pytorch版lstm预测多输入多输出
时间: 2023-05-13 11:00:37 浏览: 473
PyTorch是一种支持自动求导的深度学习库,是实现神经网络的重要工具之一。在LSTM预测多输入多输出的场景下,需要对网络进行一定的修改和参数设置。
首先,在定义网络结构时,需要设置合适的输入层、隐藏层和输出层。对于多输入多输出的场景,需要设置多个输入层和多个输出层,每个输入层传递不同的输入数据,每个输出层输出不同的预测结果。
其次,在训练网络时,需要设置合适的损失函数和优化器。对于多输出的场景,可以使用多个损失函数,每个损失函数对应一个输出,同时将多个损失函数加权求和,得到整体的损失函数。在优化器的选择上,可以考虑使用Adam等优化器,以加速收敛和提高模型的效果。
最后,在对模型进行测试和预测时,需要将多个输入数据传递给模型,同时对多个输出结果进行整合和解析。可以使用numpy等数学库对多个输出结果进行加权求和或者其他操作,得到最终的预测结果。
总之,PyTorch可以支持LSTM预测多输入多输出的场景,需要合适的网络结构、损失函数、优化器和预测策略来实现。掌握相关的技术和方法,可以提高模型的准确度和效率。
相关问题
用pytorch写lstm预测多变量时间序列
首先,你需要准备好你的数据。对于多变量时间序列数据,你需要将每个变量作为一个特征,并将它们放在一起形成一个二维数组 x,每行代表一个时间步骤。另外,你需要一个一维数组 y,代表每个时间步骤的目标值。这些数据需要被划分为训练集和测试集。
接下来,你需要构建一个 LSTM 模型。你可以使用 PyTorch 的 `nn.LSTM` 模块来构建 LSTM 层。然后,你可以使用 `nn.Linear` 模块构建一个全连接层,将 LSTM 层的输出映射到一个具有多个特征的输出空间。
下面是一个简单的 LSTM 模型示例:
```
import torch.nn as nn
class LSTM(nn.Module):
def __init__(self, input_size, hidden_size, num_layers, output_size):
super(LSTM, self).__init__()
self.hidden_size = hidden_size
self.num_layers = num_layers
self.lstm = nn.LSTM(input_size, hidden_size, num_layers)
self.fc = nn.Linear(hidden_size, output_size)
def forward(self, x):
h0 = torch.zeros(self.num_layers, x.size(1), self.hidden_size)
c0 = torch.zeros(self.num_layers, x.size(1), self.hidden_size)
out, _ = self.lstm(x, (h0, c0))
out = self.fc(out[-1, :, :])
return out
```
在这个模型中,我们使用 `nn.LSTM` 构建 LSTM 层,输入大小为 `input_size`,隐藏大小为 `hidden_size`,层数为 `num_layers`。然后我们使用 `nn.Linear` 构建一个全连接层,将 LSTM 输出映射到具有 `output_size` 个特征的输出空间。
接下来,你需要定义损失函数和优化器。对于回归问题,我们可以使用均方误差(MSE)作为损失函数,并使用随机梯度下降(SGD)或者 Adam 作为优化器。
```
criterion = nn.MSELoss()
optimizer = torch.optim.Adam(model.parameters(), lr=learning_rate)
```
然后,你可以开始训练模型。在每个训练迭代中,你需要将输入数据 x 传递到模型中,得到预测 y_pred。然后计算损失值并进行反向传播,更新模型参数。
```
for epoch in range(num_epochs):
# Forward pass
outputs = model(x_train)
loss = criterion(outputs, y_train)
# Backward and optimize
optimizer.zero_grad()
loss.backward()
optimizer.step()
if (epoch+1) % 100 == 0:
print('Epoch [{}/{}], Loss: {:.4f}'.format(epoch+1, num_epochs, loss.item()))
```
最后,你可以使用训练好的模型进行预测。你需要将测试数据 x_test 传递给模型,得到预测值 y_pred。然后你可以计算预测值与真实值之间的误差,并可视化它们的比较。
```
with torch.no_grad():
y_pred = model(x_test)
loss = criterion(y_pred, y_test)
print('Test Loss: {:.4f}'.format(loss.item()))
plt.plot(y_test.numpy(), label='True')
plt.plot(y_pred.numpy(), label='Predicted')
plt.legend()
plt.show()
```
完整的代码示例:
```
import torch
import torch.nn as nn
import numpy as np
import matplotlib.pyplot as plt
# Prepare data
# Here we generate a simple time series data with 2 features
def generate_data(num_data):
x = np.random.randn(num_data, 2)
y = np.zeros((num_data, 1))
for i in range(2, num_data):
