shape regularization effect
时间: 2023-09-23 10:08:56 浏览: 58
"Shape regularization effect" refers to the phenomenon where a model that is designed to preferentially learn certain types of patterns or structures in the data tends to produce outputs that are smoother and more regular than those produced by a model that is not subject to such constraints.
For example, in image processing, a model that is designed to preferentially learn edges and contours in images may produce smoother, more regular edges than a model that is not designed to do so. Similarly, in natural language processing, a language model that is designed to preferentially learn grammatically correct and semantically coherent sentences may produce outputs that are more coherent and less garbled than a model that is not subject to such constraints.
The shape regularization effect is a desirable property of many machine learning models, as it can help to improve the generalization performance of the model and reduce overfitting. However, it is important to strike a balance between regularization and flexibility, as too much regularization can lead to underfitting and poor performance on the training data.
相关推荐
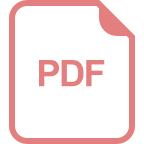














