autodl连接jupyternotebook
时间: 2023-10-27 20:06:53 浏览: 616
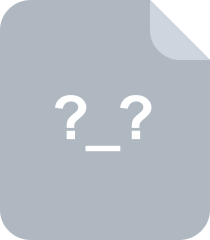
【从kitti开始自动驾驶】--10.2 测距离jupyter-notebook实现
要在Jupyter Notebook中使用AutoDL,您需要按照以下步骤进行设置:
1. 安装AutoDL的Python包:`pip install autodl`
2. 在Jupyter Notebook中导入AutoDL:`import autodl`
3. 连接到AutoDL服务器:`autodl.connect()`
4. 输入您的AutoDL凭据(用户名和密码)
5. 成功连接后,您可以在Jupyter Notebook中使用AutoDL的功能了。
阅读全文
相关推荐
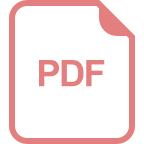
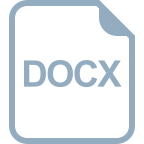















