colsample_bytree = 0.8 gammma=0.1 params = { 'eval_metric': 'rmse', 'max_depth': max_depth, 'learning_rate': learning_rate, 'n_estimators': n_estimators, 'gamma': gamma, 'min_child_weight': min_child_weight, 'subsample': subsample, 'colsample_bytree':colsample_bytree, 'n_jobs': -1, 'random_state': 42 }
时间: 2024-02-23 16:03:23 浏览: 51
在这段代码中,你定义了 XGBoost 模型的参数。其中,'colsample_bytree' 参数指定了每棵树在进行拟合时随机选择的特征占比,取值为 0.8;'gamma' 参数指定了每个叶子节点分裂所需的最小损失减少值,取值为 0.1。
同时,你还定义了其他的参数,例如最大深度、学习率、子采样率等等,这些参数都可以影响到模型的性能。
最后,你将所有的参数都存储在一个字典中,以便在调用 XGBoost 模型时使用,例如:
```
xgb.train(params, dtrain, num_boost_round=10, evals=[(dtest, "Test")], early_stopping_rounds=3)
```
这将使用上述定义的参数来训练 XGBoost 模型。
相关问题
params = { 'eval_metric': 'rmse', 'max_depth': max_depth, 'learning_rate': learning_rate, 'n_estimators': n_estimators, 'gamma': gamma, 'min_child_weight': min_child_weight, 'subsample': subsample, 'colsample_bytree':colsample_bytree, 'n_jobs': -1, 'random_state': 42 }
这段代码是在定义 XGBoost 模型的参数。其中,'colsample_bytree' 参数指定了每棵树在进行拟合时随机选择的特征占比。这个参数的取值范围是 (0, 1],表示每棵树在进行拟合时最多使用训练集中的多少特征。如果这个参数的值太小,可能会导致模型欠拟合;如果太大,可能会导致模型过拟合。
在定义参数时,你需要将 'colsample_bytree' 参数设置为一个浮点数值,例如:
```
colsample_bytree = 0.8
params = {
'eval_metric': 'rmse',
'max_depth': max_depth,
'learning_rate': learning_rate,
'n_estimators': n_estimators,
'gamma': gamma,
'min_child_weight': min_child_weight,
'subsample': subsample,
'colsample_bytree': colsample_bytree,
'n_jobs': -1,
'random_state': 42
}
```
这将使用 colsample_bytree 参数为 0.8 的 XGBoost 模型进行训练。
def xgb_cv(max_depth, learning_rate, n_estimators, gamma, min_child_weight, subsample, colsample_bytree): date_x = pd.read_csv('Train_data1.csv') # Well logging data date_x.rename(columns={"TC": 'label'}, inplace=True) date_x.drop('Depth', axis=1, inplace=True) date_x.drop('MSFL', axis=1, inplace=True) date_x.drop('CNL', axis=1, inplace=True) date_x.drop('AC', axis=1, inplace=True) date_x.drop('GR', axis=1, inplace=True) data = date_x.iloc[2:42, :] label = data.iloc[:, 1:2] data2 = data.iloc[:, :7] train_x, test_x, train_y, test_y = train_test_split(data2, label, test_size=0.5, random_state=0) xgb_train = xgb.DMatrix(train_x, label=train_y) xgb_test = xgb.DMatrix(test_x, label=test_y) params = { 'eval_metric': 'rmse', 'max_depth': int(max_depth), 'learning_rate': learning_rate, 'n_estimators': int(n_estimators), 'gamma': gamma, 'min_child_weight': int(min_child_weight), 'subsample': subsample, 'colsample_bytree': colsample_bytree, 'n_jobs': -1, 'random_state': 42 } # 进行交叉验证 cv_result = xgb.cv(params, xgb_train, num_boost_round=100, early_stopping_rounds=10, stratified=False) return -1.0 * cv_result['test-rmse-mean'].iloc[-1] # 定义参数范围 pbounds = {'max_depth': (3, 10), 'learning_rate': (0.01, 0.3), 'n_estimators': (50, 200), 'gamma': (0, 10), 'min_child_weight': (1, 10), 'subsample': (0.5, 1), 'colsample_bytree': (0.1, 1)} # 进行贝叶斯优化,找到最优超参数 optimizer = BayesianOptimization(f=xgb_cv, pbounds=pbounds, random_state=42) optimizer.maximize(init_points=5, n_iter=25) # 输出最优结果 print(optimizer.max) model = xgb.train(optimizer.max, xgb_train) model.save_model("model3.xgb") return optimizer.max
这个函数中包括了使用 xgboost 进行交叉验证、贝叶斯优化和训练模型的过程。在这个函数中,你需要传入 7 个参数,分别是 `max_depth`、`learning_rate`、`n_estimators`、`gamma`、`min_child_weight`、`subsample` 和 `colsample_bytree`。
这个函数首先读入训练数据,然后对数据进行预处理,包括删除某些列和分割数据集。接下来,它将使用 xgboost 提供的 `xgb.cv()` 函数进行交叉验证,并返回最优模型的 rmse 值。然后,它定义了超参数的范围,并使用贝叶斯优化算法寻找最优超参数。最后,它训练了一个 xgboost 模型,并将其保存到文件中。
你可以按照以下方式调用该函数,并传入所需的 7 个参数的值:
```
params1 = xgb_cv(max_depth=5, learning_rate=0.1, n_estimators=100, gamma=0.1, min_child_weight=1, subsample=0.8, colsample_bytree=0.8)
```
其中,你可以根据你的具体需求,设置这些参数的值,以得到最佳的 xgboost 模型。
阅读全文
相关推荐
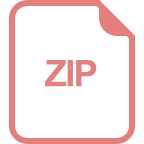
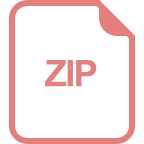
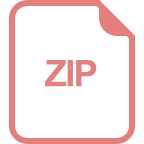


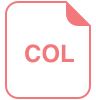
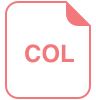
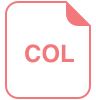
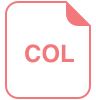
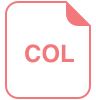
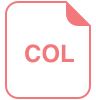
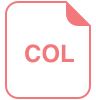


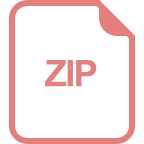