解释下列代码# -*- coding: gbk-*- import numpy as np import pandas as pd header = ['user_id', 'item_id', 'rating', 'timestamp'] with open("u.data", "r") as file_object: df = pd.read_csv(file_object, sep='\t', names=header) print(df) n_users = df.user_id.unique().shape[0] n_items = df.item_id.unique().shape[0] print('Number of users = ' + str(n_users) + ' | Number of movies =' + str(n_items)) from sklearn.model_selection import train_test_split train_data, test_data = train_test_split(df, test_size=0.2, random_state=21) train_data_matrix = np.zeros((n_users, n_items)) for line in train_data.itertuples(): train_data_matrix[line[1] - 1, line[2] -1] = line[3] test_data_matrix = np.zeros((n_users, n_items)) for line in test_data.itertuples(): test_data_matrix[line[1] - 1, line[2] - 1] = line[3] print(train_data_matrix.shape) print(test_data_matrix.shape) from sklearn.metrics.pairwise import cosine_similarity item_similarity = cosine_similarity(train_data_matrix.T) print(u" 物品相似度矩阵 :", item_similarity.shape) print(u"物品相似度矩阵: ", item_similarity) def predict(ratings, similarity, type): # 基于物品相似度矩阵的 if type == 'item': pred = ratings.dot(similarity) / np.array([np.abs(similarity).sum(axis=1)]) print(u"预测值: ", pred.shape) return pred # 预测结果 item_prediction = predict(train_data_matrix, item_similarity, type='item') print(item_prediction) from sklearn.metrics import mean_squared_error from math import sqrt def rmse(prediction, ground_truth): prediction = prediction[ground_truth.nonzero()].flatten() ground_truth = ground_truth[ground_truth.nonzero()].flatten() return sqrt(mean_squared_error(prediction, ground_truth)) item_prediction = np.nan_to_num(item_prediction) print('Item-based CF RMSE: ' + str(rmse(item_prediction, test_data_matrix)))
时间: 2024-01-24 22:19:03 浏览: 163
这段代码主要是实现了基于物品相似度矩阵的推荐系统,具体流程如下:
1. 读取 u.data 数据集文件,用 pandas 库将其转换成 DataFrame 格式,并输出该数据集;
2. 计算该数据集中有多少个用户和多少个物品;
3. 将数据集分为训练集和测试集,其中训练集占 80%;
4. 构建训练集和测试集的评分矩阵,其中行表示用户,列表示物品,值表示评分;
5. 计算物品相似度矩阵,这里使用了 sklearn 库中的 cosine_similarity 方法;
6. 基于物品相似度矩阵,预测测试集中每个用户对每个物品的评分;
7. 计算预测结果与真实评分之间的均方根误差(RMSE),用于评估模型的性能。
需要注意的是,该代码只实现了基于物品相似度矩阵的推荐系统,还可以尝试其他的推荐算法,比如基于用户相似度矩阵的推荐系统。此外,还可以对模型进行参数调优,以获得更好的性能。
阅读全文
相关推荐
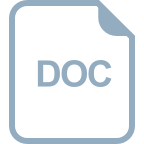
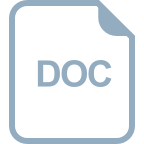
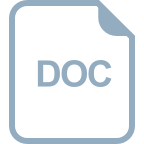
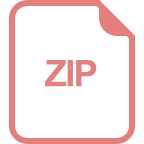
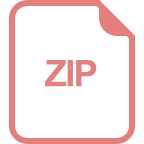
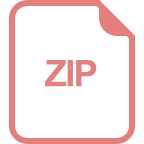
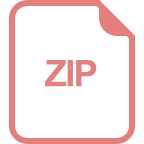
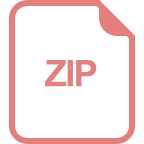
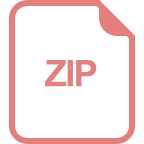
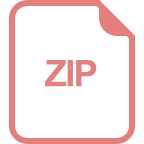
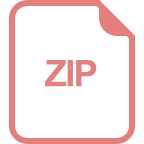
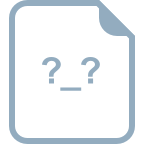
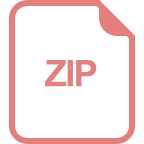
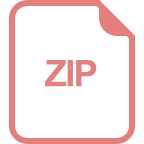
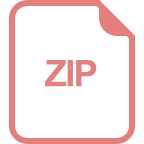
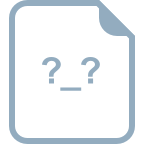
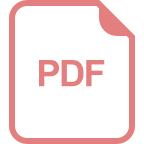
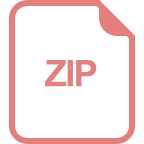
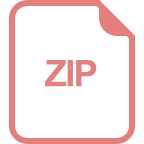