y[i] = 0.5 * y[i-1] + 0.2 * y[i-2] + 0.1 * x[i-2, 0] + 0.3 * x[i-1, 1] + 0.4
return x, y
x_train, y_train = generate_data(100)
x_test, y_test = generate_data(50)
# Define model
class LSTM(nn.Module):
def __init__(self, input_size, hidden_size, num_layers, output_size):
super(LSTM, self).__init__()
self.hidden_size = hidden_size
self.num_layers = num_layers
self.lstm = nn.LSTM(input_size, hidden_size, num_layers)
self.fc = nn.Linear(hidden_size, output_size)
def forward(self, x):
h0 = torch.zeros(self.num_layers, x.size(1), self.hidden_size)
c0 = torch.zeros(self.num_layers, x.size(1), self.hidden_size)
out, _ = self.lstm(x, (h0, c0))
out = self.fc(out[-1, :, :])
return out
model = LSTM(input_size=2, hidden_size=16, num_layers=2, output_size=1)
# Define loss and optimizer
criterion = nn.MSELoss()
optimizer = torch.optim.Adam(model.parameters(), lr=0.01)
# Train the model
num_epochs = 1000
for epoch in range(num_epochs):
# Convert numpy arrays to torch tensors
inputs = torch.from_numpy(x_train).float()
targets = torch.from_numpy(y_train).float()
# Forward pass
outputs = model(inputs)
loss = criterion(outputs, targets)
# Backward and optimize
optimizer.zero_grad()
loss.backward()
optimizer.step()
if (epoch+1) % 100 == 0:
print('Epoch [{}/{}], Loss: {:.4f}'.format(epoch+1, num_epochs, loss.item()))
# Test the model
with torch.no_grad():
x_test = torch.from_numpy(x_test).float()
y_test = torch.from_numpy(y_test).float()
y_pred = model(x_test)
loss = criterion(y_pred, y_test)
print('Test Loss: {:.4f}'.format(loss.item()))
# Visualize the results
plt.plot(y_test.numpy(), label='True')
plt.plot(y_pred.numpy(), label='Predicted')
plt.legend()
plt.show()
```
这是一个简单的 LSTM 模型,你可以根据自己的需求进行修改和扩展。
pytorch 实现lstm 多变量多输出
### 回答1:
在PyTorch中实现LSTM多变量多输出模型需要进行以下步骤:
1. 数据预处理:对于多变量输入,将各自的特征归一化到相同的尺度,整合成一个特征向量,同时对于多输出,将列数据整合成一个矩阵,最后拆分成训练集和测试集。
2. 定义模型:使用PyTorch定义LSTM多变量多输出的模型,包括输入层,隐藏层和输出层,同时使用PyTorch提供的LSTM函数。
3. 定义loss函数和optimizer:定义损失函数和优化器,在训练过程中,通过优化器来调整模型的参数,使模型能够更好地拟合数据。
4. 训练模型:将训练集输入模型进行训练,并对模型进行调参,使模型在验证集上的表现更好,同时继续训练模型,直至训练收敛。
5. 预测结果:将测试集输入模型,得到模型输出,分别对多个输出进行解析,得到多个预测结果。
6. 评估模型:通过与真实的结果进行比较,计算误差和准确率,评估模型的性能,选择性能较优的模型。
通过以上步骤,便可以在PyTorch中实现LSTM多变量多输出模型。值得注意的是,在实践中需要根据具体的数据场景和需求进行合理的调参和优化,使得模型的表现更好。
### 回答2:
PyTorch 是一个流行的 Python 深度学习框架,常用于各种机器学习任务,包括回归分析和时间序列预测。LSTM 是一种递归神经网络,通常用于处理有序、时间相关的数据。多变量多输出模型是指在一次预测中,需要处理多个变量并输出多个值。
使用 PyTorch 实现 LSTM 多变量多输出的步骤如下:
1.准备数据
将要使用的数据准备好,包括独立变量和依赖变量,例如一个基于时间序列的房价预测模型,其中独立变量可能包括房屋面积、位置、建造年份等。
2.划分数据集
将数据集划分为训练集、验证集和测试集。训练集用于训练模型,验证集用于优化模型的参数和超参数,以防止过度拟合,测试集用于评估模型的性能。
3.归一化数据
使用标准化或归一化方法将数据缩放到相似的范围内,以便在训练过程中更好地收敛,并且避免大幅度变化对模型的影响。
4.创建 LSTM 模型
使用 PyTorch 建立 LSTM 模型。在该模型中,输入数据可能经过一系列的多变量特征处理,然后通过 LSTM 层进行处理,接着输出层输出多个值。
