基于yolov8的垃圾识别
时间: 2023-12-11 08:33:00 浏览: 37
基于YOLOv8的垃圾识别系统可以用于检测和定位车辆垃圾、垃圾桶和垃圾溢出等目标。该系统使用深度学习算法实现目标检测,支持图片、视频等格式的结果可视化和结果导出。此外,该系统还支持训练模型的导入和初始化,检测置信度分数和后处理IOU阈值的调整,图像和视频的上传、检测、可视化结果展示和检测结果导出,摄像头的图像输入、检测和可视化结果展示,已检测目标个数和列表、位置信息,前向推理用时等功能。该系统使用YOLOv8目标检测算法训练数据集,使用Pysdie6库来搭建前端页面展示系统。
由于时间有限,引用中提到的垃圾识别模型训练数据集量比较小,仅限于瓶子、报纸、电池、剩饭和碎瓷片等四类垃圾。如果有兴趣,可以使用更大的数据集进行训练。
以下是基于YOLOv8的垃圾识别系统的Python代码示例:
```python
# 导入必要的库
import cv2
import numpy as np
import time
import os
# 加载YOLOv8模型
net = cv2.dnn.readNet("yolov8.weights", "yolov8.cfg")
# 加载类别标签
classes = []
with open("coco.names", "r") as f:
classes = [line.strip() for line in f.readlines()]
# 设置随机颜色
colors = np.random.uniform(0, 255, size=(len(classes), 3))
# 加载图像
img = cv2.imread("test.jpg")
# 获取图像尺寸
height, width, channels = img.shape
# 构建输入图像的blob
blob = cv2.dnn.blobFromImage(img, 1/255.0, (416, 416), swapRB=True, crop=False)
# 设置输入blob到网络中
net.setInput(blob)
# 进行前向推理
start = time.time()
outs = net.forward(net.getUnconnectedOutLayersNames())
end = time.time()
# 输出前向推理用时
print("Inference time: {:.5f} seconds".format(end - start))
# 初始化检测结果列表
class_ids = []
confidences = []
boxes = []
# 遍历每个输出层
for out in outs:
# 遍历每个检测框
for detection in out:
# 获取类别置信度
scores = detection[5:]
class_id = np.argmax(scores)
confidence = scores[class_id]
# 过滤掉低置信度的检测结果
if confidence > 0.5:
# 获取检测框的坐标
center_x = int(detection[0] * width)
center_y = int(detection[1] * height)
w = int(detection[2] * width)
h = int(detection[3] * height)
# 计算检测框的左上角坐标
x = int(center_x - w / 2)
y = int(center_y - h / 2)
# 添加检测结果到列表中
class_ids.append(class_id)
confidences.append(float(confidence))
boxes.append([x, y, w, h])
# 进行非最大抑制
indices = cv2.dnn.NMSBoxes(boxes, confidences, 0.5, 0.4)
# 遍历每个检测结果
for i in indices:
i = i[0]
box = boxes[i]
x = box[0]
y = box[1]
w = box[2]
h = box[3]
# 绘制检测框和类别标签
color = colors[class_ids[i]]
cv2.rectangle(img, (x, y), (x + w, y + h), color, 2)
text = "{}: {:.4f}".format(classes[class_ids[i]], confidences[i])
cv2.putText(img, text, (x, y - 5), cv2.FONT_HERSHEY_SIMPLEX, 0.5, color, 2)
# 显示检测结果
cv2.imshow("Image", img)
cv2.waitKey(0)
cv2.destroyAllWindows()
```
相关推荐
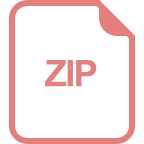
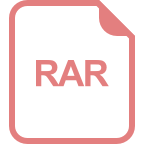
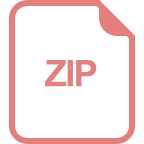














