if 0 < dropout: layers.append(nn.Dropout(dropout)) layers.append(weight_norm(nn.Linear(dims[-2], dims[-1]), dim=None)) if '' != act: layers.append(getattr(nn, act)())怎么理解
时间: 2023-05-19 20:05:41 浏览: 47
这段代码是一个神经网络的层定义,其中包括一个可选的 dropout 层、一个线性层和一个可选的激活函数层。如果 dropout 参数大于 0,则添加一个 dropout 层。然后添加一个线性层,该层的输入维度为 dims[-2],输出维度为 dims[-1]。最后,如果 act 参数不为空,则添加一个激活函数层,该层的类型由 act 参数指定。
相关问题
layers=[] if 0 < dropout: layers.append(nn.Dropout(dropout))是什么意思
这段代码是在构建神经网络的层时使用的,其中 layers 是一个列表,用于存储神经网络的各个层。如果 dropout 大于 0,则会在神经网络的每一层之间添加一个 Dropout 层,以防止过拟合。如果 dropout 等于 0,则不会添加 Dropout 层。
class MLP(nn.Module): def __init__(self, input_dim, fc_dims, dropout_p=0.4, use_batchnorm=False): super(MLP, self).__init__() if isinstance(fc_dims, Iterable): fc_dims = list(fc_dims) assert isinstance(fc_dims, (list, tuple)), 'fc_dims must be either a list or a tuple, but got {}'.format( type(fc_dims)) layers = [] for dim in fc_dims: layers.append(nn.Linear(input_dim, dim)) if use_batchnorm and dim != 1: layers.append(nn.BatchNorm1d(dim)) if dim != 1: layers.append(nn.ReLU(inplace=True)) if dropout_p is not None and dim != 1: layers.append(nn.Dropout(p=dropout_p)) input_dim = dim self.fc_layers = nn.Sequential(*layers) def forward(self, input): return self.fc_layers(input)
这段代码定义了一个多层感知机(MLP)的神经网络模型。
在 `__init__` 方法中,模型的各个组件和参数被定义:
- `input_dim` 表示输入特征的维度。
- `fc_dims` 是一个列表,表示隐藏层的维度。每个元素是一个整数,表示该隐藏层的维度大小。
- `dropout_p` 是一个浮点数,表示在隐藏层之间进行 dropout 的概率。默认值为 0.4。
- `use_batchnorm` 是一个布尔值,表示是否在隐藏层之间使用批归一化。默认值为 False。
在 `forward` 方法中,定义了模型的前向传播过程:
1. 首先通过循环创建多个线性层,并将它们添加到 `layers` 列表中。每个线性层的输入维度为 `input_dim`,输出维度为当前隐藏层的维度。
2. 如果设置了批归一化(`use_batchnorm=True`)且当前隐藏层的维度不为 1,将批归一化层添加到 `layers` 列表中。
3. 将 ReLU 激活函数添加到 `layers` 列表中,除非当前隐藏层的维度为 1。
4. 如果设置了 dropout(`dropout_p` 不为 None)且当前隐藏层的维度不为 1,将 dropout 层添加到 `layers` 列表中。
5. 将当前隐藏层的维度更新为下一层的维度。
6. 将 `layers` 列表中的层组合成一个序列,存储在 `fc_layers` 中。
在 `forward` 方法的最后,输入通过 `fc_layers` 进行前向传播,得到输出。
这段代码实现了一个多层感知机模型,通过堆叠多个线性层和可选的批归一化、ReLU 激活函数和 dropout 层,对输入进行特征提取和非线性变换。
相关推荐
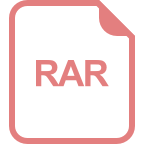
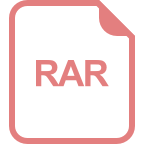









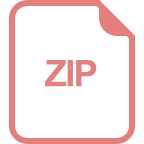
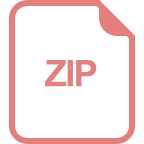