通过pytorch中在unet网络中定义一个可以修改类别权重的代码
时间: 2024-01-04 20:04:36 浏览: 34
下面是一个示例代码,展示如何在PyTorch中在UNet网络中定义一个可以修改类别权重的代码:
```python
import torch
import torch.nn as nn
class UNet(nn.Module):
def __init__(self, n_channels, n_classes, bilinear=True, class_weights=None):
super(UNet, self).__init__()
self.n_channels = n_channels
self.n_classes = n_classes
self.bilinear = bilinear
self.class_weights = class_weights
self.inc = DoubleConv(n_channels, 64)
self.down1 = Down(64, 128)
self.down2 = Down(128, 256)
self.down3 = Down(256, 512)
factor = 2 if bilinear else 1
self.down4 = Down(512, 1024 // factor)
self.up1 = Up(1024, 512 // factor, bilinear)
self.up2 = Up(512, 256 // factor, bilinear)
self.up3 = Up(256, 128 // factor, bilinear)
self.up4 = Up(128, 64, bilinear)
self.outc = OutConv(64, n_classes)
def forward(self, x):
x1 = self.inc(x)
x2 = self.down1(x1)
x3 = self.down2(x2)
x4 = self.down3(x3)
x5 = self.down4(x4)
x = self.up1(x5, x4)
x = self.up2(x, x3)
x = self.up3(x, x2)
x = self.up4(x, x1)
logits = self.outc(x)
if self.class_weights is not None:
# apply class weights
weight_tensor = torch.tensor(self.class_weights, dtype=torch.float32)
weight_tensor = weight_tensor.to(logits.device)
weight_tensor = weight_tensor.unsqueeze(0).unsqueeze(-1).unsqueeze(-1)
logits = logits * weight_tensor
return logits
```
在这个示例代码中,我们使用了PyTorch中的nn.Module类来定义了一个UNet网络。我们还定义了一个可选的类别权重参数class_weights,它可以是一个列表,其中包含每个类别的权重。
在前向传递方法中,如果class_weights参数不为None,则我们会将权重应用于输出的逻辑张量。我们首先将权重列表转换为张量,然后将其移动到模型当前设备上。最后,我们将权重张量与逻辑张量相乘,以将权重应用于每个类别的预测。
相关推荐
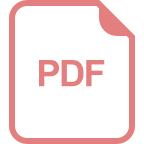
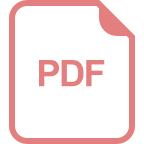
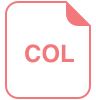