5.训练模型
使用训练集训练模型,并通过验证集调整模型的参数和超参数,以避免过拟合。
6.评估模型
使用测试集评估训练好的模型的性能,在评估指标方面,常用的方法包括均方误差 (MSE) 和平均绝对误差 (MAE)。
在 PyTorch 中实现 LSTM 多变量多输出模型可参考包括多个模块的链式结构,包括多个全连接层和 LSTM 层。可以使用 Sequential() 构建一个序列模型,并使用 LSTM 的 PyTorch 实现进行训练。在每次迭代过程中计算并优化代价函数,直到达到预定的训练时长或满足特定的模型性能要求。
总之,在 PyTorch 中实现 LSTM 多变量多输出模型可以使用标准化、准备数据并按顺序构建模型。训练和评估模型时,需要注意认真设置有关神经网络层及其输入维度的各个超级参数,例如时间步长、隐层节点数量等等。
### 回答3:
PyTorch 是一个基于 Python 的科学计算库,它支持动态计算图机制,并且拥有很好的灵活性和可扩展性。在使用 PyTorch 实现 LSTM 多变量多输出的过程中,我们需要了解以下几个方面的知识点:
1. LSTM(Long-Short Term Memory)
LSTM 是一种递归神经网络(RNN)的扩展,它可以处理时序数据、文本等序列型数据,并支持学习长时间记忆。LSTM 的一个重要特征是可以从过去的信息中学习提取特征,以便更好地预测未来。
2. 多变量多输出
多变量多输出问题是一种回归问题,需要预测多个连续变量的值。在 PyTorch 中,可以通过设置多输出的 LSTM 模型来实现这个问题。同时,需要注意数据的处理方式,需要将输入数据按照时间序列进行归一化处理,并拆分成多个输入变量和多个输出变量。
3. PyTorch 的 LSTM 模型实现
在 PyTorch 中,实现 LSTM 模型需要使用 torch.nn.LSTM 类,它接受以下参数:
- input_size:输入的特征维度;
- hidden_size:隐藏状态的维度;
- num_layers:LSTM 层数;
- batch_first:是否将 batch 放在第一维。
在实现多输出的 LSTM 模型时,需要在 LSTM 层后面添加全连接层,并设置输出的维度。代码实现如下:
``` python
import torch.nn as nn
class LSTM_Model(nn.Module):
def __init__(self, input_size, hidden_size, num_layers, output_size):
super(LSTM_Model, self).__init__()
self.hidden_size = hidden_size
self.num_layers = num_layers
self.lstm = nn.LSTM(input_size, hidden_size, num_layers, batch_first=True)
self.fc = nn.Linear(hidden_size, output_size)
def forward(self, x):
h0 = torch.zeros(self.num_layers, x.size(0), self.hidden_size).to(device)
c0 = torch.zeros(self.num_layers, x.size(0), self.hidden_size).to(device)
out, _ = self.lstm(x, (h0, c0))
out = self.fc(out[:, -1, :])
return out
```
4. 训练和预测
在训练时,需要定义损失函数和优化器,并迭代多次进行反向传播和参数更新。在预测时,需要将输入数据经过模型预测得到输出数据。同时,需要逆归一化处理得到真实的输出数据。代码实现如下:
``` python
model = LSTM_Model(input_size, hidden_size, num_layers, output_size).to(device)
criterion = nn.MSELoss()
optimizer = torch.optim.Adam(model.parameters(), lr=learning_rate)
for epoch in range(num_epochs):
for i, (inputs, targets) in enumerate(train_loader):
inputs = inputs.to(device)
targets = targets.to(device)
outputs = model(inputs)
loss = criterion(outputs, targets)
optimizer.zero_grad()
loss.backward()
optimizer.step()
for i, (inputs, targets) in enumerate(test_loader):
inputs = inputs.to(device)
targets = targets.to(device)
outputs = model(inputs)
outputs = outputs.tolist()
targets = targets.tolist()
predictions = scaler_y.inverse_transform(outputs)
actuals = scaler_y.inverse_transform(targets)
```
综上所述,可以通过 PyTorch 实现 LSTM 多变量多输出的问题,需要了解 LSTM 的基本原理和 PyTorch 的 LSTM 模型实现,同时还需要处理数据、定义损失函数和优化器、训练和预测模型。
相关推荐
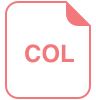
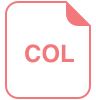
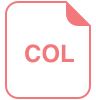









