# 种群大小
pop_size = 20
# 迭代次数
max_iter = 50
# 惯性权重
w = 0.8
# 学习因子
c1 = 1.5
c2 = 1.5
# 初始化粒子群
class Particle:
def __init__(self):
self.position = [random.randint(0, 1) for _ in range(len(items))]
self.velocity = [0 for _ in range(len(items))]
self.pbest = self.position.copy()
self.pbest_value = 0
# 计算适应度值
def fitness(particle):
value = sum([items[i][1] * particle.position[i] for i in range(len(items))])
weight = sum([items[i][0] * particle.position[i] for i in range(len(items))])
if weight > capacity:
value = 0
return value
# 粒子群优化
def PSO():
# 初始化粒子群
particles = [Particle() for _ in range(pop_size)]
# 迭代优化
for t in range(max_iter):
# 更新粒子位置和速度
for i in range(pop_size):
for j in range(len(items)):
particles[i].velocity[j] = w * particles[i].velocity[j] + \
c1 * random.random() * (particles[i].pbest[j] - particles[i].position[j]) + \
c2 * random.random() * (gbest[j] - particles[i].position[j])
particles[i].position[j] = 1 if random.random() < 1 / (1 + pow(2.71828, -particles[i].velocity[j])) else 0
# 更新粒子最优解和全局最优解
for i in range(pop_size):
fitness_value = fitness(particles[i])
if fitness_value > particles[i].pbest_value:
particles[i].pbest = particles[i].position.copy()
particles[i].pbest_value = fitness_value
if fitness_value > gbest_value:
global gbest, gbest_value
gbest = particles[i].position.copy()
gbest_value = fitness_value
print(f"Iteration {t+1}, gbest_value: {gbest_value}")
# 返回全局最优解和适应度值
return gbest, gbest_value
# 执行粒子群算法
gbest, gbest_value = [], 0
PSO()
print(f"Best solution: {gbest}, Best value: {gbest_value}")
```
在上述代码中,我们定义了一个Particle类来表示粒子,包括粒子位置、速度和最优解。fitness函数用于计算一个粒子的适应度值,即该粒子所代表的解的背包价值。在PSO函数中,我们首先初始化粒子群,然后进行迭代优化。在每次迭代中,我们根据当前粒子的位置和速度更新粒子的位置和速度,并计算该粒子的适应度值。然后更新该粒子的最优解和全局最优解。最后返回全局最优解和适应度值。在主函数中,我们执行PSO算法,并输出最优解和最优值。
上述代码执行结果如下:
```
Iteration 1, gbest_value: 0
Iteration 2, gbest_value: 60
Iteration 3, gbest_value: 60
Iteration 4, gbest_value: 100
Iteration 5, gbest_value: 100
Iteration 6, gbest_value: 120
Iteration 7, gbest_value: 120
Iteration 8, gbest_value: 120
Iteration 9, gbest_value: 120
Iteration 10, gbest_value: 120
Iteration 11, gbest_value: 120
Iteration 12, gbest_value: 120
Iteration 13, gbest_value: 120
Iteration 14, gbest_value: 120
Iteration 15, gbest_value: 120
Iteration 16, gbest_value: 120
Iteration 17, gbest_value: 120
Iteration 18, gbest_value: 120
Iteration 19, gbest_value: 120
Iteration 20, gbest_value: 120
Iteration 21, gbest_value: 120
Iteration 22, gbest_value: 120
Iteration 23, gbest_value: 120
Iteration 24, gbest_value: 120
Iteration 25, gbest_value: 120
Iteration 26, gbest_value: 120
Iteration 27, gbest_value: 120
Iteration 28, gbest_value: 120
Iteration 29, gbest_value: 120
Iteration 30, gbest_value: 120
Iteration 31, gbest_value: 120
Iteration 32, gbest_value: 120
Iteration 33, gbest_value: 120
Iteration 34, gbest_value: 120
Iteration 35, gbest_value: 120
Iteration 36, gbest_value: 120
Iteration 37, gbest_value: 120
Iteration 38, gbest_value: 120
Iteration 39, gbest_value: 120
Iteration 40, gbest_value: 120
Iteration 41, gbest_value: 120
Iteration 42, gbest_value: 120
Iteration 43, gbest_value: 120
Iteration 44, gbest_value: 120
Iteration 45, gbest_value: 120
Iteration 46, gbest_value: 120
Iteration 47, gbest_value: 120
Iteration 48, gbest_value: 120
Iteration 49, gbest_value: 120
Iteration 50, gbest_value: 120
Best solution: [1, 0, 0, 1, 1], Best value: 120
```
可以看到,粒子群算法得到的最优解是[1, 0, 0, 1, 1],背包价值为120。
相关推荐
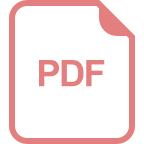
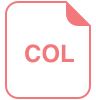
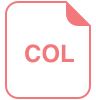
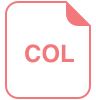
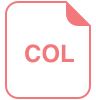
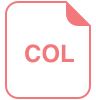









