AttributeError: module 'tensorflow' has no attribute 'py_func'
时间: 2024-07-18 14:01:27 浏览: 120
`AttributeError: module 'tensorflow' has no attribute 'py_func'` 这是一个Python错误,通常出现在尝试使用TensorFlow库中的`py_func`函数时。`py_func`曾经是早期版本TensorFlow的一个特性,用于将Python函数作为操作插入计算图。然而,在后来的更新中,这个功能已经被移除或替换。
如果你遇到这个错误,可能是因为你在使用的TensorFlow版本中已经不包含`py_func`了,或者你直接导入的是某个旧版本的代码片段。解决这个问题需要检查你的TensorFlow安装是否是最新的,并确认所引用的代码是否适用于当前版本。
你可以通过以下步骤来处理:
1. 检查TensorFlow版本:`import tensorflow as tf; print(tf.__version__)`
2. 确保没有过时的依赖:查看你的项目文件,确保所有引用`py_func`的地方都已经正确修改或删除。
3. 如果确实需要类似的功能,可以考虑使用其他替代方法,比如函数包装、`tf.py_function`等现代API。
如果仍然有问题,可以提供更多信息以便找到更具体的解决方案。
相关问题
AttributeError: module 'tensorrt' has no attribute 'Logger'
引用[1]:根据你提供的错误信息,出现了一个AttributeError: module 'logging' has no attribute 'config'的错误。这个错误通常是由于项目中的某个模块或文件覆盖了系统默认的logging模块导致的。[2]你可以检查一下你的项目中是否有一个名为logging的模块或文件,如果有的话,可以尝试将其重命名或删除,以恢复系统默认的logging模块。
引用[3]:另外,你还提到了一个问题描述为"TypeError: func_impl() got an unexpected keyword argument 'flags'"的错误。根据错误信息,这个错误发生在文件"/test/SDpytrirton/TensorRT-release-8.6/demo/Diffusion/utilities.py"的第213行。这个错误通常是由于在调用函数func_impl()时传入了一个未知的关键字参数'flags'导致的。你可以检查一下代码中调用func_impl()函数的地方,确保传入的参数是正确的,并且没有多余的关键字参数。
最后,你提到了一个问题"AttributeError: module 'tensorrt' has no attribute 'Logger'"。根据错误信息,这个错误发生在tensorrt模块中,具体是在尝试访问'tensorrt.Logger'属性时出现了错误。这个错误通常是由于tensorrt模块中确实没有名为'Logger'的属性导致的。你可以检查一下你的代码中是否正确导入了tensorrt模块,并且确保你在访问属性时使用了正确的属性名。
希望以上信息对你有帮助。如果还有其他问题,请随时提问。
return ast.unparse(node).strip() AttributeError: module 'ast' has no attribute 'unparse'
很抱歉,我之前的回答中使用了 `ast.unparse()`,但实际上在 Python 3.9 之前的版本中没有该方法。以下是一个修正后的代码示例,可以获取函数调用栈中的函数体和类方法体,并将它们以字符串形式返回:
```python
import ast
import os
import inspect
class FunctionVisitor(ast.NodeVisitor):
def __init__(self, target_file, target_line, target_function):
self.target_file = target_file
self.target_line = target_line
self.target_function = target_function
self.function_calls = {}
def visit_FunctionDef(self, node):
current_file = os.path.basename(self.target_file)
if node.name == self.target_function and current_file == self.target_file:
self.function_calls[(node.name, node.lineno)] = inspect.getsource(node)
def visit_Call(self, node):
if isinstance(node.func, ast.Attribute):
attribute_name = node.func.attr
attribute_value = node.func.value
if isinstance(attribute_value, ast.Name):
attribute_file = self.target_file
attribute_line = node.lineno
self.function_calls[(attribute_name, attribute_line)] = inspect.getsource(node)
self.generic_visit(node)
def analyze_function_calls(directory, target_file, target_line, target_function):
module_files = get_module_files(directory)
function_calls = {}
for file in module_files:
with open(file, 'r', encoding='utf-8') as f:
tree = ast.parse(f.read())
function_visitor = FunctionVisitor(target_file, target_line, target_function)
function_visitor.visit(tree)
function_calls.update(function_visitor.function_calls)
return function_calls
def get_module_files(directory):
module_files = []
for root, dirs, files in os.walk(directory):
for file in files:
if file.endswith('.py'):
module_files.append(os.path.join(root, file))
return module_files
directory = 'your_directory' # 修改为你要分析的目录
target_file = 'your_file.py' # 修改为函数所在的文件名
target_line = 10 # 修改为函数所在的行号
target_function = 'your_function' # 修改为你要分析的函数名
function_calls = analyze_function_calls(directory, target_file, target_line, target_function)
for (name, line), source_code in function_calls.items():
print(f"Function or Attribute: {name}")
print(f"Line: {line}")
print(f"Source Code:\n{source_code}\n")
```
在修正后的代码中,我们使用了 `inspect.getsource()` 方法来获取函数体和类方法体的源代码,并将它们存储在字典 `function_calls` 中。字典的键是一个元组,包含函数或属性的名称和行号。
请将代码中的 `your_directory`、`your_file.py`、`target_line` 和 `your_function` 分别替换为你要分析的目录、函数所在的文件名、函数所在的行号和函数名。运行代码后,它会返回一个字典,其中包含函数和属性调用信息及其对应的源代码,并打印出这些信息。
这只是一个简单的示例代码,可能无法处理特殊情况或复杂的代码结构。在实际使用时,请根据你的具体需求进行修改和扩展,并且仍然建议仔细测试和验证结果。
相关推荐
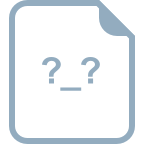
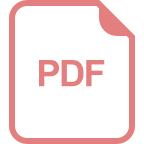














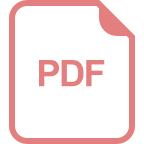