给定阈值,利用TF-IDF公式提取关键词。帮我生成简易代码
时间: 2024-12-13 20:20:54 浏览: 9
当然,以下是使用Python的scikit-learn库计算TF-IDF并提取关键词的一个简单例子。假设我们已经有了预处理过的文本数据和停用词列表。
```python
from sklearn.feature_extraction.text import TfidfVectorizer
import pandas as pd
# 假设data是你的文本数据列表
data = ['这是一条新闻', '这是第二条新闻', '第三条新闻是关于技术的']
# 停用词列表(可根据需要添加)
stop_words = set(['这', '是', '的'])
# 创建TfidfVectorizer实例
vectorizer = TfidfVectorizer(stop_words=stop_words)
# 计算TF-IDF矩阵
tfidf_matrix = vectorizer.fit_transform(data)
# 获取特征名称(即关键词)
features = vectorizer.get_feature_names()
# 设置阈值,例如大于0.5
threshold = 0.5
# 找出TF-IDF值大于阈值的关键词
important_features = [feature for feature, score in zip(features, tfidf_matrix.sum(axis=0)) if score > threshold]
print(f"重要的关键词: {important_features}")
相关问题
1、采集新浪新闻数据并把文本信息存储到.txt文件中; 2、利用现代汉语语料库进行汉语分词和词性自动标注,并进行文本的“词频统计”3、利用词典的词汇切分方法进行正向最大匹配、逆向最大匹配算法及双向匹配算法比较几种分词方法的优劣, 给定阀值,利用 TF-IDF 公式提取关键词
1. 采集新浪新闻数据并存储到.txt文件中:
要实现这个任务,首先你需要通过网络爬虫技术获取新浪新闻网站的数据。可以使用Python的requests库发送HTTP请求,然后BeautifulSoup或Scrapy等库解析HTML内容,找到包含新闻文本的部分。将抓取的内容保存到txt文件中,每条新闻作为一个独立的行。
```python
import requests
from bs4 import BeautifulSoup
url = "https://news.sina.com.cn/" # 新浪新闻首页URL
response = requests.get(url)
soup = BeautifulSoup(response.text, 'html.parser')
# 查找所有新闻标题和内容元素,这里假设它们有统一的class或id
news_elements = soup.find_all('div', class_='news-item') # 示例选择器
with open('sina_news.txt', 'w', encoding='utf-8') as f:
for element in news_elements:
title = element.find('h3').text
content = element.find('p').text
f.write(f"{title}\n{content}\n")
```
2. 汉语文本处理:
使用NLTK(自然语言工具包)或jieba等库对txt文件进行分词和词性标注,然后计算词频。Python中的jieba库非常适合汉字的分词:
```python
import jieba.posseg as pseg
from collections import Counter
def process_text(file_path):
with open(file_path, 'r', encoding='utf-8') as f:
text = f.read()
words_and_pos = pseg.cut(text)
word_freq = Counter(word.word for word, flag in words_and_pos)
return word_freq
word_freq_dict = process_text('sina_news.txt')
```
3. 分词方法比较:
对于正向最大匹配、逆向最大匹配和双向匹配(如HMM分词),你可以分别实现这三种算法,并对结果进行对比分析。例如,正向最大匹配从左往右寻找最优分词结果;逆向最大匹配则是从右往左;而双向匹配结合了两者,同时考虑前后的上下文。
- 正向最大匹配示例:
```python
def max_match_forward(text):
... (实现正向最大匹配算法)
```
- 双向匹配示例:
```python
def bidirectional_matching(text):
... (实现双向匹配算法)
```
然后比较三种方法的准确性和效率,以及对长词和罕见词的处理效果。
4. 利用TF-IDF提取关键词:
对词频统计的结果,可以应用TF-IDF(Term Frequency-Inverse Document Frequency)公式筛选出重要的关键词:
```python
from sklearn.feature_extraction.text import TfidfVectorizer
vectorizer = TfidfVectorizer(min_df=threshold) # 设置最低词频阈值
tfidf_matrix = vectorizer.fit_transform([str(word_freq_dict)])
feature_names = vectorizer.get_feature_names_out()
top_keywords = tfidf_matrix[0].sort_values(ascending=False)[:num_keywords] # num_keywords是你想要的关键词数量
keywords = [feature_names[i] for i in top_keywords.index]
```
阅读全文
相关推荐





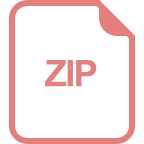
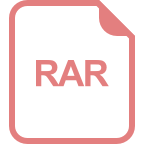

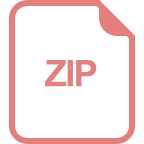








