resnet18残差块
时间: 2023-10-31 12:07:33 浏览: 107
ResNet18 是一种深度残差网络,其中的残差块是其核心组成部分。每个残差块由两个卷积层和一个跳跃连接组成。跳跃连接将输入直接添加到残差块的输出中,以便在训练期间更好地传播梯度并减轻梯度消失问题。
具体来说,ResNet18 的残差块包括以下几个步骤:
1. 输入通过一个卷积层进行卷积操作。
2. 将卷积层的输出传递给另一个卷积层进行卷积操作。
3. 将第二个卷积层的输出与输入相加,得到残差块的输出。
这个过程可以用以下代码表示:
```
class ResidualBlock(nn.Module):
def __init__(self, in_channels, out_channels, stride=1):
super(ResidualBlock, self).__init__()
self.conv1 = nn.Conv2d(in_channels, out_channels, kernel_size=3, stride=stride, padding=1, bias=False)
self.bn1 = nn.BatchNorm2d(out_channels)
self.conv2 = nn.Conv2d(out_channels, out_channels, kernel_size=3, stride=1, padding=1, bias=False)
self.bn2 = nn.BatchNorm2d(out_channels)
self.relu = nn.ReLU(inplace=True)
self.stride = stride
def forward(self, x):
identity = x
out = self.conv1(x)
out = self.bn1(out)
out = self.relu(out)
out = self.conv2(out)
out = self.bn2(out)
if self.stride != 1 or identity.shape[1] != out.shape[1]:
identity = nn.Conv2d(identity.shape[1], out.shape[1], kernel_size=1, stride=self.stride, bias=False)(identity)
identity = nn.BatchNorm2d(out.shape[1])(identity)
out += identity
out = self.relu(out)
return out
```
阅读全文
相关推荐
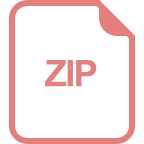
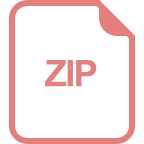
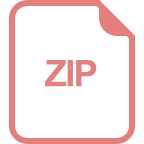















