nn.parameter()
时间: 2023-08-21 10:00:45 浏览: 27
torch.nn.parameter()是PyTorch中的一个类,用于表示神经网络中的可学习参数。这些参数可以是权重、偏置等,它们会在训练过程中被不断更新以优化模型的性能。这个类提供了一些方法来管理和操作这些参数,例如获取参数的值、设置参数的值、计算参数的梯度等。在神经网络的训练过程中,使用这个类来管理参数是非常重要的。
相关问题
nn.Parameter
`nn.Parameter`是PyTorch中的一个类,它是`Tensor`的子类,用于表示模型参数。与普通的`Tensor`不同,`nn.Parameter`会自动被注册为模型的可训练参数,并且可以在模型中进行优化更新。
一般来说,在PyTorch中,模型的参数都会被定义为`nn.Parameter`对象,以便在反向传播时自动跟踪梯度,从而进行梯度下降更新。`nn.Parameter`的使用非常简单,只需要将一个`Tensor`用`nn.Parameter`进行包装即可。
例如,下面的代码定义了一个大小为(3, 4)的权重矩阵,并将其封装成`nn.Parameter`对象:
```
import torch.nn as nn
import torch
weight = torch.randn(3, 4)
weight_param = nn.Parameter(weight)
```
在模型的`__init__`函数中,通常会使用`nn.Parameter`封装所有的模型参数,例如:
```
class MyModel(nn.Module):
def __init__(self):
super(MyModel, self).__init__()
self.weight = nn.Parameter(torch.randn(3, 4))
self.bias = nn.Parameter(torch.zeros(3))
```
这样,在模型的前向计算中,就可以直接使用`self.weight`和`self.bias`这两个`nn.Parameter`对象了。
nn.parameter
`nn.parameter`是PyTorch中的一个类,用于表示模型参数。该类的实例可以被视为可训练的张量,因为它们在反向传播期间会自动计算梯度,并可以通过优化器进行更新。通常,我们使用`nn.parameter`类来定义神经网络的权重和偏差参数,例如:
```python
import torch.nn as nn
class MyNet(nn.Module):
def __init__(self):
super(MyNet, self).__init__()
self.weight = nn.parameter.Parameter(torch.randn(10, 5))
self.bias = nn.parameter.Parameter(torch.zeros(10))
def forward(self, x):
out = torch.matmul(x, self.weight) + self.bias
return out
```
在上面的代码中,`weight`和`bias`都是`nn.parameter`类的实例,表示神经网络的权重和偏差参数。这些参数可以使用`torch.randn`和`torch.zeros`函数进行初始化。在`forward`方法中,我们使用这些参数来计算模型的输出。
相关推荐
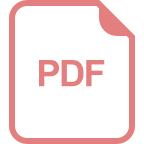
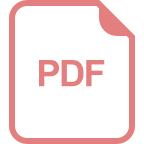




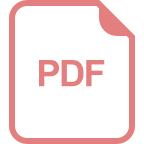
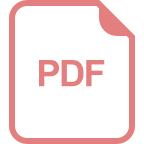
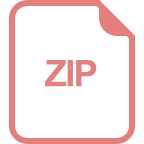
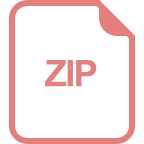
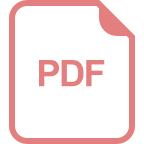
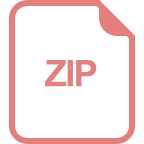
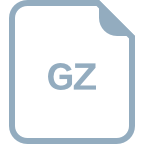
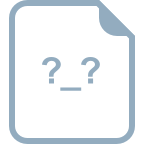
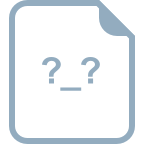