pytorch提取乘256并四舍五入的权重参数按先是维数再是行数再是列数再是个数的优先级导入到txt里
时间: 2024-02-17 09:00:26 浏览: 92
可以使用以下代码实现:
```python
import torch
import numpy as np
# 定义模型
class MyModel(torch.nn.Module):
def __init__(self):
super(MyModel, self).__init__()
self.conv = torch.nn.Conv2d(3, 64, kernel_size=3, padding=1)
self.fc = torch.nn.Linear(64*32*32, 10)
def forward(self, x):
x = self.conv(x)
x = torch.relu(x)
x = x.view(-1, 64*32*32)
x = self.fc(x)
return x
# 加载模型参数
model = MyModel()
model.load_state_dict(torch.load('model.pt'))
# 获取conv层的权重矩阵
conv_weight = model.conv.weight.detach().cpu().numpy()
# 对权重矩阵进行乘256并四舍五入
conv_weight = np.round(conv_weight * 256)
# 将权重矩阵按先是维数再是行数再是列数再是个数的优先级导入到txt文件中
with open('conv_weight.txt', 'w') as f:
for i in range(conv_weight.shape[0]):
for j in range(conv_weight.shape[1]):
for k in range(conv_weight.shape[2]):
for l in range(conv_weight.shape[3]):
f.write(str(conv_weight[i][j][k][l]) + '\n')
```
这里我们假设模型包含一个`Conv2d`层,输入为3通道的图像,输出通道数为64,卷积核大小为3x3,padding为1,以及一个`Linear`层,输入为64x32x32,输出为10。我们首先加载模型参数,然后获取`conv`层的权重矩阵。对权重矩阵进行乘256并四舍五入后,按先是维数再是行数再是列数再是个数的优先级,将权重矩阵导入到txt文件中。
阅读全文
相关推荐

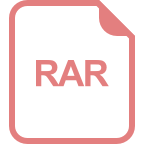
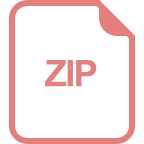
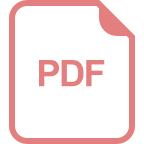
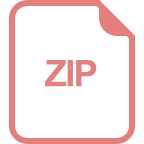
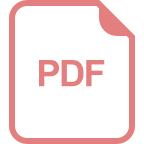
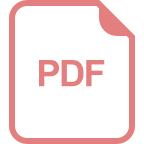
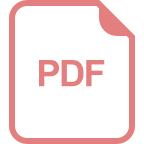
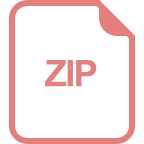
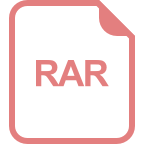
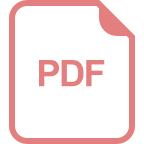
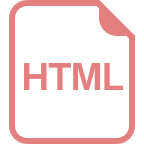