导入F.normalize
时间: 2023-09-05 09:12:54 浏览: 49
在PyTorch中,`F.normalize`是一个函数,可以用于将张量规范化为单位范数(即L2范数为1)。它可以在神经网络中用于归一化特征向量,以便更好地进行训练。要使用`F.normalize`,需要先导入`torch.nn.functional`模块,代码如下:
```python
import torch.nn.functional as F
x = torch.randn(2, 3)
x_norm = F.normalize(x, p=2, dim=1)
```
在这个例子中,我们使用`F.normalize`将一个大小为`(2, 3)`的张量`x`在第1个维度上进行L2范数归一化,并赋值给`x_norm`。`p`参数指定了使用哪种范数(默认为L2范数),`dim`参数指定在哪个维度上进行归一化。
相关问题
请解释一下import matplotlib.pyplot as plt import numpy as np import torch import torch.utils.data.dataloader as Loader import torchvision import torchvision.transforms as transforms import torch.nn as nn import torch.nn.functional as F import torch.optim as optim from torch.utils.tensorboard import SummaryWriter BATCH SIZE = 4 transform - transforms.Compose( [transforms. ToTensor0, transforms.Normalize((0.5,), (0.5.)) trainset = torchvision.datasets.FashionMNIST(' /data', download True, train= True, transform=transform) testset = torchvision.datasets.FashionMNIST(' /data', download= True, train=False, transform= transform) trainloader = Loader.DataLoader(trainset, batch size= BATCH SIZE, shuffle True)testloader = Loader.Datal oader(testset, batch size=BATCH SIZE, shuffle- False)classes s ('T- shirt/top', 'Trouser', 'Pullover', 'Dress', 'Coat', 'Sandal', 'Shirt', 'Sneaker', 'Bag', 'Ankle Boot') class Net(nn.Module);: def_ init (self): super(Net, self). init 0这段代码
这段代码是一个用于训练和测试Fashion MNIST数据集的神经网络模型。具体来说,代码首先导入了一些需要用到的Python库,如matplotlib、numpy、torch等。然后定义了一些超参数,如BATCH SIZE,以及数据集的变换transform。
接着,代码使用torchvision库中的FashionMNIST数据集,并将其分成训练集和测试集。然后利用torch.utils.data.dataloader库中的DataLoader函数来将数据集划分成相应的batch,并进行打乱顺序操作。
最后,代码定义了一个名为Net的神经网络模型,并继承了nn.Module类。在Net类中,定义了该模型的结构,包括卷积层、池化层、全连接层等,并应用了激活函数relu和softmax。整个模型的训练过程将在接下来的代码中完成。
给下面这段代码每行注释import os import json import torch from PIL import Image from torchvision import transforms from model import resnet34 def main(): device = torch.device("cuda:0" if torch.cuda.is_available() else "cpu") data_transform = transforms.Compose( [transforms.Resize(256), transforms.CenterCrop(224), transforms.ToTensor(), transforms.Normalize([0.485, 0.456, 0.406], [0.229, 0.224, 0.225])]) # load image # 指向需要遍历预测的图像文件夹 imgs_root = "../dataset/val" assert os.path.exists(imgs_root), f"file: '{imgs_root}' dose not exist." # 读取指定文件夹下所有jpg图像路径 img_path_list = [os.path.join(imgs_root, i) for i in os.listdir(imgs_root) if i.endswith(".jpg")] # read class_indict json_path = './class_indices.json' assert os.path.exists(json_path), f"file: '{json_path}' dose not exist." json_file = open(json_path, "r") class_indict = json.load(json_file) # create model model = resnet34(num_classes=16).to(device) # load model weights weights_path = "./newresNet34.pth" assert os.path.exists(weights_path), f"file: '{weights_path}' dose not exist." model.load_state_dict(torch.load(weights_path, map_location=device)) # prediction model.eval() batch_size = 8 # 每次预测时将多少张图片打包成一个batch with torch.no_grad(): for ids in range(0, len(img_path_list) // batch_size): img_list = [] for img_path in img_path_list[ids * batch_size: (ids + 1) * batch_size]: assert os.path.exists(img_path), f"file: '{img_path}' dose not exist." img = Image.open(img_path) img = data_transform(img) img_list.append(img) # batch img # 将img_list列表中的所有图像打包成一个batch batch_img = torch.stack(img_list, dim=0) # predict class output = model(batch_img.to(device)).cpu() predict = torch.softmax(output, dim=1) probs, classes = torch.max(predict, dim=1) for idx, (pro, cla) in enumerate(zip(probs, classes)): print("image: {} class: {} prob: {:.3}".format(img_path_list[ids * batch_size + idx], class_indict[str(cla.numpy())], pro.numpy())) if __name__ == '__main__': main()
1. import os:导入操作系统模块
2. import json:导入处理json格式的模块
3. import torch:导入深度学习框架PyTorch模块
4. from PIL import Image:从PIL模块中导入Image模块,用于图像处理
5. from torchvision import transforms:从torchvision模块中导入transforms模块,用于数据预处理
6. from model import resnet34:从自定义模块model中导入resnet34模型
7. def main(): 定义一个名为main的函数
8. device = torch.device("cuda:0" if torch.cuda.is_available() else "cpu"):使用GPU加速计算,如果GPU可用,就使用GPU,否则使用CPU
9. data_transform = transforms.Compose([...]):定义一个数据预处理的组合操作,对图像进行缩放、中心裁剪、转换为张量并标准化
10. transforms.Resize(256):将图像缩放至256*256大小
11. transforms.CenterCrop(224):对缩放后的图像从中心裁剪出224*224大小的图像
12. transforms.ToTensor():将图像转换为张量
13. transforms.Normalize([0.485, 0.456, 0.406], [0.229, 0.224, 0.225]):将张量标准化,均值为0.485、0.456、0.406,标准差为0.229、0.224、0.225
相关推荐
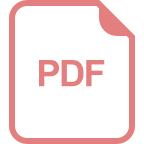
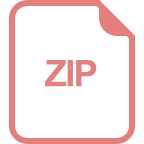












