如何使用Python实现基于直方图和Hash算法的图像检索系统,并介绍如何调整图像对比度?请提供相应的代码示例。
时间: 2024-11-05 11:19:27 浏览: 24
为了创建一个基于直方图和Hash算法的图像检索系统,首先需要了解不同算法的工作原理和应用场景。直方图对比度侧重于图像的整体亮度分布,而Hash算法如均值Hash、差值Hash和感知Hash则更关注图像的局部特征和视觉相似性。在Python中,我们可以利用图像处理库如OpenCV和Pillow来提取图像特征,并实现算法。
参考资源链接:[Python实现图像对比度检索:直方图、Hash算法](https://wenku.csdn.net/doc/h4sd9s7jyg?spm=1055.2569.3001.10343)
代码实现可以分为几个步骤:
1. **读取和预处理图像**:使用Pillow库读取图像,并根据需要调整对比度。对比度调整可以通过修改图像的亮度和对比度参数来实现,公式为 `newpixel = alpha * oldpixel + beta`,其中 `alpha` 是对比度系数,`beta` 是亮度系数。
```python
from PIL import Image, ImageEnhance
def adjust_contrast(image, alpha, beta):
enhancer = ImageEnhance.Contrast(image)
image = enhancer.enhance(alpha)
return Image.blend(image, image.point(lambda x: x*alpha + beta), 0.5)
```
2. **计算直方图**:利用OpenCV库计算调整对比度后的图像直方图。
```python
import cv2
import numpy as np
def calculate_histogram(image):
image = np.array(image, dtype=np.float32) / 255.0
hist = cv2.calcHist([image], [0], None, [256], [0, 1])
cv2.normalize(hist, hist)
return hist.flatten()
```
3. **实现Hash算法**:分别实现均值Hash、差值Hash和感知Hash算法,并计算图像的哈希值。
```python
# 示例:均值Hash算法实现
def hash_mean(image):
resized = image.resize((8, 8), Image.ANTIALIAS)
avg = np.mean(resized, axis=(0, 1))
quantized = (avg/255).astype(np.uint8)
hash_str = ''.join(['1' if pixel > avg else '0' for pixel in quantized])
return hash_str
```
4. **相似度计算**:实现一个相似度计算函数,可以是汉明距离或其他距离度量方法。
```python
def hamming_distance(hash1, hash2):
return sum(c1 != c2 for c1, c2 in zip(hash1, hash2))
```
5. **检索和比较图像**:对一个图像库中的所有图像应用上述步骤,并通过相似度计算找出与目标图像最相似的图像。
```python
# 示例:检索函数框架
def search_image(target_image_path, image_folder, algorithm):
target_image = Image.open(target_image_path)
target_image = adjust_contrast(target_image, alpha=1.2, beta=20)
target_histogram = calculate_histogram(target_image)
target_hash = algorithm(target_image) # 调用具体算法函数
# 假设image_list为文件夹中所有图像路径的列表
for image_path in image_list:
image = Image.open(image_path)
image = adjust_contrast(image, alpha=1.2, beta=20)
histogram = calculate_histogram(image)
hash_str = algorithm(image)
distance = hamming_distance(target_hash, hash_str)
# 根据距离排序并找到最小距离的图像
# ...
return sorted_images
```
在这个例子中,我们通过调整对比度参数 `alpha` 和 `beta` 来改善图像的对比度,然后使用直方图和Hash算法来计算图像特征,并通过相似度计算来检索最相似的图像。这个框架可以根据实际需要进行扩展和优化。
对于课程设计或者项目开发,上述代码提供了一个很好的起点。通过实际操作这些代码,学生和开发者可以深入理解图像检索的原理和实现方法,并学会如何在不同的场景下选择和应用不同的图像处理技术。
参考资源链接:[Python实现图像对比度检索:直方图、Hash算法](https://wenku.csdn.net/doc/h4sd9s7jyg?spm=1055.2569.3001.10343)
阅读全文
相关推荐
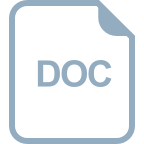
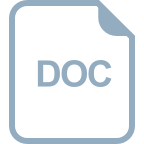
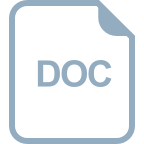

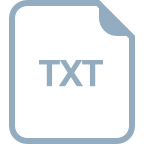
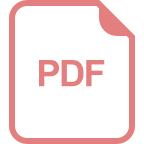
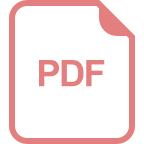
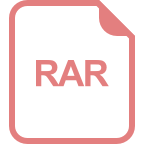
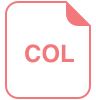
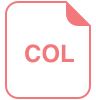
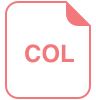
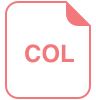
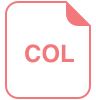
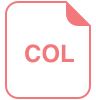
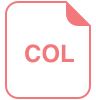
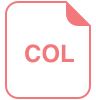
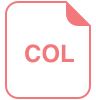
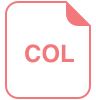
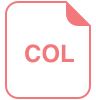