opencv-python瑕疵检测
时间: 2024-01-20 10:02:16 浏览: 29
根据提供的引用内容,以下是使用OpenCV进行瑕疵检测的示例:
1. 使用Python进行缺陷检测和展示【引用1】:
```python
import cv2
# 读取图像
image = cv2.imread('image.jpg')
# 将图像转换为灰度图像
gray = cv2.cvtColor(image, cv2.COLOR_BGR2GRAY)
# 进行图像处理和缺陷检测
# ...
# 展示结果
cv2.imshow('Defect Detection', image)
cv2.waitKey(0)
cv2.destroyAllWindows()
```
2. 基于OpenCV的工件缺陷检测【引用2】:
```python
import cv2
# 读取图像
image = cv2.imread('image.jpg')
# 将图像转换为灰度图像
gray = cv2.cvtColor(image, cv2.COLOR_BGR2GRAY)
# 进行图像处理和缺陷检测
# ...
# 展示结果
cv2.imshow('Defect Detection', image)
cv2.waitKey(0)
cv2.destroyAllWindows()
```
请注意,上述示例仅展示了使用OpenCV进行瑕疵检测的基本框架,具体的图像处理和缺陷检测算法需要根据实际需求进行实现。
相关问题
用python和OpenCV检测瑕疵
好的,这是一个非常有趣的问题!那么你想要检测什么类型的瑕疵呢?例如,是检测产品表面的裂痕、凹陷或者其他类型的瑕疵吗?无论如何,我可以给你提供一些通用的步骤来进行基于OpenCV的瑕疵检测:
1. 图像预处理:使用OpenCV的函数进行图像去噪、灰度化、边缘检测、二值化等操作,以便更好地检测瑕疵。
2. 特征提取:使用OpenCV的函数提取图像的特征,例如纹理、形状、颜色等等。
3. 瑕疵识别:使用机器学习算法或深度学习模型对提取的特征进行分类和识别,以判断图像中是否存在瑕疵。
4. 瑕疵标记:将检测到的瑕疵标记出来,例如使用OpenCV的函数对瑕疵进行矩形框标定、颜色填充等操作。
总的来说,基于OpenCV进行瑕疵检测是一项非常复杂的任务,需要结合多种技术和算法。但是,如果你有一定的编程基础和计算机视觉的知识,那么应该可以掌握这项技术。
opencv对摄像头瑕疵检测
对于摄像头瑕疵检测,可以使用OpenCV中的图像处理函数来实现。一种常见的方法是使用背景差分(Background Subtraction)算法来检测移动物体或者瑕疵。
具体实现步骤如下:
1. 采集一段时间的背景图像,用于后续的背景差分;
2. 每一帧图像与背景图像做差分,得到移动物体或瑕疵的二值图像;
3. 对二值图像进行形态学操作,如腐蚀和膨胀,去除噪声点和填充断裂的区域,得到更加准确的瑕疵区域;
4. 可以根据瑕疵区域的位置和大小,来判断是否需要修复或更换摄像头。
下面是一个示例代码,使用背景差分算法来检测摄像头瑕疵:
``` python
import cv2
# 采集背景图像
cap = cv2.VideoCapture(0)
background = None
for i in range(30):
ret, frame = cap.read()
if not ret:
break
if background is None:
background = cv2.cvtColor(frame, cv2.COLOR_BGR2GRAY)
else:
background = cv2.addWeighted(background, 0.9, cv2.cvtColor(frame, cv2.COLOR_BGR2GRAY), 0.1, 0)
# 循环处理每一帧图像
while True:
ret, frame = cap.read()
if not ret:
break
# 背景差分
diff = cv2.absdiff(cv2.cvtColor(frame, cv2.COLOR_BGR2GRAY), background)
thresh = cv2.threshold(diff, 30, 255, cv2.THRESH_BINARY)[1]
# 形态学操作
kernel = cv2.getStructuringElement(cv2.MORPH_RECT, (5, 5))
thresh = cv2.erode(thresh, kernel, iterations=1)
thresh = cv2.dilate(thresh, kernel, iterations=1)
# 显示结果
cv2.imshow("frame", frame)
cv2.imshow("thresh", thresh)
if cv2.waitKey(1) == ord('q'):
break
cap.release()
cv2.destroyAllWindows()
```
在上述示例代码中,我们首先采集一段时间的背景图像,并使用其作为后续背景差分的参考。然后循环读取每一帧图像,与背景图像做差分,得到移动物体或瑕疵的二值图像。接着对二值图像进行形态学操作,去除噪声点和填充断裂的区域,得到更加准确的瑕疵区域。最后显示原图像和瑕疵二值图像,按下“q”键退出程序。
相关推荐
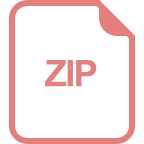
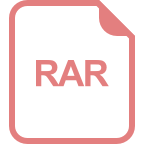











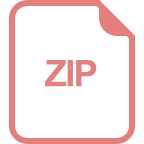