将下面代码简洁化:def split_dataset(img_path, target_folder_path, output_path): filename = [] total_imgs = os.listdir(img_path) #for root, dirs, files in os.walk(img_path): for img in total_imgs: filename.append(img) np.random.shuffle(filename) train = filename[:int(len(filename) * 0.9)] test = filename[int(len(filename) * 0.9):] out_images = os.path.join(output_path, 'imgs') if not os.path.exists(out_images): os.makedirs(out_images) out_images_train = os.path.join(out_images, 'training') if not os.path.exists(out_images_train): os.makedirs(out_images_train) out_images_test = os.path.join(out_images, 'test') if not os.path.exists(out_images_test): os.makedirs(out_images_test) out_annotations = os.path.join(output_path, 'annotations') if not os.path.exists(out_annotations): os.makedirs(out_annotations) out_annotations_train = os.path.join(out_annotations, 'training') if not os.path.exists(out_annotations_train): os.makedirs(out_annotations_train) out_annotations_test = os.path.join(out_annotations, 'test') if not os.path.exists(out_annotations_test): os.makedirs(out_annotations_test) for i in train: print(os.path.join(img_path, i)) print(os.path.join(out_images_train, i)) shutil.copyfile(os.path.join(img_path, i), os.path.join(out_images_train, i)) annotations_name = "gt_" + i[:-3] + 'txt' shutil.copyfile(os.path.join(target_folder_path, annotations_name), os.path.join(out_annotations_train, annotations_name)) for i in test: shutil.copyfile(os.path.join(img_path, i), os.path.join(out_images_test, i)) annotations_name = "gt_" + i[:-3] + 'txt' shutil.copyfile(os.path.join(target_folder_path, annotations_name), os.path.join(out_annotations_test, annotations_name))
时间: 2024-04-11 20:32:44 浏览: 18
def split_dataset(img_path, target_folder_path, output_path):
filename = os.listdir(img_path)
np.random.shuffle(filename)
train = filename[:int(len(filename) * 0.9)]
test = filename[int(len(filename) * 0.9):]
out_images = os.path.join(output_path, 'imgs')
os.makedirs(out_images, exist_ok=True)
out_images_train = os.path.join(out_images, 'training')
os.makedirs(out_images_train, exist_ok=True)
out_images_test = os.path.join(out_images, 'test')
os.makedirs(out_images_test, exist_ok=True)
out_annotations = os.path.join(output_path, 'annotations')
os.makedirs(out_annotations, exist_ok=True)
out_annotations_train = os.path.join(out_annotations, 'training')
os.makedirs(out_annotations_train, exist_ok=True)
out_annotations_test = os.path.join(out_annotations, 'test')
os.makedirs(out_annotations_test, exist_ok=True)
for i in train:
img_src = os.path.join(img_path, i)
img_dst = os.path.join(out_images_train, i)
shutil.copyfile(img_src, img_dst)
annotations_name = "gt_" + i[:-3] + 'txt'
annotations_src = os.path.join(target_folder_path, annotations_name)
annotations_dst = os.path.join(out_annotations_train, annotations_name)
shutil.copyfile(annotations_src, annotations_dst)
for i in test:
img_src = os.path.join(img_path, i)
img_dst = os.path.join(out_images_test, i)
shutil.copyfile(img_src, img_dst)
annotations_name = "gt_" + i[:-3] + 'txt'
annotations_src = os.path.join(target_folder_path, annotations_name)
annotations_dst = os.path.join(out_annotations_test, annotations_name)
shutil.copyfile(annotations_src, annotations_dst)
相关推荐
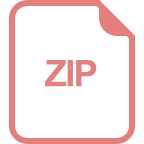
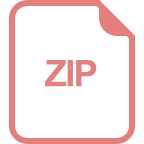














