xx = torch.tensor(X[start:end], dtype=torch.float, requires_grad=True) TypeError: can't convert np.ndarray of type numpy.object_. The only supported types are: float64, float32, float16, complex64, complex128, int64, int32, int16, int8, uint8, and bool
时间: 2024-04-16 19:26:26 浏览: 28
这个错误表明在创建 `xx` 张量时,输入的 `X[start:end]` 是一个 NumPy 数组(`np.ndarray`),并且数组中包含了不支持转换的数据类型 `numpy.object_`。在使用 `torch.tensor` 创建张量时,仅支持以下数据类型:`float64`、`float32`、`float16`、`complex64`、`complex128`、`int64`、`int32`、`int16`、`int8`、`uint8` 和 `bool`。
为了解决这个问题,您可以确保 `X[start:end]` 是一个支持的数据类型的 NumPy 数组。您可以使用 `astype()` 方法来转换 `X[start:end]` 的数据类型,例如:
```python
xx = torch.tensor(X[start:end].astype(np.float32), dtype=torch.float, requires_grad=True)
```
这将将 `X[start:end]` 转换为 `float32` 类型,并且可以成功创建 `xx` 张量。请确保您已经导入了 NumPy 和 PyTorch 库。
相关问题
xx = torch.tensor(X[start:end], dtype=torch.float, requires_grad=True)
这行代码是使用 PyTorch 创建一个张量(tensor),其中 `X` 是一个输入数据的张量,`start` 和 `end` 是索引范围。这个张量 `xx` 的数据类型被指定为 `torch.float`,并且设置了 `requires_grad` 为 True,表示我们希望对这个张量进行梯度计算。梯度计算是用于反向传播算法的,可以用来优化模型的参数。
s_t = torch.tensor(s_t, dtype=torch.float) TypeError: only size-1 arrays can be converted to Python scalars
这个错误是由于尝试将一个大小不为1的数组转换为标量值所引起的。在PyTorch中,`torch.tensor`函数只接受大小为1的数组作为输入。如果你尝试转换一个大小大于1的数组,就会引发这个错误。
要解决这个问题,你需要确保将大小为1的数组传递给`torch.tensor`函数。你可以使用`numpy`库的`reshape`函数将数组重新调整为大小为1的形状,然后再将其传递给`torch.tensor`函数。
下面是一个示例代码,演示了如何解决这个问题:
```python
import torch
import numpy as np
s_t = np.array([1, 2, 3]) # 假设这是你的数组
s_t = np.reshape(s_t, (1, -1)) # 将数组重新调整为大小为1的形状
s_t = torch.tensor(s_t, dtype=torch.float) # 将调整后的数组转换为张量
```
请注意,`np.reshape`函数中的参数`(1, -1)`表示将数组调整为大小为1的形状,其中`-1`表示自动计算该维度的大小。你可以根据实际情况调整这些参数。
相关推荐
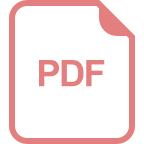
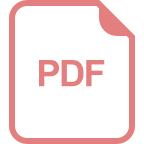
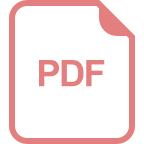













