使用pytorch的lstm预测车辆轨迹的python代码
时间: 2023-02-07 08:01:19 浏览: 191
下面是一个使用 PyTorch LSTM 来预测车辆轨迹的 Python 代码示例:
```python
import torch
import torch.nn as nn
class LSTM(nn.Module):
def __init__(self, input_size, hidden_size, num_layers, num_classes):
super(LSTM, self).__init__()
self.hidden_size = hidden_size
self.num_layers = num_layers
self.lstm = nn.LSTM(input_size, hidden_size, num_layers, batch_first=True)
self.fc = nn.Linear(hidden_size, num_classes)
def forward(self, x):
# Set initial hidden and cell states
h0 = torch.zeros(self.num_layers, x.size(0), self.hidden_size).to(device)
c0 = torch.zeros(self.num_layers, x.size(0), self.hidden_size).to(device)
# Forward propagate LSTM
out, _ = self.lstm(x, (h0, c0)) # out: tensor of shape (batch_size, seq_length, hidden_size)
# Decode the hidden state of the last time step
out = self.fc(out[:, -1, :])
return out
# Define the model
input_size = 1 # input size is 1 dimension (x, y, z coordinates of a single time step)
hidden_size = 8 # hidden size of the LSTM
num_layers = 1 # number of LSTM layers
num_classes = 2 # number of output classes (x, y coordinates of the next time step)
model = LSTM(input_size, hidden_size, num_layers, num_classes)
# Define the loss function and optimizer
criterion = nn.MSELoss()
optimizer = torch.optim.Adam(model.parameters(), lr=learning_rate)
# Train the model
for epoch in range(num_epochs):
for i, (inputs, labels) in enumerate(train_loader):
inputs = inputs.to(device)
labels = labels.to(device)
# Forward pass
outputs = model(inputs)
loss = criterion(outputs, labels)
# Backward and optimize
optimizer.zero_grad()
loss.backward()
optimizer.step()
if (i+1) % 100 == 0:
print(f'Epoch [{epoch+1}/{num_epochs}], Step [{i+1}/{total_step}], Loss: {loss.item():.4f}')
# Test the model
with torch.no_grad():
correct = 0
total = 0
for inputs, labels in test_loader:
inputs = inputs.to(device)
labels = labels.to(device)
outputs =
阅读全文
相关推荐
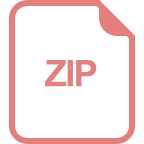
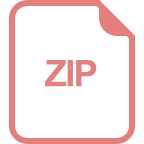
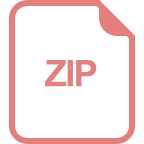










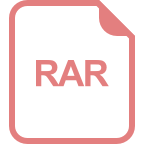
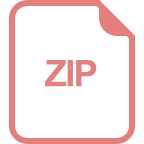
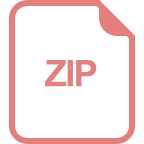
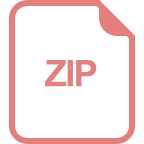
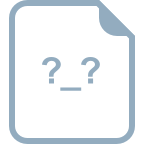
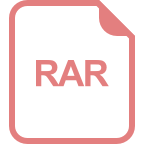
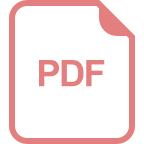