解释utilize the LSTM model in torch.nn
时间: 2024-01-07 14:46:24 浏览: 39
LSTM (Long Short-Term Memory) is a type of recurrent neural network (RNN) architecture that is designed to overcome the vanishing gradient problem in traditional RNNs. It is particularly useful for processing sequential data such as time-series, natural language, and speech data.
In PyTorch, the LSTM model is implemented in the torch.nn module. The LSTM class can be called with the required input and output dimensions, as well as the number of layers and hidden units in each layer. The forward method of the LSTM class takes the input sequence and returns the output sequence and the final hidden state of the LSTM.
Here is an example of utilizing the LSTM model in PyTorch:
```
import torch
import torch.nn as nn
# Define LSTM model
class LSTMModel(nn.Module):
def __init__(self, input_dim, hidden_dim, num_layers, output_dim):
super(LSTMModel, self).__init__()
self.hidden_dim = hidden_dim
self.num_layers = num_layers
self.lstm = nn.LSTM(input_dim, hidden_dim, num_layers, batch_first=True)
self.fc = nn.Linear(hidden_dim, output_dim)
def forward(self, x):
# Initialize hidden state and cell state
h0 = torch.zeros(self.num_layers, x.size(0), self.hidden_dim).requires_grad_()
c0 = torch.zeros(self.num_layers, x.size(0), self.hidden_dim).requires_grad_()
# Forward pass through LSTM layer
lstm_out, (h_n, c_n) = self.lstm(x, (h0.detach(), c0.detach()))
# Flatten LSTM output to feed into fully connected layer
lstm_out = lstm_out[:, -1, :]
# Forward pass through fully connected layer
out = self.fc(lstm_out)
return out
# Instantiate LSTM model
input_dim = 10
hidden_dim = 20
num_layers = 2
output_dim = 1
model = LSTMModel(input_dim, hidden_dim, num_layers, output_dim)
# Define loss function and optimizer
criterion = nn.MSELoss()
optimizer = torch.optim.Adam(model.parameters(), lr=0.01)
# Train LSTM model
for epoch in range(num_epochs):
# Forward pass
outputs = model(inputs)
loss = criterion(outputs, targets)
# Backward and optimize
optimizer.zero_grad()
loss.backward()
optimizer.step()
```
In this example, we define an LSTM model with an input dimension of 10, a hidden dimension of 20, 2 layers, and an output dimension of 1. We then instantiate the model and define the loss function and optimizer. Finally, we train the model using the forward method to calculate the output and the loss.backward() method to calculate the gradients and update the weights.
相关推荐
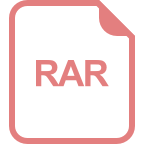
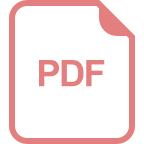















