pytorch训练模型并保存最优模型的代码
时间: 2023-08-11 22:03:12 浏览: 91
以下是PyTorch训练模型并保存最优模型的代码示例:
```python
import torch
import torch.nn as nn
import torch.optim as optim
from torch.utils.data import DataLoader
from torchvision import datasets, transforms
# Define the device to use
device = torch.device("cuda" if torch.cuda.is_available() else "cpu")
# Define the transforms for data preprocessing
transform = transforms.Compose([
transforms.ToTensor(),
transforms.Normalize((0.5,), (0.5,))
])
# Load the MNIST dataset
train_dataset = datasets.MNIST('data/', train=True, download=True, transform=transform)
test_dataset = datasets.MNIST('data/', train=False, download=True, transform=transform)
# Define the data loaders
train_loader = DataLoader(train_dataset, batch_size=64, shuffle=True)
test_loader = DataLoader(test_dataset, batch_size=64, shuffle=False)
# Define the model architecture
class Net(nn.Module):
def __init__(self):
super(Net, self).__init__()
self.fc1 = nn.Linear(784, 128)
self.fc2 = nn.Linear(128, 64)
self.fc3 = nn.Linear(64, 10)
self.dropout = nn.Dropout(0.2)
def forward(self, x):
x = x.view(x.shape[0], -1)
x = self.dropout(torch.relu(self.fc1(x)))
x = self.dropout(torch.relu(self.fc2(x)))
x = self.fc3(x)
return x
model = Net().to(device)
# Define the loss function and optimizer
criterion = nn.CrossEntropyLoss()
optimizer = optim.Adam(model.parameters(), lr=0.001)
# Train the model
best_accuracy = 0.0
for epoch in range(10):
train_loss = 0.0
train_accuracy = 0.0
for images, labels in train_loader:
images, labels = images.to(device), labels.to(device)
optimizer.zero_grad()
outputs = model(images)
loss = criterion(outputs, labels)
loss.backward()
optimizer.step()
train_loss += loss.item() * images.size(0)
_, predicted = torch.max(outputs.data, 1)
train_accuracy += (predicted == labels).sum().item()
train_loss = train_loss / len(train_loader.dataset)
train_accuracy = train_accuracy / len(train_loader.dataset)
print(f"Epoch {epoch+1} - Training loss: {train_loss:.4f}, Training accuracy: {train_accuracy:.4f}")
# Evaluate the model on the test set
test_accuracy = 0.0
with torch.no_grad():
for images, labels in test_loader:
images, labels = images.to(device), labels.to(device)
outputs = model(images)
_, predicted = torch.max(outputs.data, 1)
test_accuracy += (predicted == labels).sum().item()
test_accuracy = test_accuracy / len(test_loader.dataset)
print(f"Epoch {epoch+1} - Test accuracy: {test_accuracy:.4f}")
# Save the best model based on test accuracy
if test_accuracy > best_accuracy:
best_accuracy = test_accuracy
torch.save(model.state_dict(), 'best_model.pth')
print("Training complete!")
```
这段代码使用MNIST数据集训练一个简单的神经网络模型,并保存在测试集上表现最好的模型参数到`best_model.pth`文件中。在训练过程中,每个epoch结束后,模型会在测试集上计算准确率,如果当前模型的测试准确率比之前的最高准确率要高,就保存这个模型的参数。最后,训练完成后输出"Training complete!"。
相关推荐
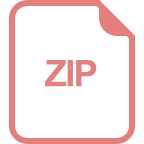
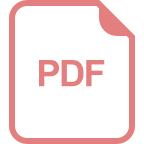
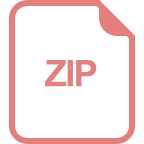
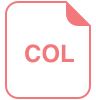
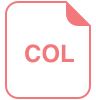
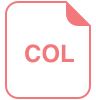
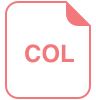
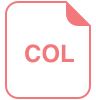









